Wide and Deep Reinforcement Learning Extended for Grid-Based Action Games.
ICAART(2019)
摘要
For the last decade, Deep Reinforcement Learning (DRL) has undergone very rapid development. However, less has been done to integrate linear methods into it. Our research aims at a simple and practical Wide and Deep Reinforcement Learning framework to extend DRL algorithms by combining linear (wide) and non-linear (deep) methods. This framework can help to integrate expert knowledge or to fuse sensor information while at the same time improving the performance of existing DRL algorithms. To test this framework we have developed an extension of the popular Deep Q-Networks Algorithm, which we call Wide Deep Q-Networks. We analyze its performance compared to Deep Q-Networks and Linear Agents, as well as human agents by applying our new algorithm to Berkeley’s Pac-Man environment. Our algorithm considerably outperforms Deep Q-Networks both in terms of learning speed and ultimate performance, showing its potential for boosting existing algorithms. Furthermore, it is robust to the failure of one of its components.
更多查看译文
关键词
Wide and deep reinforcement learning, Wide deep Q-networks, Value function approximation, Reinforcement learning agents, Model fusion reinforcement learning
AI 理解论文
溯源树
样例
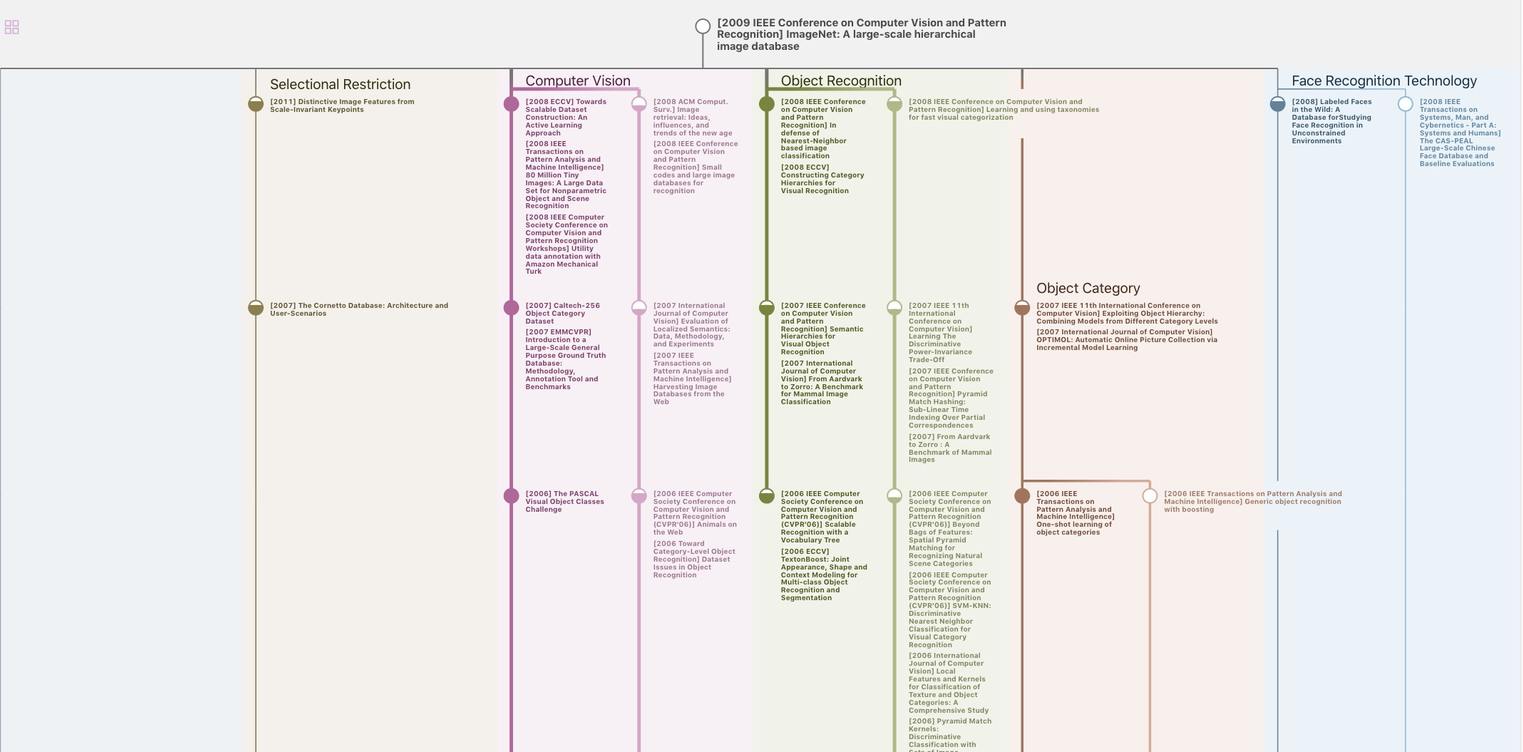
生成溯源树,研究论文发展脉络
Chat Paper
正在生成论文摘要