A Hybrid Deep Learning Approach With Gcn And Lstm For Traffic Flow Prediction
2019 IEEE INTELLIGENT TRANSPORTATION SYSTEMS CONFERENCE (ITSC)(2019)
摘要
Traffic flow prediction is an important functional component of Intelligent Transportation Systems (ITS). In this paper, we propose a hybrid deep learning approach, called graph and attention-based long short-term memory network (GLA), to efficiently capture the spatial-temporal features in Traffic flow. Firstly, we apply graph convolutional network (GCN) to mine the spatial relationships of Traffic flow over multiple observation stations, in which the adjacent matrix is determined by a data-driven approach. Then, we feed the output of the GCN model to the long short-term memory (LSTM) model which extracts temporal features embedded in Traffic flow. Further, we implement a soft attention mechanism on the extracted spatial-temporal Traffic features to make final prediction. We test the proposed method over the PeMS data sets. Experimental results show that the proposed model performs better than the competing methods.
更多查看译文
关键词
hybrid deep learning approach,LSTM,traffic flow prediction,functional component,intelligent transportation systems,short-term memory network,spatial-temporal features,graph convolutional network,data-driven approach,GCN model,short-term memory model,spatial-temporal traffic features
AI 理解论文
溯源树
样例
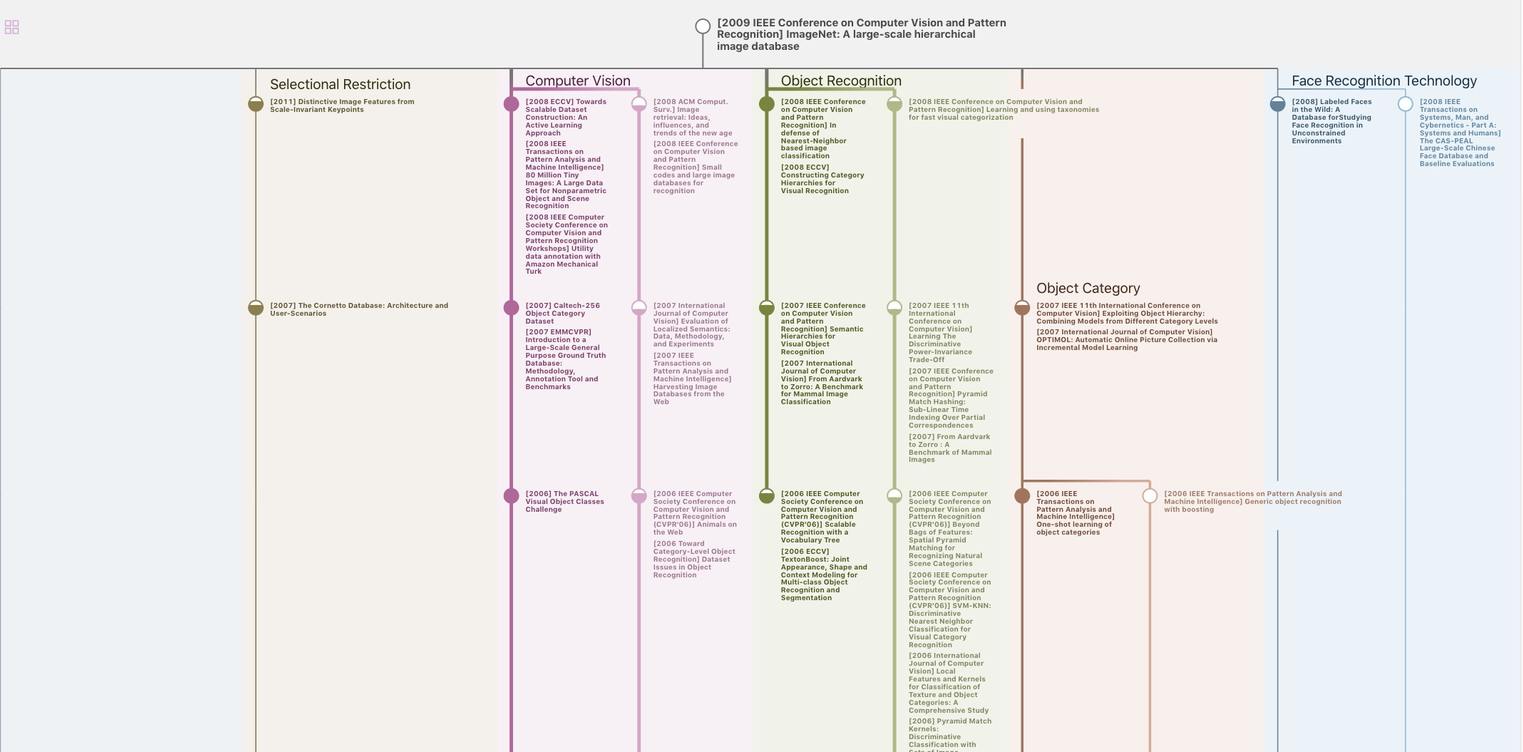
生成溯源树,研究论文发展脉络
Chat Paper
正在生成论文摘要