A Hierarchical Bayes Approach to Modeling Heterogeneity in Discrete Choice Experiments: An Application to Public Preferences for Prenatal Screening
The Patient - Patient-Centered Outcomes Research(2019)
摘要
Background Previous studies assessing preferences for prenatal screening have focused on preferences of the affected population and have largely assumed homogeneous preferences. We aimed to estimate public preferences and willingness to pay for prenatal screening and diagnosis from a Canadian general population sample, and to model preferences at the individual level. Methods A discrete choice experiment was used to elicit preferences for different aspects of prenatal screening and diagnostic strategies. Strategies differed in five attributes: timing of the results, false-negative rate, false-positive rate, risk of miscarriage, and out-of-pocket cost. Respondents made forced and unforced choices using a dual response approach. Hierarchical Bayes analysis was applied to estimate individual-level part-worth utilities. Individual probability and expected uptake of prenatal screening under different scenarios were also assessed. Subgroup analyses were conducted using individual-level preferences. Results The final analyses were based on a sample of 4601 respondents. Results showed that the two most important attributes were false-negative rate and miscarriage risk. There was significant heterogeneity in preferences among respondents. Individuals’ perception of the risk of pregnancy with chromosomal abnormalities affected their preferences for screening. The relatively high uptake of safe and accurate screening among all groups of respondents indicated people’s desire for information about the health of their unborn baby regardless of their decision to continue the pregnancy. Conclusion Our findings are consistent with previous studies based on affected-population preferences. This concordance should be reassuring from a policy perspective and can inform the design of publicly funded prenatal screening programs.
更多查看译文
AI 理解论文
溯源树
样例
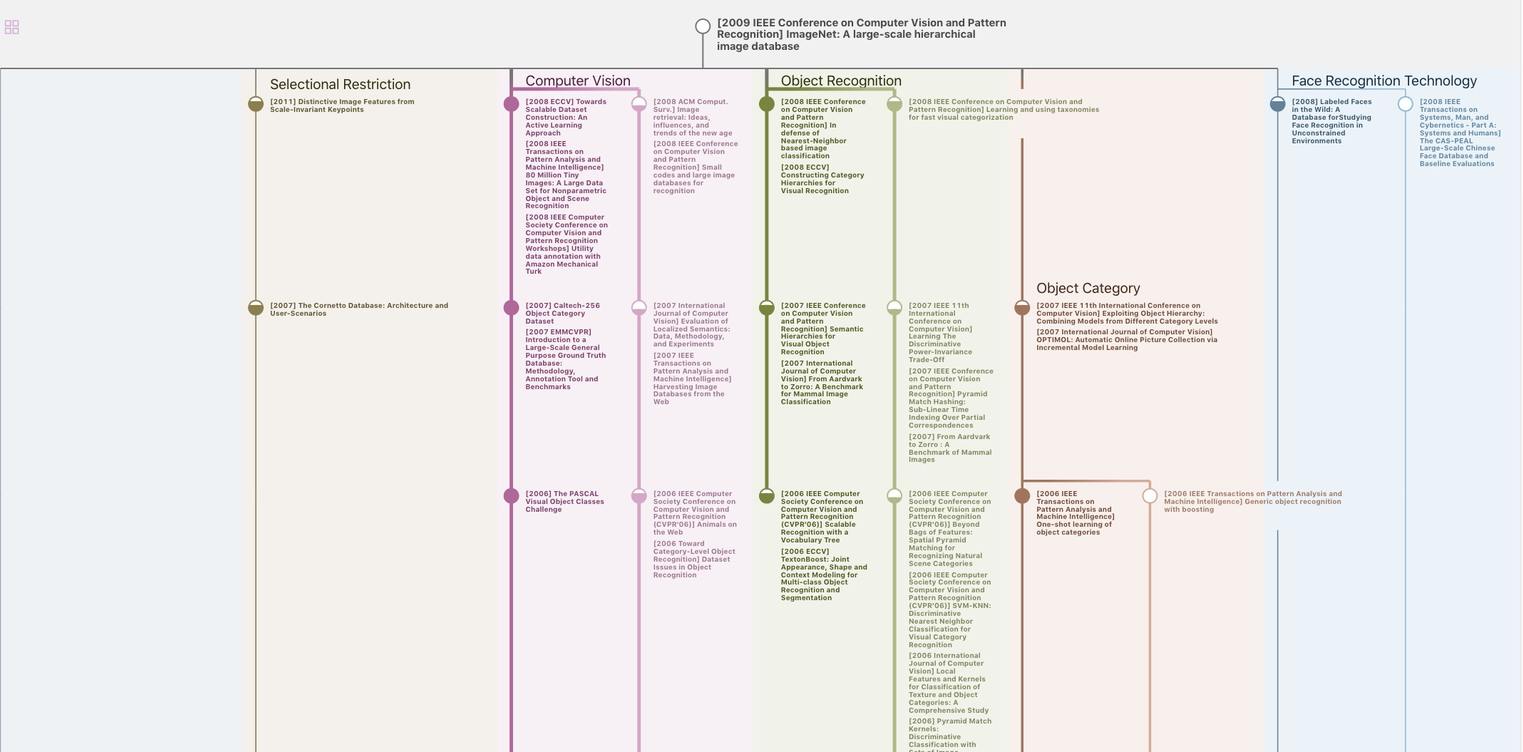
生成溯源树,研究论文发展脉络
Chat Paper
正在生成论文摘要