Towards Robust Learning With Different Label Noise Distributions
2020 25TH INTERNATIONAL CONFERENCE ON PATTERN RECOGNITION (ICPR)(2020)
摘要
Noisy labels are an unavoidable consequence of labeling processes and detecting them is an important step towards preventing performance degradations in Convolutional Neural Networks. Discarding noisy labels avoids a harmful memorization, while the associated image content can still be exploited in a semi-supervised learning (SSL) setup. Clean samples are usually identified using the small loss trick, i.e. they exhibit a low loss. However, we show that different noise distributions make the application of this trick less straightforward and propose to continuously relabel all images to reveal a discriminative loss against multiple distributions. SSL is then applied twice, once to improve the clean-noisy detection and again for training the final model. We design an experimental setup based on ImageNet32/64 for better understanding the consequences of representation learning with differing label noise distributions and find that non-uniform out-of-distribution noise better resembles real-world noise and that in most cases intermediate features are not affected by label noise corruption. Experiments in CIFAR-10/100, ImageNet32/64 and WebVision (real-world noise) demonstrate that the proposed label noise Distribution Robust Pseudo-Labeling (DRPL) approach gives substantial improvements over recent state-of-the-art. Code is available at https://git.io/JJ0PV.
更多查看译文
关键词
robust learning,noisy labels,convolutional neural networks,image content,semisupervised learning,SSL,discriminative loss,clean-noisy detection,out-of-distribution noise,real-world noise,label noise corruption,label noise distribution robust pseudolabeling
AI 理解论文
溯源树
样例
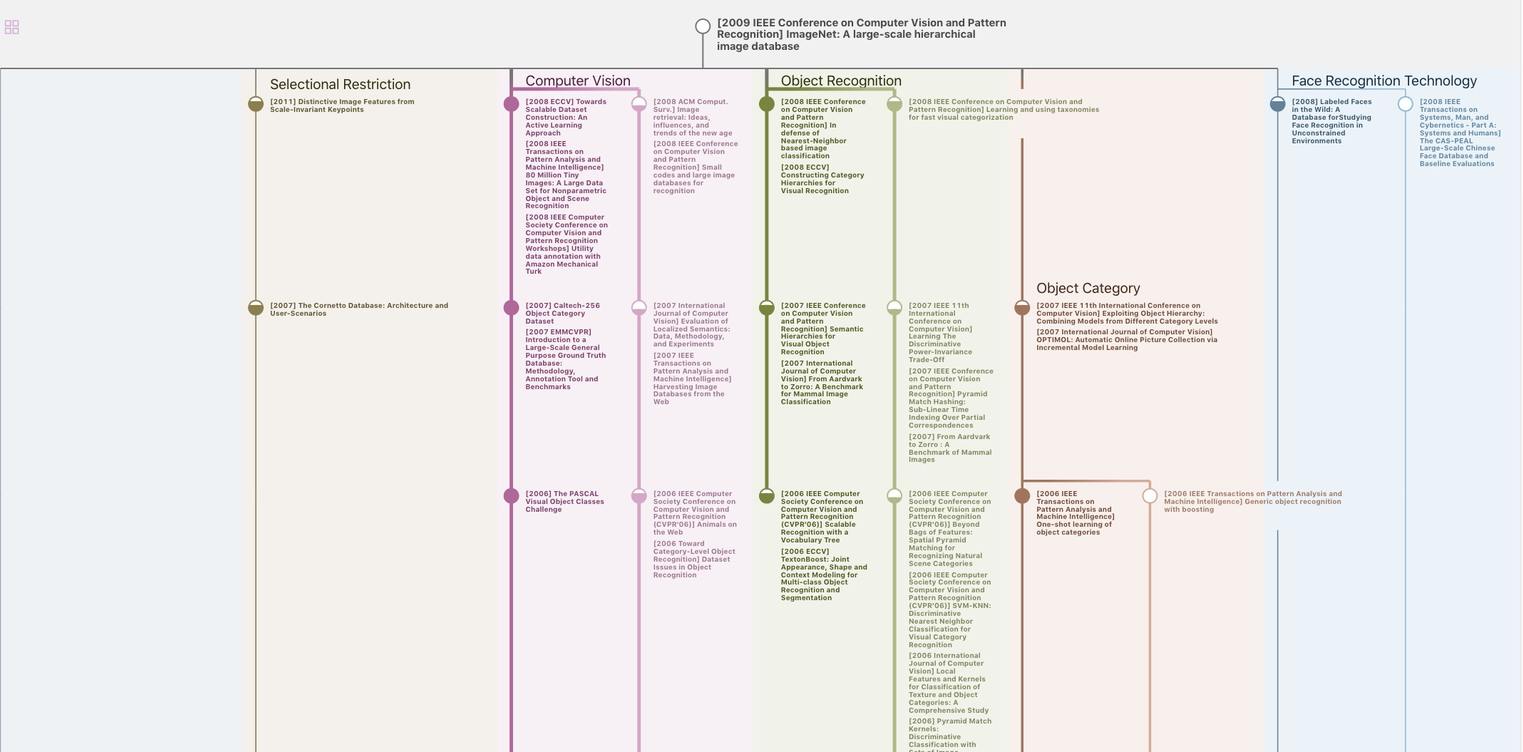
生成溯源树,研究论文发展脉络
Chat Paper
正在生成论文摘要