Data-driven design of metal–organic frameworks for wet flue gas CO 2 capture
NATURE(2019)
摘要
Limiting the increase of CO 2 in the atmosphere is one of the largest challenges of our generation 1 . Because carbon capture and storage is one of the few viable technologies that can mitigate current CO 2 emissions 2 , much effort is focused on developing solid adsorbents that can efficiently capture CO 2 from flue gases emitted from anthropogenic sources 3 . One class of materials that has attracted considerable interest in this context is metal–organic frameworks (MOFs), in which the careful combination of organic ligands with metal-ion nodes can, in principle, give rise to innumerable structurally and chemically distinct nanoporous MOFs. However, many MOFs that are optimized for the separation of CO 2 from nitrogen 4 – 7 do not perform well when using realistic flue gas that contains water, because water competes with CO 2 for the same adsorption sites and thereby causes the materials to lose their selectivity. Although flue gases can be dried, this renders the capture process prohibitively expensive 8 , 9 . Here we show that data mining of a computational screening library of over 300,000 MOFs can identify different classes of strong CO 2 -binding sites—which we term ‘adsorbaphores’—that endow MOFs with CO 2 /N 2 selectivity that persists in wet flue gases. We subsequently synthesized two water-stable MOFs containing the most hydrophobic adsorbaphore, and found that their carbon-capture performance is not affected by water and outperforms that of some commercial materials. Testing the performance of these MOFs in an industrial setting and consideration of the full capture process—including the targeted CO 2 sink, such as geological storage or serving as a carbon source for the chemical industry—will be necessary to identify the optimal separation material.
更多查看译文
关键词
Atomistic models,Cheminformatics,Metal–organic frameworks,Science,Humanities and Social Sciences,multidisciplinary
AI 理解论文
溯源树
样例
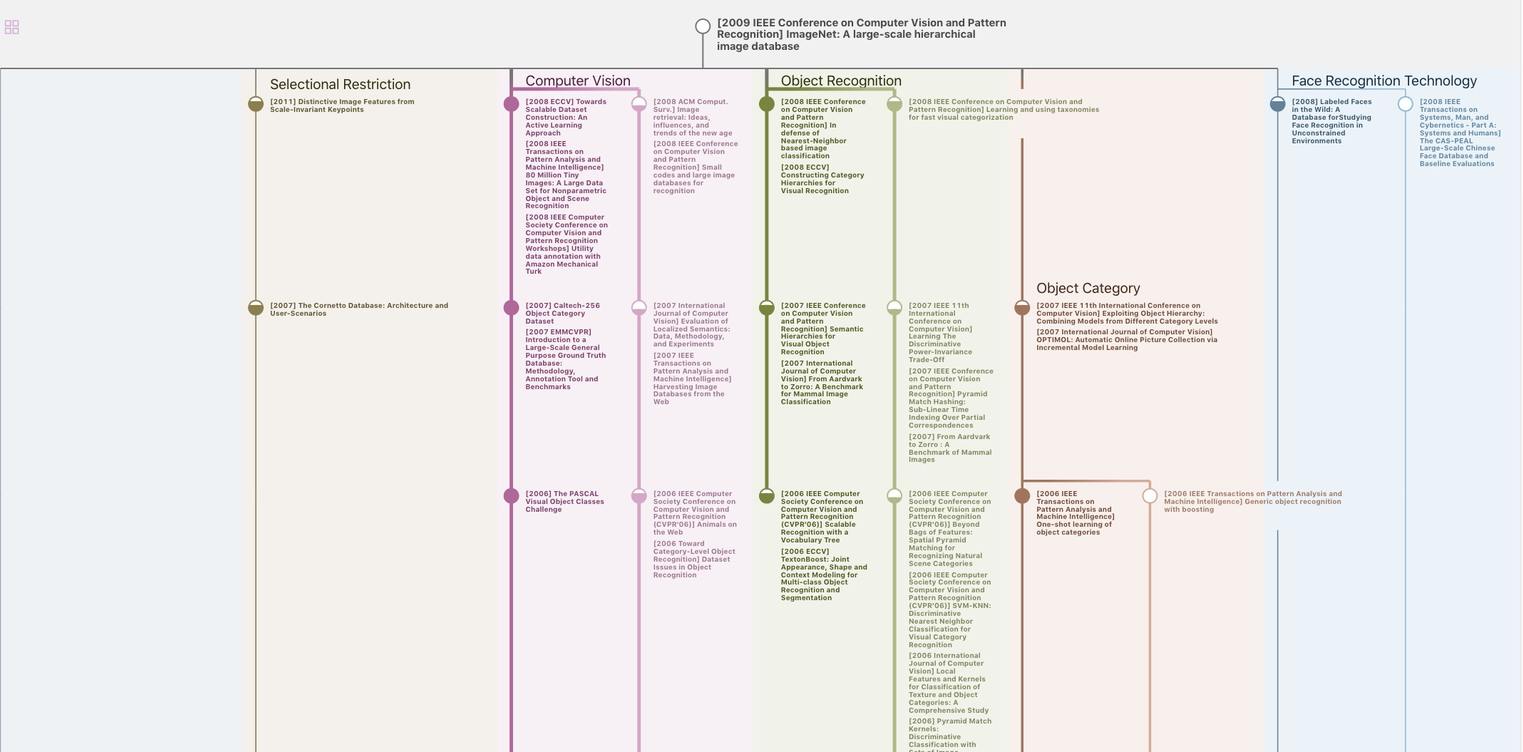
生成溯源树,研究论文发展脉络
Chat Paper
正在生成论文摘要