Latent-Space Laplacian Pyramids for Adversarial Representation Learning with 3D Point Clouds
VISAPP: PROCEEDINGS OF THE 15TH INTERNATIONAL JOINT CONFERENCE ON COMPUTER VISION, IMAGING AND COMPUTER GRAPHICS THEORY AND APPLICATIONS, VOL 4: VISAPP(2019)
摘要
Constructing high-quality generative models for 3D shapes is a fundamental task in computer vision with diverse applications in geometry processing, engineering, and design. Despite the recent progress in deep generative modelling, synthesis of finely detailed 3D surfaces, such as high-resolution point clouds, from scratch has not been achieved with existing approaches. In this work, we propose to employ the latent-space Laplacian pyramid representation within a hierarchical generative model for 3D point clouds. We combine the recently proposed latent-space GAN and Laplacian GAN architectures to form a multi-scale model capable of generating 3D point clouds at increasing levels of detail. Our evaluation demonstrates that our model outperforms the existing generative models for 3D point clouds.
更多查看译文
关键词
Deep Learning, 3D Point Clouds, Generative Adversarial Networks, Multi-scale 3D Modelling
AI 理解论文
溯源树
样例
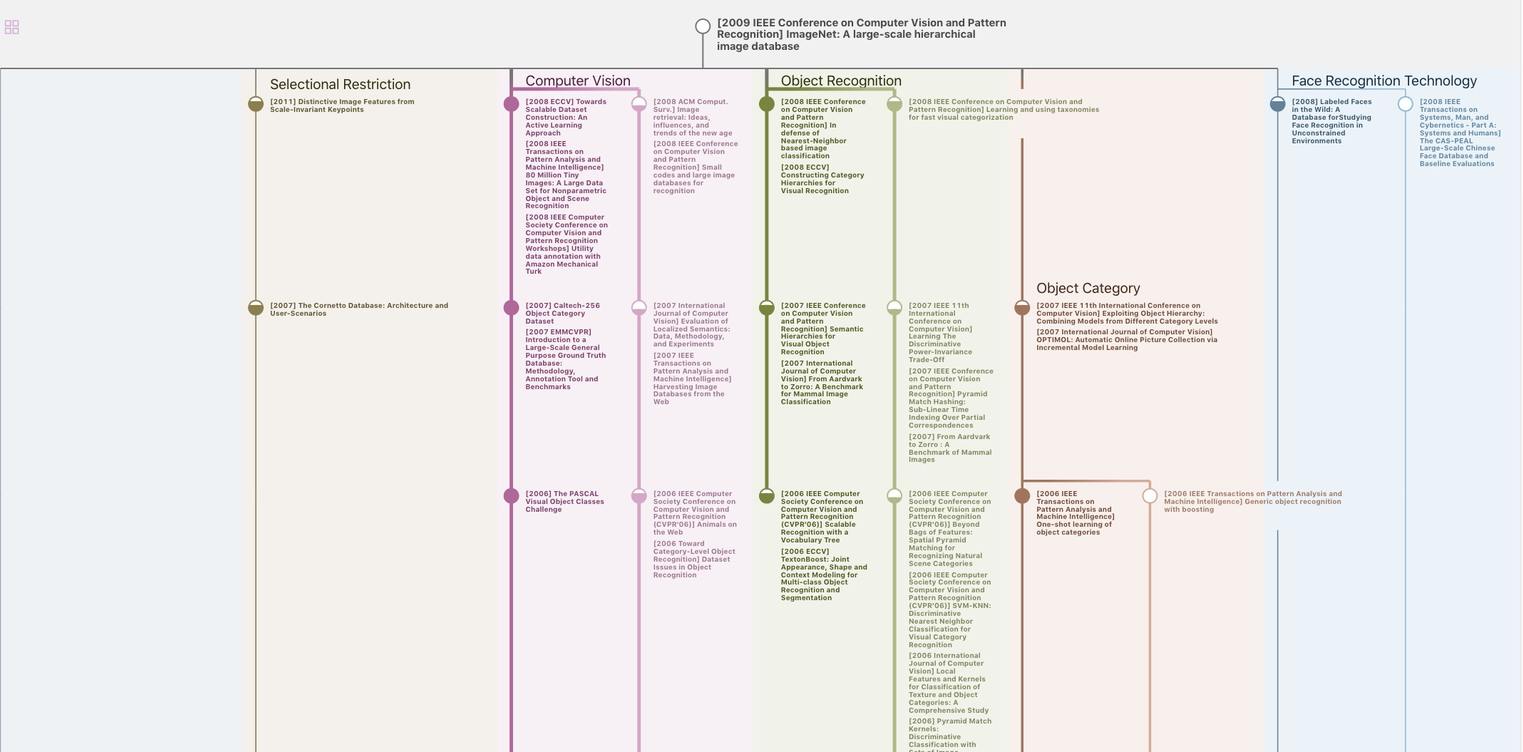
生成溯源树,研究论文发展脉络
Chat Paper
正在生成论文摘要