Towards an Embedded and Real-Time Joint Human-Machine Monitoring Framework: Dataset optimization Techniques for Anomaly Detection
2019 IFIP/IEEE 27th International Conference on Very Large Scale Integration (VLSI-SoC)(2019)
摘要
Unmanned Remotely Operated Vehicles (ROVs) are widely used across many civil application domains including real-time monitoring, security and surveillance, and search and rescue missions. Most of these applications require the human operator to control the ROV under stressful conditions and harsh environments. As such, the remote-control operator is prone to sometimes issuing anomalous commands, because of either unwanted hand or finger motion or even irrational decisions, results of fatigue, stress, etc. To enable detection of such anomalies, we propose the use of a joint human-ROV monitoring framework, by monitoring the human operator's bio-signals and the ROV's sensory data. The framework is anticipated to run on the ROV, enabling it to recognize and possibly ignore anomalies, potentially paving the way for a shared control algorithm. In this paper therefore, we present a first step towards achieving this goal, focusing on optimizing the fused dataset consisting of the aforementioned signals and investigated different techniques such as feature extraction and statistical component analysis, in an effort to reduce the dimensionality of the dataset. To this end, we present a dataset constructed by surface Electromyography (sEMG) signals from various operators, fused with the ROV's inertial sensors. Through our proposed optimizations, we are able to reduce both the data size as well as the necessary features and signal components, while maintaining the ability to detect anomalies with at least 85% accuracy depending on the dimensionality reduction technique (over raw data). We evaluated our dataset over a variety of classifier configurations and embedded platforms with noteworthy energy and performance benefits.
更多查看译文
关键词
Remotely Operated Vehicles (ROVs),Anomaly Detection,Embedded and Real Time Systems
AI 理解论文
溯源树
样例
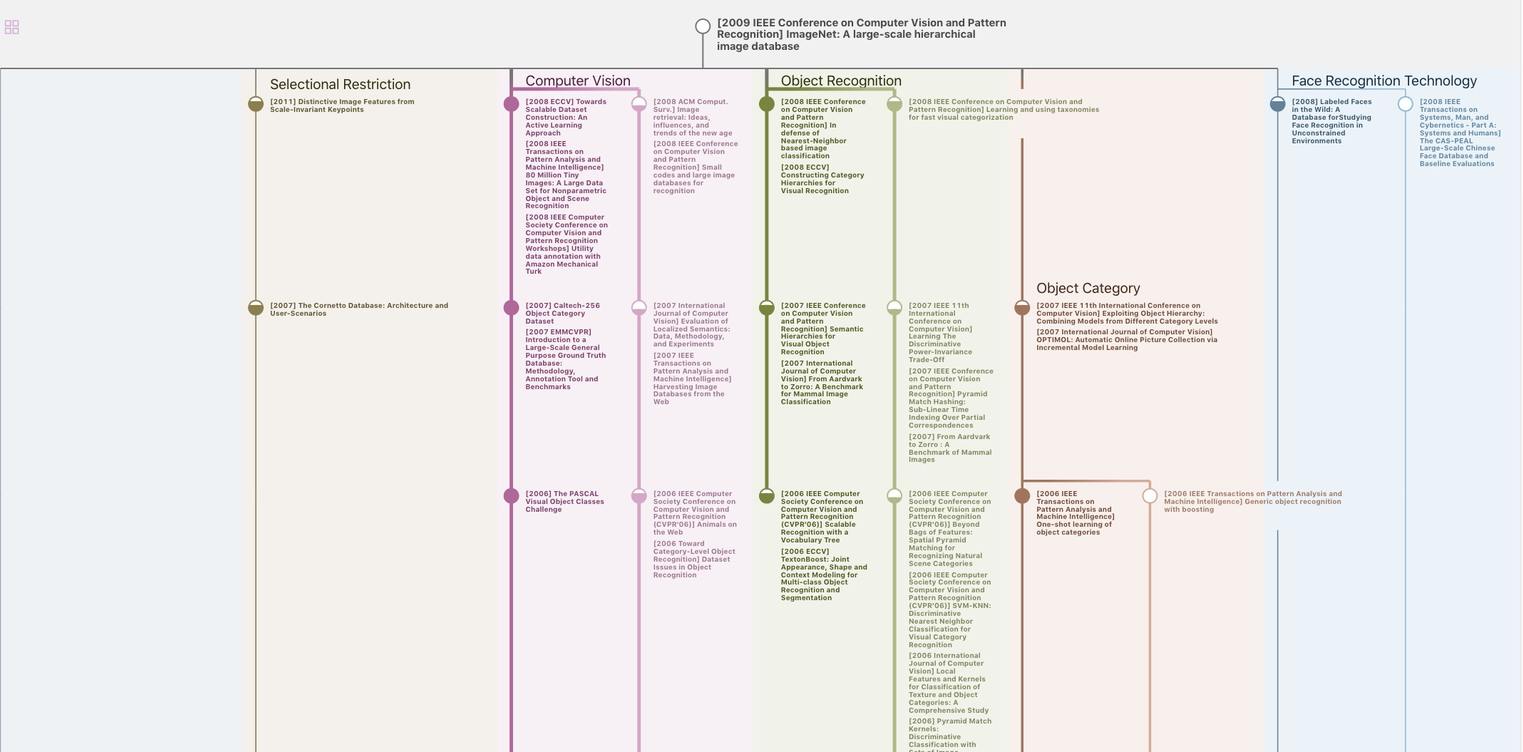
生成溯源树,研究论文发展脉络
Chat Paper
正在生成论文摘要