Traffic Assignment Based On Parsimonious Data: The Ideal Flow Network
2019 IEEE INTELLIGENT TRANSPORTATION SYSTEMS CONFERENCE (ITSC)(2019)
摘要
Traffic assignment models are used to estimate and distribute flows in a road network so that congestion and travel time delay are minimized. However, most of these models require the costly origin-destination (OD) data. In this paper, through the ideal flow network (IFN) and the maximum entropy principle, traffic flows can be estimated even with limited road network data. The macro level OD matrix is mathematically transformed into micro level OD on each intersection, which yields richer transportation structure data such as capacity and lane width. When data available is limited to only a few links or intersections, the remaining missing data can be set by computing for the stochastic matrix from the capacity ratio. Even if the capacity ratio is not available, the connectivity of the road network structure can be used to derive the stochastic matrix. With this characteristic of the IFN, even with limited and parsimonious data that can be collected from any link or intersection using a video camera, GPS, or any ITS sensing device, link flows of an entire network can be updated dynamically. Link flow results using IFN are almost the same as actual results, which is illustrated using the Sioux Falls transportation network.
更多查看译文
关键词
IFN,Sioux Falls transportation network,parsimonious data,ideal flow network,traffic assignment models,travel time delay,origin-destination data,maximum entropy principle,traffic flows,road network data,richer transportation structure data,lane width,stochastic matrix,road network structure,link flows,ITS sensing device,video camera,GPS
AI 理解论文
溯源树
样例
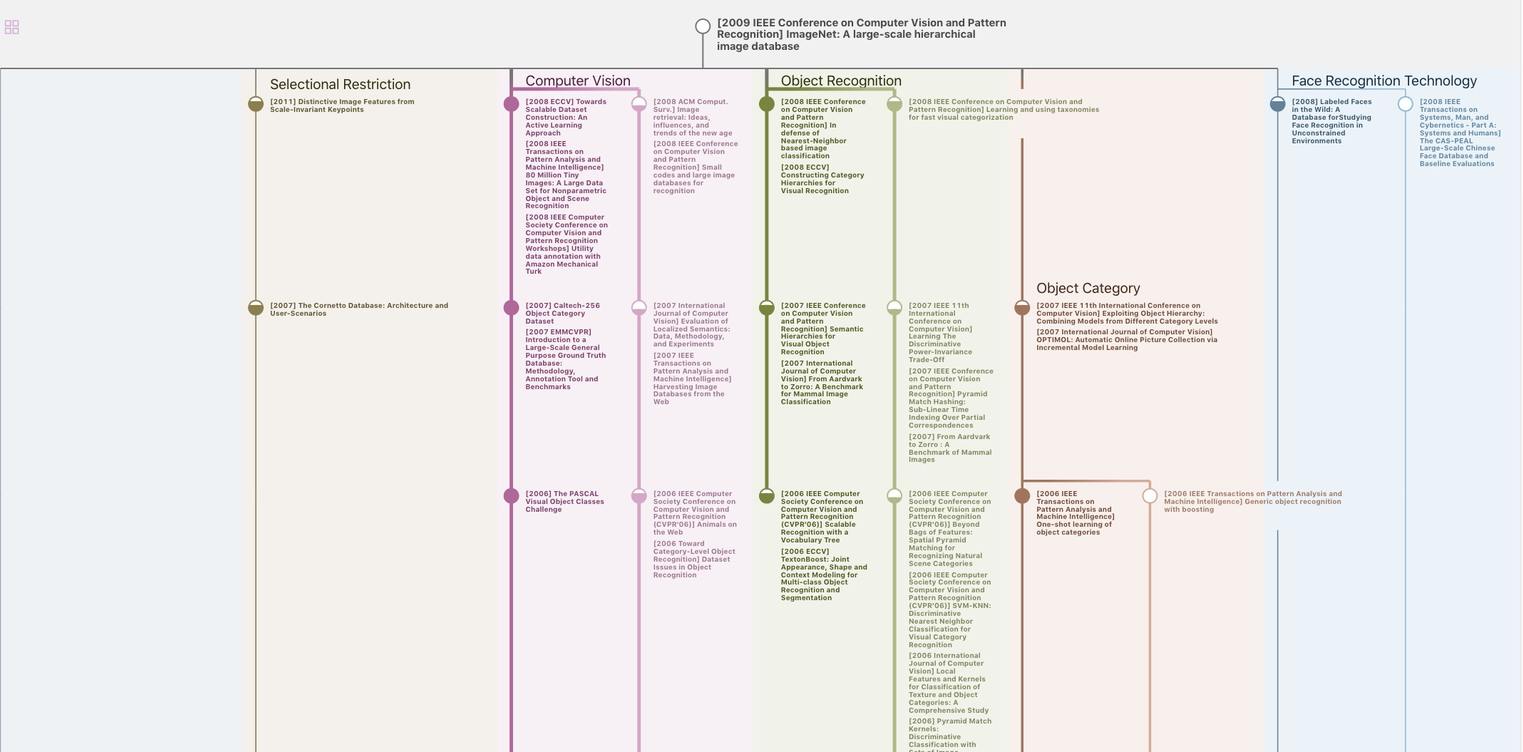
生成溯源树,研究论文发展脉络
Chat Paper
正在生成论文摘要