Optimal Approximate Sampling from Discrete Probability Distributions
Proceedings of the ACM on Programming Languages(2020)
摘要
This paper addresses a fundamental problem in random variate generation: given access to a random source that emits a stream of independent fair bits, what is the most accurate and entropy-efficient algorithm for sampling from a discrete probability distribution (p_1, …, p_n), where the probabilities of the output distribution (p̂_1, …, p̂_n) of the sampling algorithm must be specified using at most k bits of precision? We present a theoretical framework for formulating this problem and provide new techniques for finding sampling algorithms that are optimal both statistically (in the sense of sampling accuracy) and information-theoretically (in the sense of entropy consumption). We leverage these results to build a system that, for a broad family of measures of statistical accuracy, delivers a sampling algorithm whose expected entropy usage is minimal among those that induce the same distribution (i.e., is "entropy-optimal") and whose output distribution (p̂_1, …, p̂_n) is a closest approximation to the target distribution (p_1, …, p_n) among all entropy-optimal sampling algorithms that operate within the specified k-bit precision. This optimal approximate sampler is also a closer approximation than any (possibly entropy-suboptimal) sampler that consumes a bounded amount of entropy with the specified precision, a class which includes floating-point implementations of inversion sampling and related methods found in many software libraries. We evaluate the accuracy, entropy consumption, precision requirements, and wall-clock runtime of our optimal approximate sampling algorithms on a broad set of distributions, demonstrating the ways that they are superior to existing approximate samplers and establishing that they often consume significantly fewer resources than are needed by exact samplers.
更多查看译文
关键词
discrete random variables, random variate generation
AI 理解论文
溯源树
样例
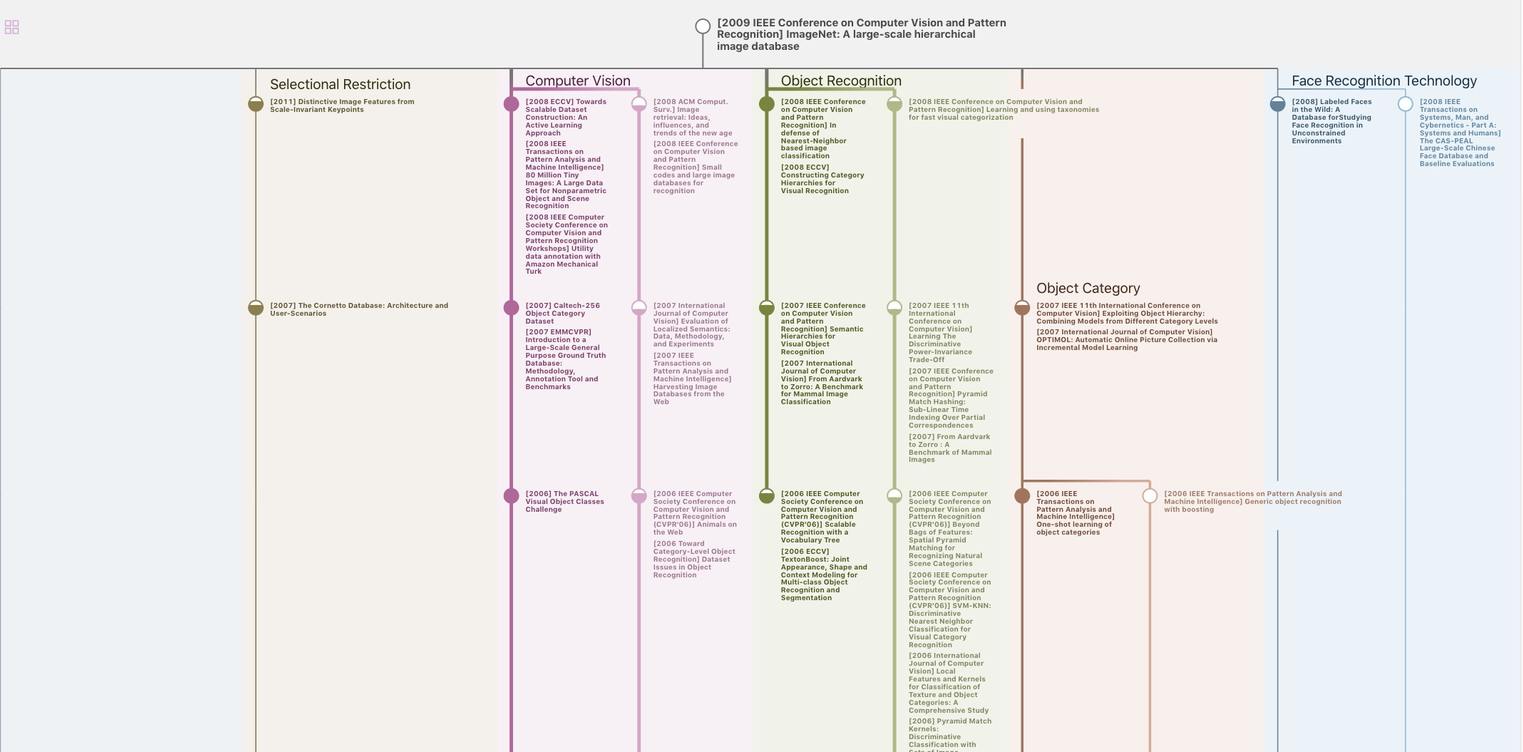
生成溯源树,研究论文发展脉络
Chat Paper
正在生成论文摘要