Prediction of Hepatic Steatosis (Fatty Liver) using Machine Learning
Proceedings of the 2019 3rd International Conference on Computational Biology and Bioinformatics(2019)
摘要
The exact reasons of the incidences of fatty liver disease (Hepatic Steatosis, HS) are not known. Heavy alcohol use leads to alcoholic steatohepatitis (alcoholic fatty liver disease). Contrarily, Non-alcoholic fatty liver disease (NAFLD), is a condition of fat build-up in the liver when alcohol consumption is not the cause. However, certain clinical and demographic factors impact the incidence of HS. We evaluated the predictive capability of fatty liver using a computational model and NHANES-III data. Six predictor variables (age, gender, BMI, triglycerides, HDL, and total cholesterol) and one output variable (HS) were used. The challenge of class imbalanced data was handled using SMOTE algorithm combined with Gower's distance. Data were divided into training and test in 70:30 ratio with 8,903 and 3,816 observations respectively. Three families of models were trained: SVM (Fine and Medium Gaussian SVM), Bagged Trees, Boosted Tree (Gentle and ADA Boosted Tree). 10-fold cross-validation was used. Of the five models, 'Gentle Boosted Tree' model provided the highest average testing accuracy of 79.03% (79%). The average sensitivity, specificity, and AUC of the 'Gentle Boosted Tree' model were 75.88%, 81.86% and 0.79 respectively. The novelty of this paper lies in developing and testing algorithms with imbalanced data for prediction of fatty liver condition.
更多查看译文
关键词
Bagged trees, Class-imbalanced data, Gentle Boosting, Gower's distance, Hepatic Steatosis, Non-alcoholic fatty liver disease, Prediction, SMOTE, SVM
AI 理解论文
溯源树
样例
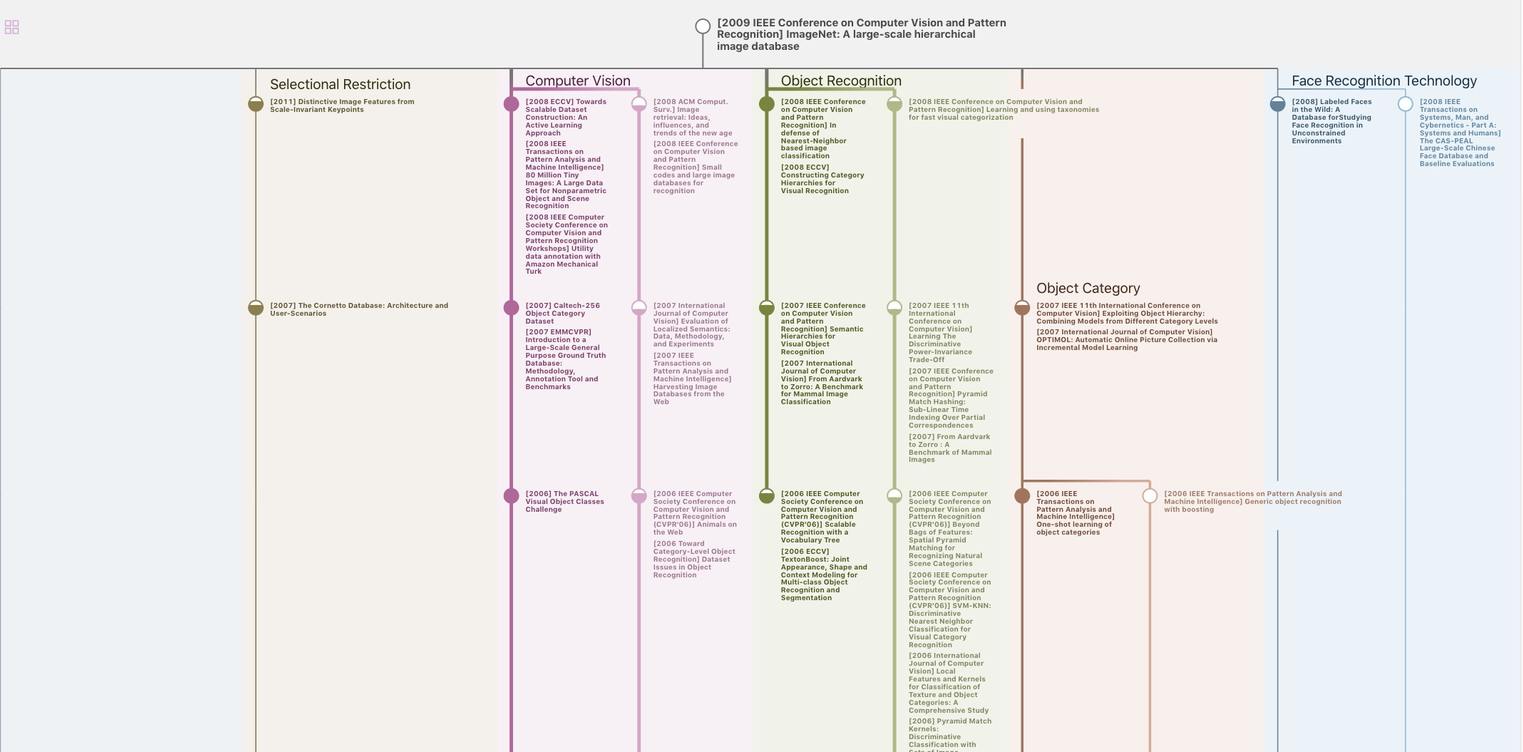
生成溯源树,研究论文发展脉络
Chat Paper
正在生成论文摘要