Sublinear Optimal Policy Value Estimation in Contextual Bandits
INTERNATIONAL CONFERENCE ON ARTIFICIAL INTELLIGENCE AND STATISTICS, VOL 108(2019)
摘要
We study the problem of estimating the expected reward of the optimal policy in the stochastic disjoint linear bandit setting. We prove that for certain settings it is possible to obtain an accurate estimate of the optimal policy value even with a number of samples that is sublinear in the number that would be required to \emph{find} a policy that realizes a value close to this optima. We establish nearly matching information theoretic lower bounds, showing that our algorithm achieves near optimal estimation error. Finally, we demonstrate the effectiveness of our algorithm on joke recommendation and cancer inhibition dosage selection problems using real datasets.
更多查看译文
关键词
contextual bandits,optimal policy value estimation
AI 理解论文
溯源树
样例
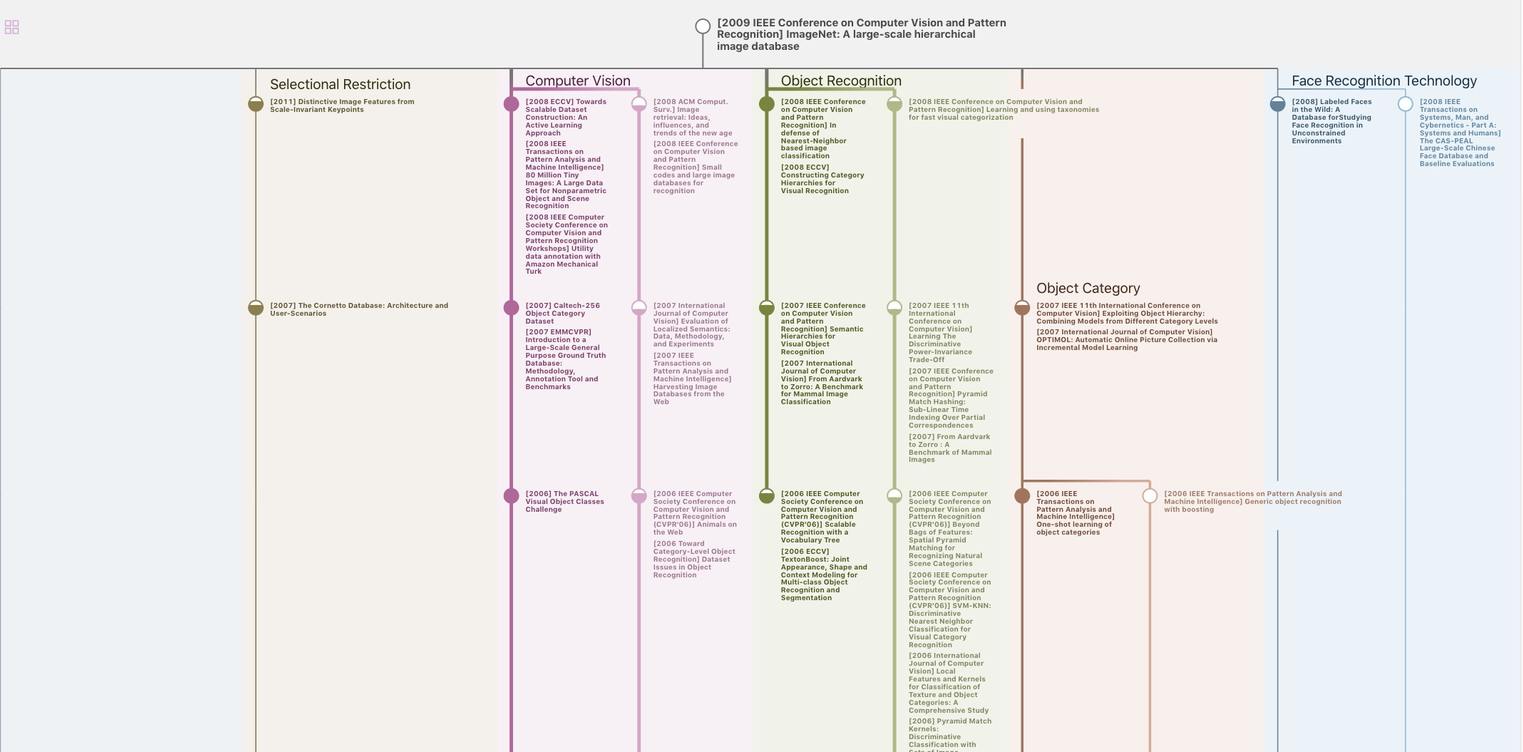
生成溯源树,研究论文发展脉络
Chat Paper
正在生成论文摘要