Video Retrieval with Similarity-Preserving Deep Temporal Hashing
ACM Transactions on Multimedia Computing, Communications, and Applications (TOMM)(2020)
摘要
Despite the fact that remarkable progress has been made in recent years, Content-based Video Retrieval (CBVR) is still an appealing research topic due to increasing search demands in the Internet era of big data. This article aims to explore an efficient CBVR system by discriminately hashing videos into short binary codes. Existing video hashing methods usually encounter two weaknesses originating from the following sources: (1) Most works adopt the separated stages method or the frame-pooling based end-to-end architecture. However, the spatial-temporal properties of videos cannot be fully explored or kept well in the follow-up hashing step. (2) Discriminative learning based on pairwise or triplet constraints often suffers from slow convergence and poor local optimization, mainly because of the limited samples for each update. To alleviate these problems, we propose an end-to-end video retrieval framework called the Similarity-Preserving Deep Temporal Hashing (SPDTH) network. Specifically, we equip the model with the ability to capture spatial-temporal properties of videos and to generate binary codes by stacked Gated Recurrent Units (GRUs). It unifies video temporal modeling and learning to hash into one step to allow for maximum retention of information. We also introduce a deep metric learning objective called ℓ2All_loss for network training by preserving intra-class similarity and inter-class separability, and a quantization loss between the real-valued outputs and the binary codes is minimized. Extensive experiments on several challenging datasets demonstrate that SPDTH can consistently outperform state-of-the-art methods.
更多查看译文
关键词
Content-based video retrieval, convolutional neural network, recurrent neural network, video hashing
AI 理解论文
溯源树
样例
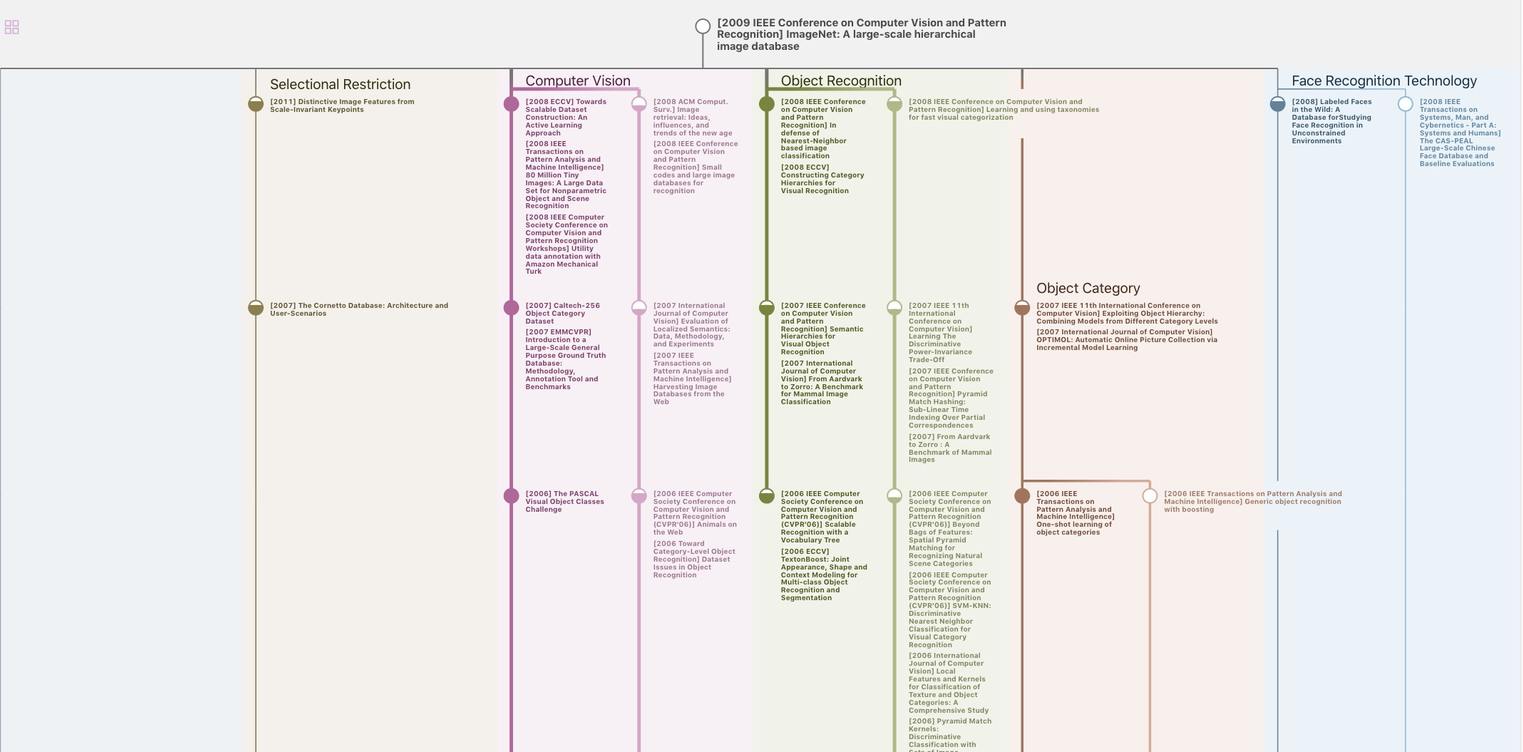
生成溯源树,研究论文发展脉络
Chat Paper
正在生成论文摘要