Cubic Regularized ADMM with Convergence to a Local Minimum in Non-convex Optimization
2019 57th Annual Allerton Conference on Communication, Control, and Computing (Allerton)(2019)
摘要
How to escape saddle points is a critical issue in non-convex optimization. Previous methods on this issue mainly assume that the objective function is Hessian-Lipschitz, which leave a gap for applications using non-Hessian-Lipschitz functions. In this paper, we propose Cubic Regularized Alternating Direction Method of Multipliers (CR-ADMM) to escape saddle points of separable non-convex functions containing a non-Hessian-Lipschitz component. By carefully choosing a parameter, we prove that CR-ADMM converges to a local minimum of the original function with a rate of O(1/T
1/3
) in time horizon T, which is faster than gradient-based methods. We also show that when one or more steps of CR-ADMM are not solved exactly, CRADMM can converge to a neighborhood of the local minimum. Through the experiments of matrix factorization problems, CRADMM is shown to have a faster rate and a lower optimality gap compared with other gradient-based methods. Our approach can also find applications in other scenarios where regularized non-convex cost minimization is performed, such as parameter optimization of deep neural networks.
更多查看译文
关键词
gradient-based methods,CR-ADMM,local minimum,lower optimality gap,nonconvex cost minimization,parameter optimization,nonconvex optimization,saddle points,objective function,Hessian-Lipschitz,nonHessian-Lipschitz functions,nonconvex functions,nonHessian-Lipschitz component,cubic regularized alternating direction method of multipliers,cubic regularized ADMM
AI 理解论文
溯源树
样例
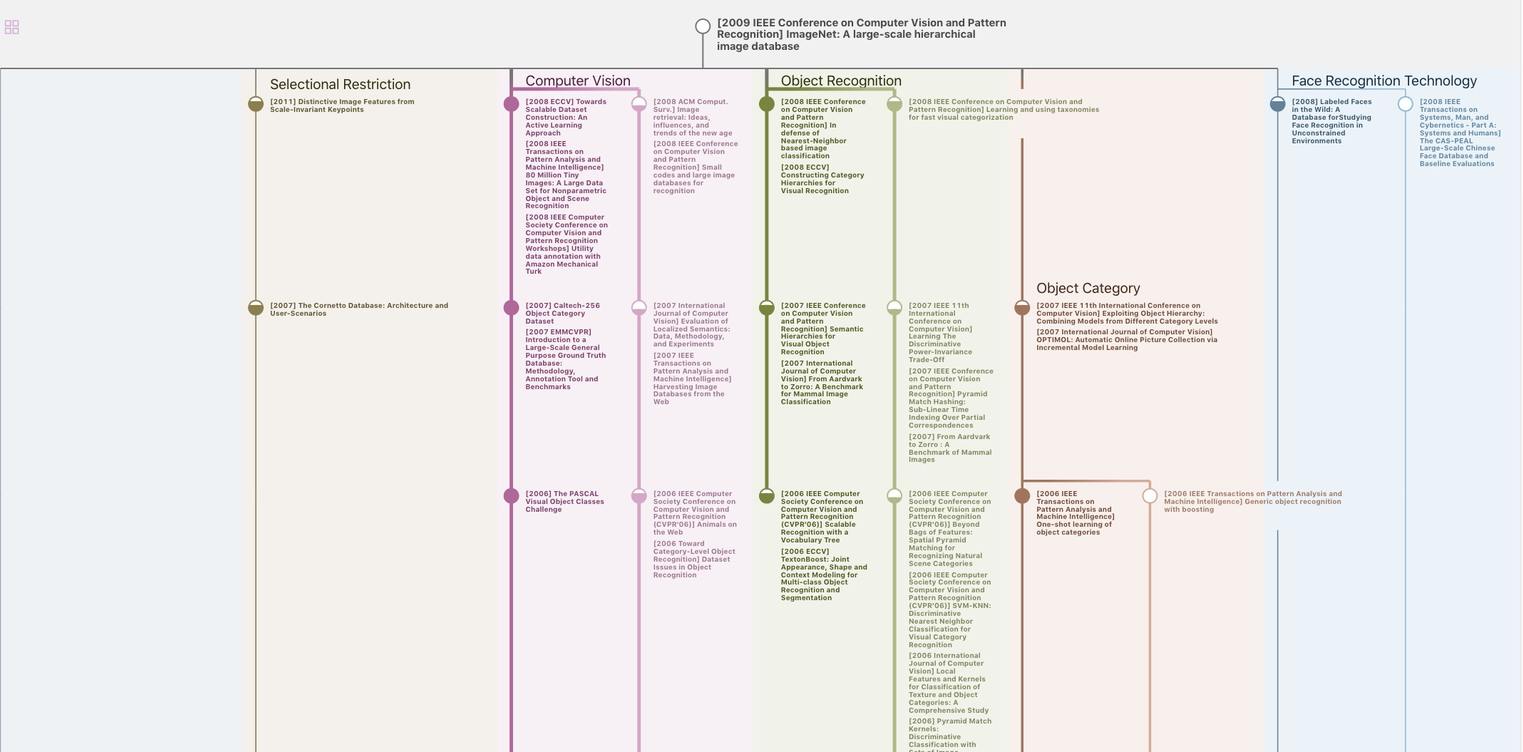
生成溯源树,研究论文发展脉络
Chat Paper
正在生成论文摘要