Automated Diagnosis of Atrial Fibrillation Using Principal Component Analysis-Discriminant Analysis.
TENCON(2019)
摘要
Atrial Fibrillation (AF) is quivering or irregular heartbeat by which the two upper chambers of the heart (atria) get affected resulting in disruption of blood flow throughout the body. If left untreated, AF can lead to several heart-related complications. In this study, we propose a signal processing model to automatically detect AF from electrocardiogram (ECG) signal at a shorter duration (9 s) that will be useful for server-based applications, remote monitoring and automated detection of AF. The ECG data collected from ‘CPSC-18 Challenge’ have been preprocessed with FIR filter followed by the extraction of important features. RR interval (RRI) and RRI combined with mean RS ratio have particularly been found to be useful in detecting AF. Principal Component Analysis-Discriminant Analysis (PCA-DA) along with two other popular classifiers have been applied on the extracted features. The PCA-DA based approach had been superior in the detection of AF giving an accuracy of 97% with sensitivity and specificity both being 0.97. The proposed approach can be beneficial for noninvasive and faster screening of AF.
更多查看译文
关键词
Atrial Fibrillation Detection,Biomedical Signal Processing,Machine Learning,Time domain features,PCA-DA
AI 理解论文
溯源树
样例
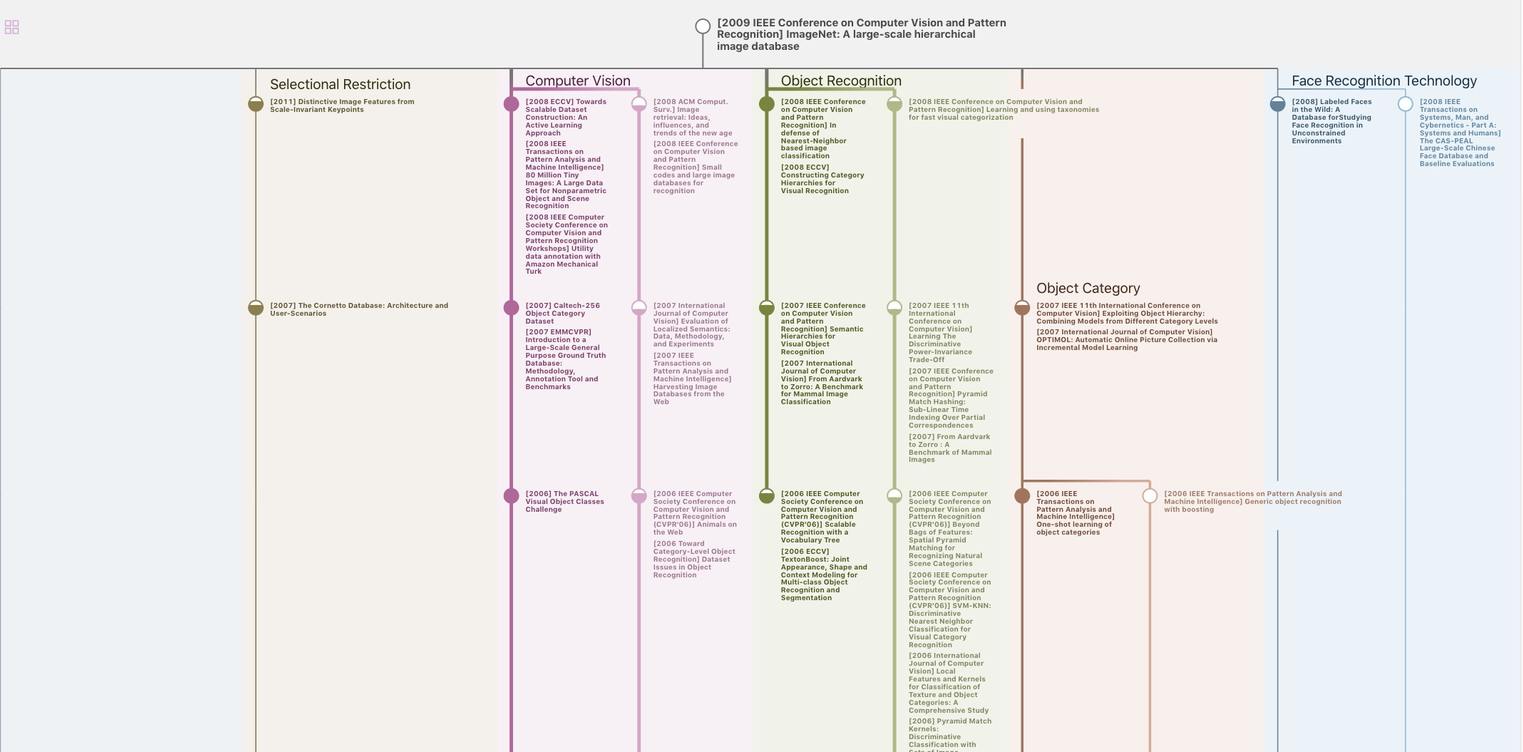
生成溯源树,研究论文发展脉络
Chat Paper
正在生成论文摘要