Why Higher Working Memory Capacity May Help You Learn: Sampling, Search, And Degrees Of Approximation
COGNITIVE SCIENCE(2019)
摘要
Algorithms for approximate Bayesian inference, such as those based on sampling (i.e., Monte Carlo methods), provide a natural source of models of how people may deal with uncertainty with limited cognitive resources. Here, we consider the idea that individual differences in working memory capacity (WMC) may be usefully modeled in terms of the number of samples, or "particles," available to perform inference. To test this idea, we focus on two recent experiments that report positive associations between WMC and two distinct aspects of categorization performance: the ability to learn novel categories, and the ability to switch between different categorization strategies ("knowledge restructuring"). In favor of the idea of modeling WMC as a number of particles, we show that a single model can reproduce both experimental results by varying the number of particles-increasing the number of particles leads to both faster category learning and improved strategy-switching. Furthermore, when we fit the model to individual participants, we found a positive association between WMC and best-fit number of particles for strategy switching. However, no association between WMC and best-fit number of particles was found for category learning. These results are discussed in the context of the general challenge of disentangling the contributions of different potential sources of behavioral variability.
更多查看译文
关键词
Working memory, Category learning, Knowledge partitioning, Strategy switching, Approximate Bayesian inference, Particle filtering
AI 理解论文
溯源树
样例
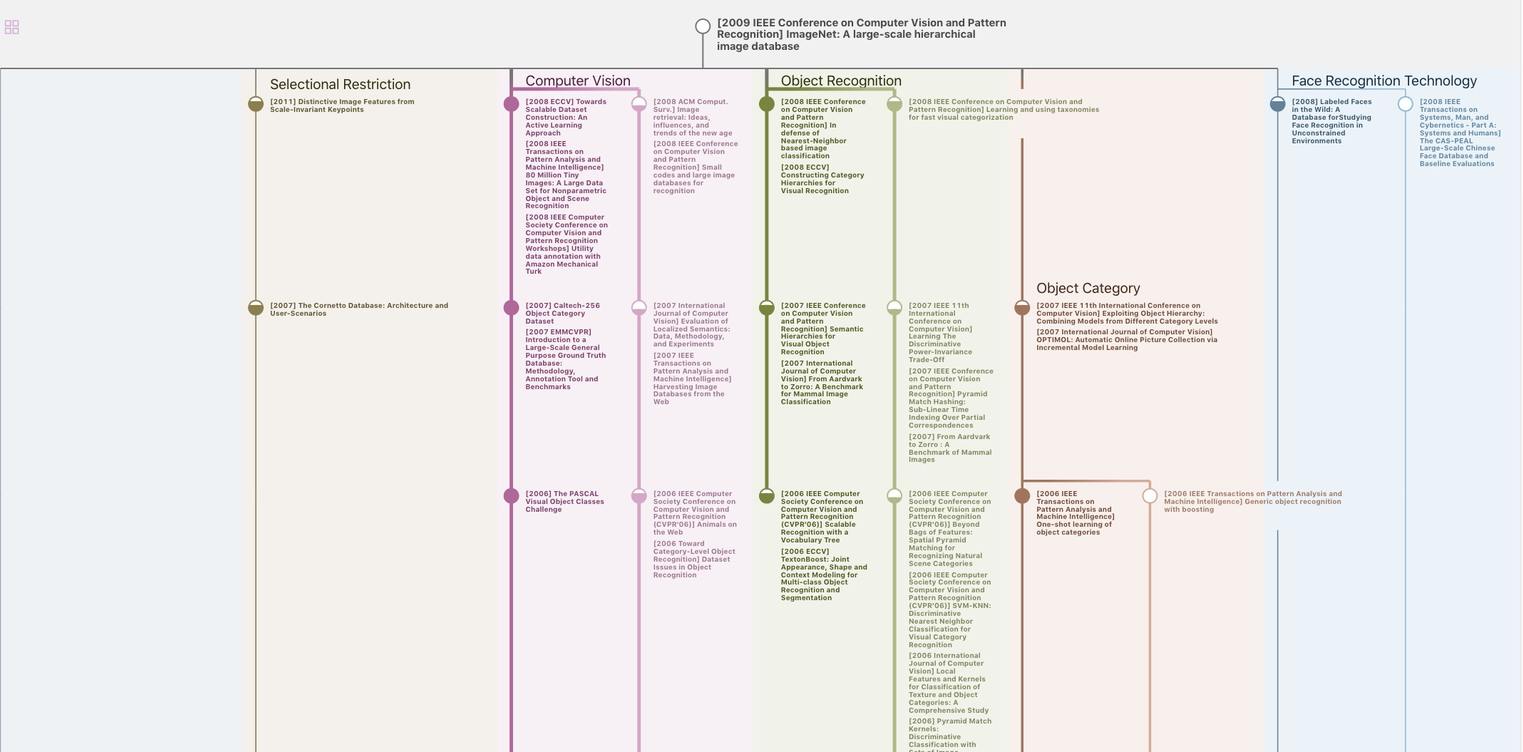
生成溯源树,研究论文发展脉络
Chat Paper
正在生成论文摘要