Non-Invasive Continuous Blood-Pressure Monitoring Models Based on Photoplethysmography and Electrocardiography.
SENSORS(2019)
摘要
Blood pressure is an extremely important blood hemodynamic parameter. The pulse wave contains abundant blood-pressure information, and the convenience and non-invasivity of its measurement make it ideal for non-invasive continuous monitoring of blood pressure. Based on combined photoplethysmography and electrocardiogram signals, this study aimed to extract the waveform information, introduce individual characteristics, and construct systolic and diastolic blood-pressure (SBP and DBP) estimation models using the back-propagation error (BP) neural network. During the model construction process, the mean impact value method was employed to investigate the impact of each feature on the model output and reduce feature redundancy. Moreover, the multiple population genetic algorithm was applied to optimize the BP neural network and determine the initial weights and threshold of the network. Finally, the models were integrated for further optimization to generate the final individualized continuous blood-pressure monitoring models. The results showed that the predicted values of the model in this study correlated significantly with the measured values of the electronic sphygmomanometer. The estimation errors of the model met the Association for the Advancement of Medical Instrumentation (AAMI) criteria (the SBP error was 2.5909 +/- 3.4148 mmHg, and the DBP error was 2.6890 +/- 3.3117 mmHg) and the Grade A British Hypertension Society criteria.
更多查看译文
关键词
characteristics of pulse waveform,error back-propagation neural network,model integration,multiple population genetic algorithm,noninvasive continuous blood pressure monitoring
AI 理解论文
溯源树
样例
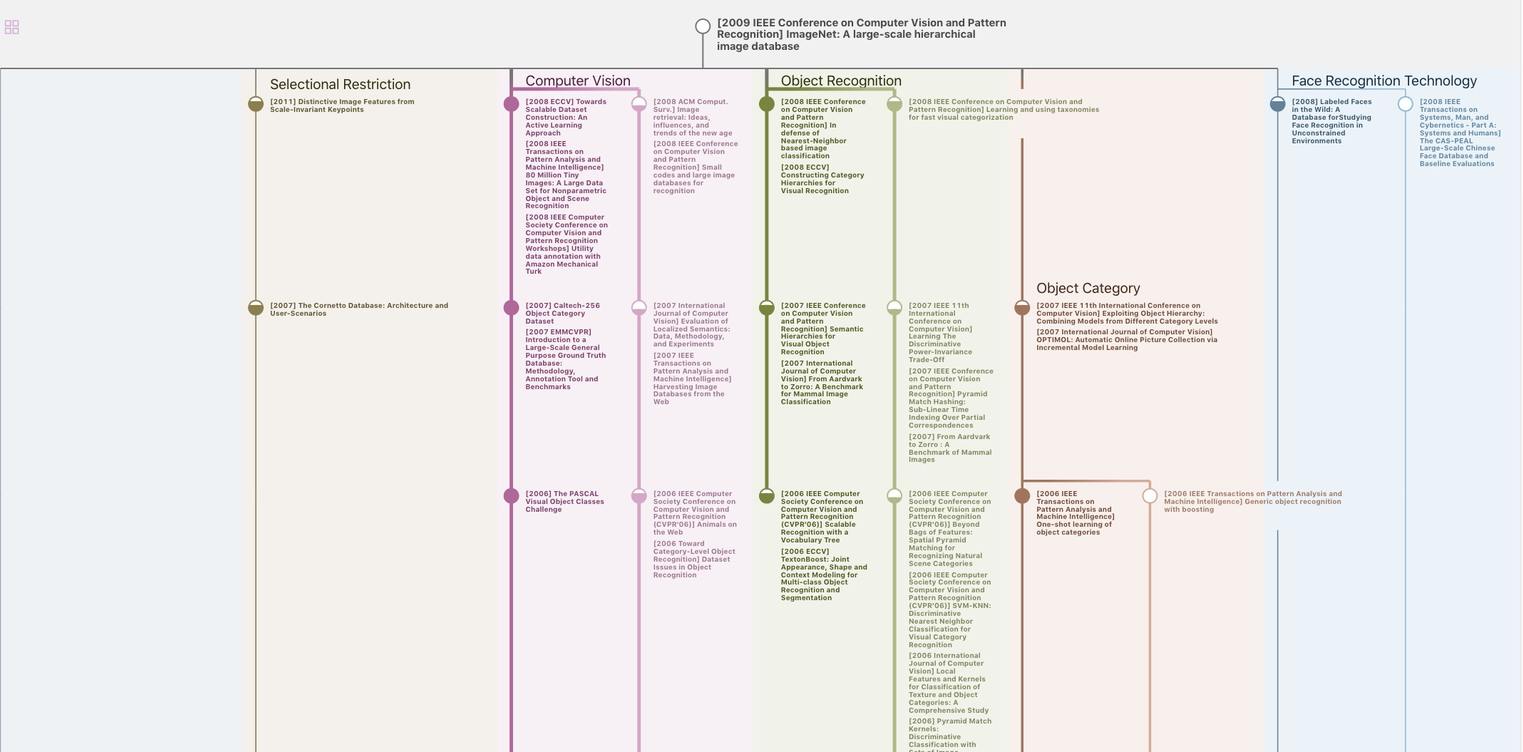
生成溯源树,研究论文发展脉络
Chat Paper
正在生成论文摘要