A Deep Reinforcement Learning Approach To High-Speed Train Timetable Rescheduling Under Disturbances
2019 IEEE INTELLIGENT TRANSPORTATION SYSTEMS CONFERENCE (ITSC)(2019)
摘要
Train timetable rescheduling (TTR) aims to address the recovery of train operation order in reordering and retiming strategies during disturbances. Considering this problem, this paper introduces a deep reinforcement learning (DRL) approach to minimize the average total delay for all trains along the railway line. Specifically, the detailed train operation in block sections and stations is illustrated to establish a learning environment involving its state sets, action sets, and the reward function. The learning agent is responsible for adjusting running times, dwell times and departure sequences for trains and conflicts are resolved simultaneously. Numerical experiments are performed on an adapted timetable carried out on the Beijing-Shanghai high-speed railway line. The experimental results indicate that the proposed approach reduces the average total delay by 46.38% in real time, compared to the First-Come-First-Served (FCFS) method.
更多查看译文
关键词
Train timetable rescheduling, Deep reinforcement learning, Conflict resolution
AI 理解论文
溯源树
样例
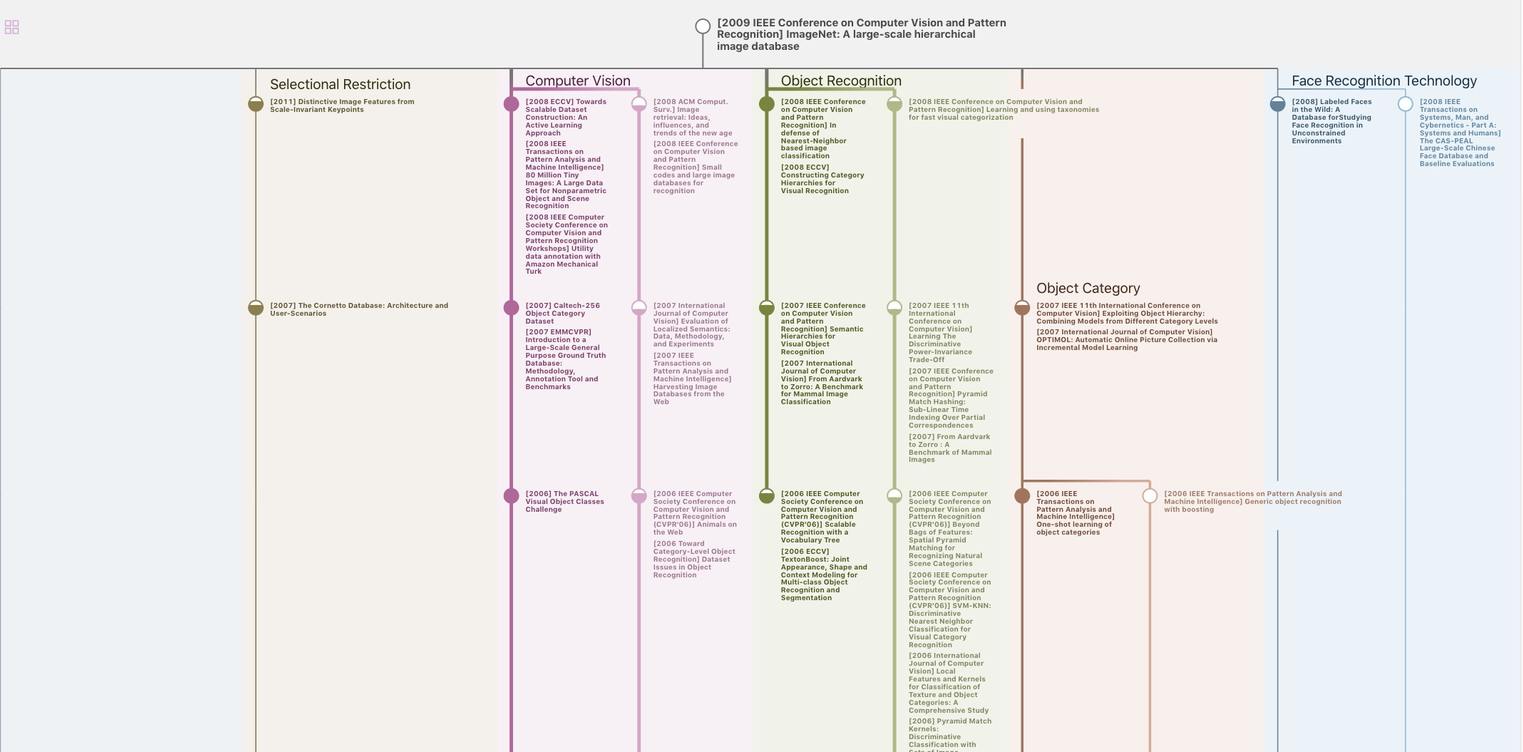
生成溯源树,研究论文发展脉络
Chat Paper
正在生成论文摘要