Learning the Correct Robot Trajectory in Real-Time from Physical Human Interactions
ACM Transactions on Human-Robot Interaction (THRI)(2020)
摘要
We present a learning and control strategy that enables robots to harness physical human interventions to update their trajectory and goal during autonomous tasks. Within the state of the art, the robot typically reacts to physical interactions by modifying a local segment of its trajectory, or by searching for the global trajectory offline, using either replanning or previous demonstrations. Instead, we explore a one-shot approach: here, the robot updates its entire trajectory and goal in real time without relying on multiple iterations, offline demonstrations, or replanning. Our solution is grounded in optimal control and gradient descent, and extends linear-quadratic regulator controllers to generalize across methods that locally or globally modify the robot’s underlying trajectory. In the best case, this Linear-quadratic regulator + Learning approach matches the optimal offline response to physical interactions, and—in more challenging cases—our strategy is robust to noisy and unexpected human corrections. We compare the proposed solution against other real-time strategies in a user study and demonstrate its efficacy in terms of both objective and subjective measures.
更多查看译文
关键词
Learning from demonstrations, optimal control, physical human-robot interaction
AI 理解论文
溯源树
样例
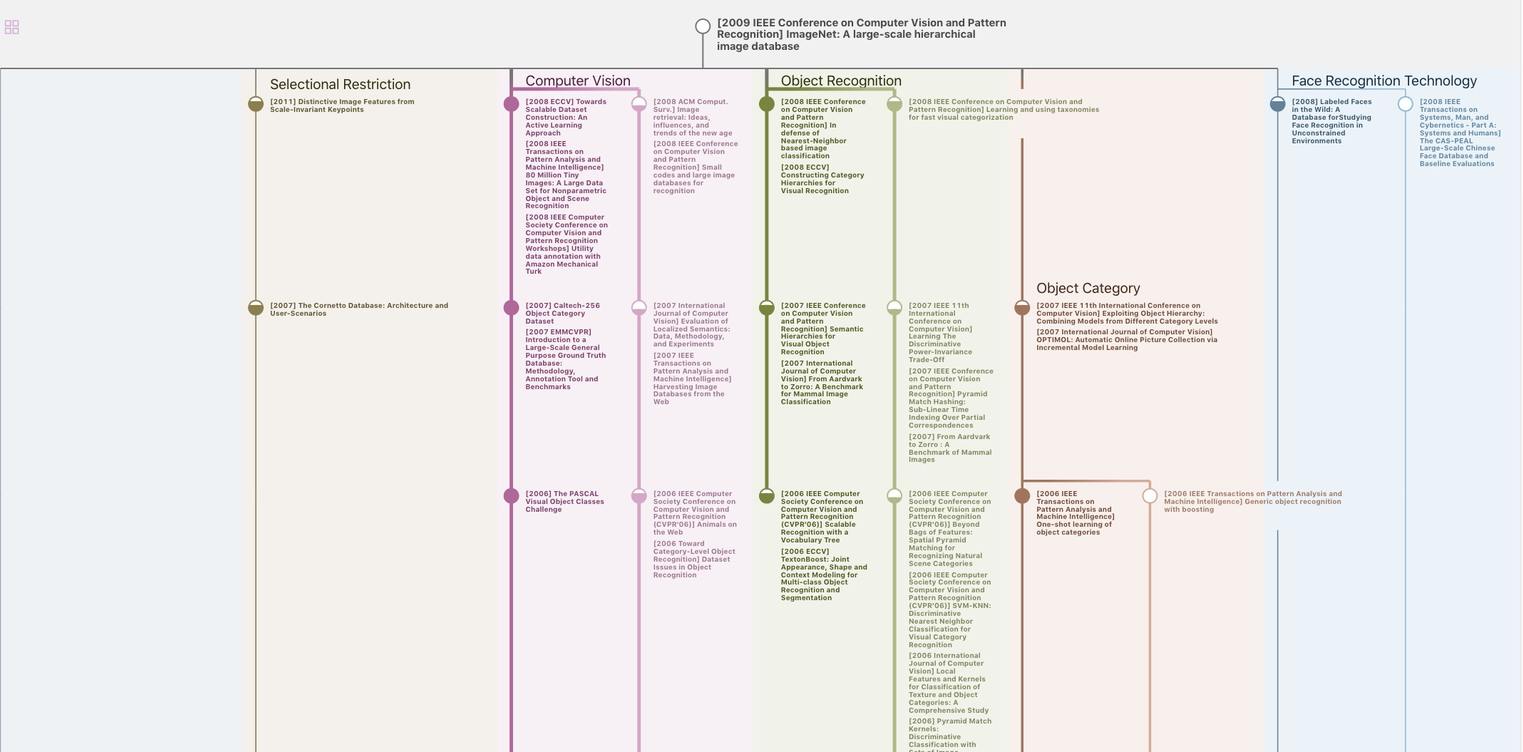
生成溯源树,研究论文发展脉络
Chat Paper
正在生成论文摘要