Automatic Recognition of Multiple Affective States in Virtual Rehabilitation by Exploiting the Dependency Relationships
2019 8th International Conference on Affective Computing and Intelligent Interaction (ACII)(2019)
摘要
The automatic recognition of multiple affective states can be enhanced if the underpinning computational models explicitly consider the interactions between the states. This work proposes a computational model that incorporates the dependencies between four states (tiredness, anxiety, pain, and engagement)known to appear in virtual rehabilitation sessions of post-stroke patients, to improve the automatic recognition of the patients' states. A dataset of five stroke patients which includes their fingers' pressure (PRE), hand movements (MOV)and facial expressions (FAE)during ten sessions of virtual rehabilitation was used. Our computational proposal uses the Semi-Naive Bayesian classifier (SNBC)as base classifier in a multiresolution approach to create a multimodal model with the three sensors (PRE, MOV, and FAE)with late fusion using SNBC (FSNB classifier). There is a FSNB classifier for each state, and they are linked in a circular classifier chain (CCC)to exploit the dependency relationships between the states. Results of CCC are over 90% of ROC AUC for the four states. Relationships of mutual exclusion between engagement and all the other states and some co-occurrences between pain and anxiety for the five patients were detected. Virtual rehabilitation platforms that incorporate the automatic recognition of multiple patient's states could leverage intelligent and empathic interactions to promote adherence to rehabilitation exercises.
更多查看译文
关键词
automatic affective states recognition,virtual rehabilitation,multi-label classification,classifier chains,stroke
AI 理解论文
溯源树
样例
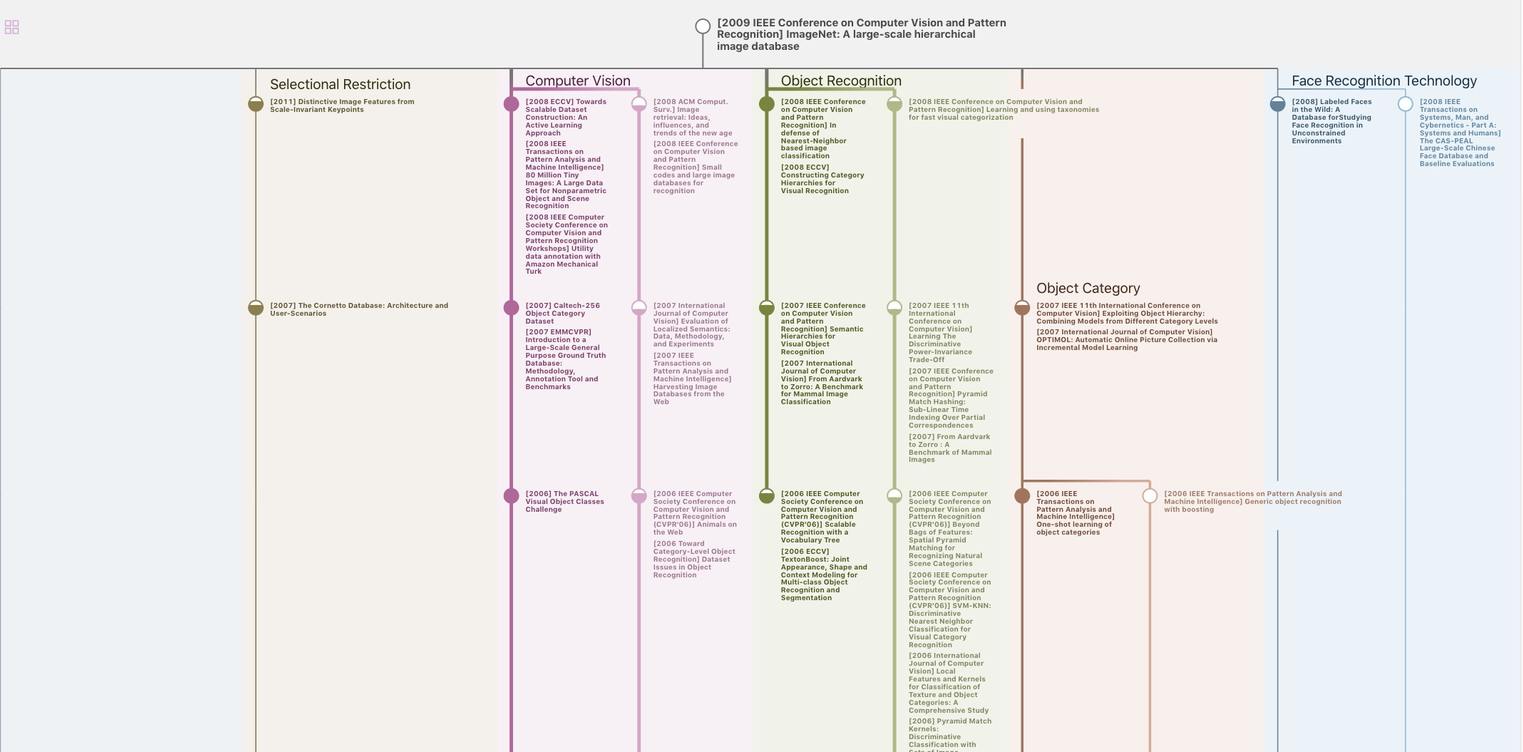
生成溯源树,研究论文发展脉络
Chat Paper
正在生成论文摘要