Lessons from reinforcement learning for biological representations of space
Vision Research(2020)
摘要
Neuroscientists postulate 3D representations in the brain in a variety of different coordinate frames (e.g. ‘head-centred’, ‘hand-centred’ and ‘world-based’). Recent advances in reinforcement learning demonstrate a quite different approach that may provide a more promising model for biological representations underlying spatial perception and navigation. In this paper, we focus on reinforcement learning methods that reward an agent for arriving at a target image without any attempt to build up a 3D ‘map’. We test the ability of this type of representation to support geometrically consistent spatial tasks such as interpolating between learned locations using decoding of feature vectors. We introduce a hand-crafted representation that has, by design, a high degree of geometric consistency and demonstrate that, in this case, information about the persistence of features as the camera translates (e.g. distant features persist) can improve performance on the geometric tasks. These examples avoid Cartesian (in this case, 2D) representations of space. Non-Cartesian, learned representations provide an important stimulus in neuroscience to the search for alternatives to a ‘cognitive map’.
更多查看译文
关键词
Deep Reinforcement Learning,3D spatial representation,Moving observer,Navigation,View-based,Parallax
AI 理解论文
溯源树
样例
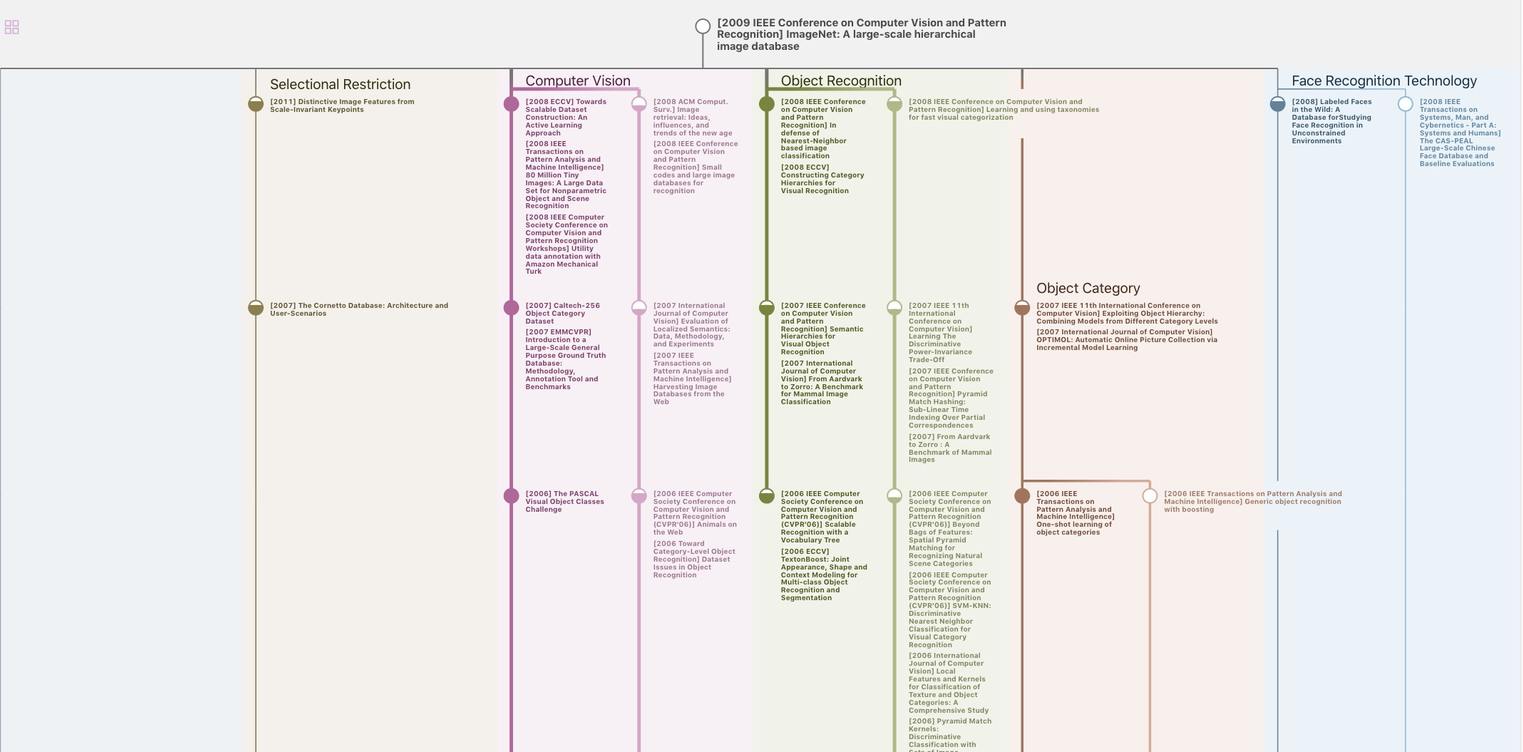
生成溯源树,研究论文发展脉络
Chat Paper
正在生成论文摘要