Spatio-temporal Multi-level Fusion for Human Action Recognition
Proceedings of the Tenth International Symposium on Information and Communication Technology(2019)
摘要
Two-stream convolutional networks have achieved great success for action recognition tasks. In this paper, we propose a spatiotemporal network that integrates the spatial and temporal features at multi-level to model the correlation between spatial and temporal information. Based on TSN model [16] where videos are divided into segments, our model integrates spatio-temporal information at either local or global levels. At local levels, temporal information is transferred to spatial stream in each segment. Considering at a global level, we integrate features of entire action extracted from two streams to obtain the final action representation. Moreover, in order to take into consideration the chronological sequence of the segments, we propose strategies for segment aggregation by using Conv3D and LSTM (Long-short term memory). In the training process, we also applied and evaluated several strategies such as auxiliary classifier, cross modality initialization to improve the convergence rate. Experimentation on the standard dataset UCF-101 (split-1) demonstrates the effectiveness of proposed network. Our model achieved an accuracy of 87.1% for spatial network, higher than TSN (85.5%) thanks to segment aggregation strategy with LSTM seq-to-seq. In proposed two-stream network, the strategy of multi-level fusion allows to get a better model in comparing with network using only global fusion with an improvement of 1.4% in accuracy and 1.2% in F1-score. Our two-stream network obtained also very promising results with an accuracy of 92.57%.
更多查看译文
关键词
3D convolution, Action Recognition, LSTM, Temporal Segment Networks
AI 理解论文
溯源树
样例
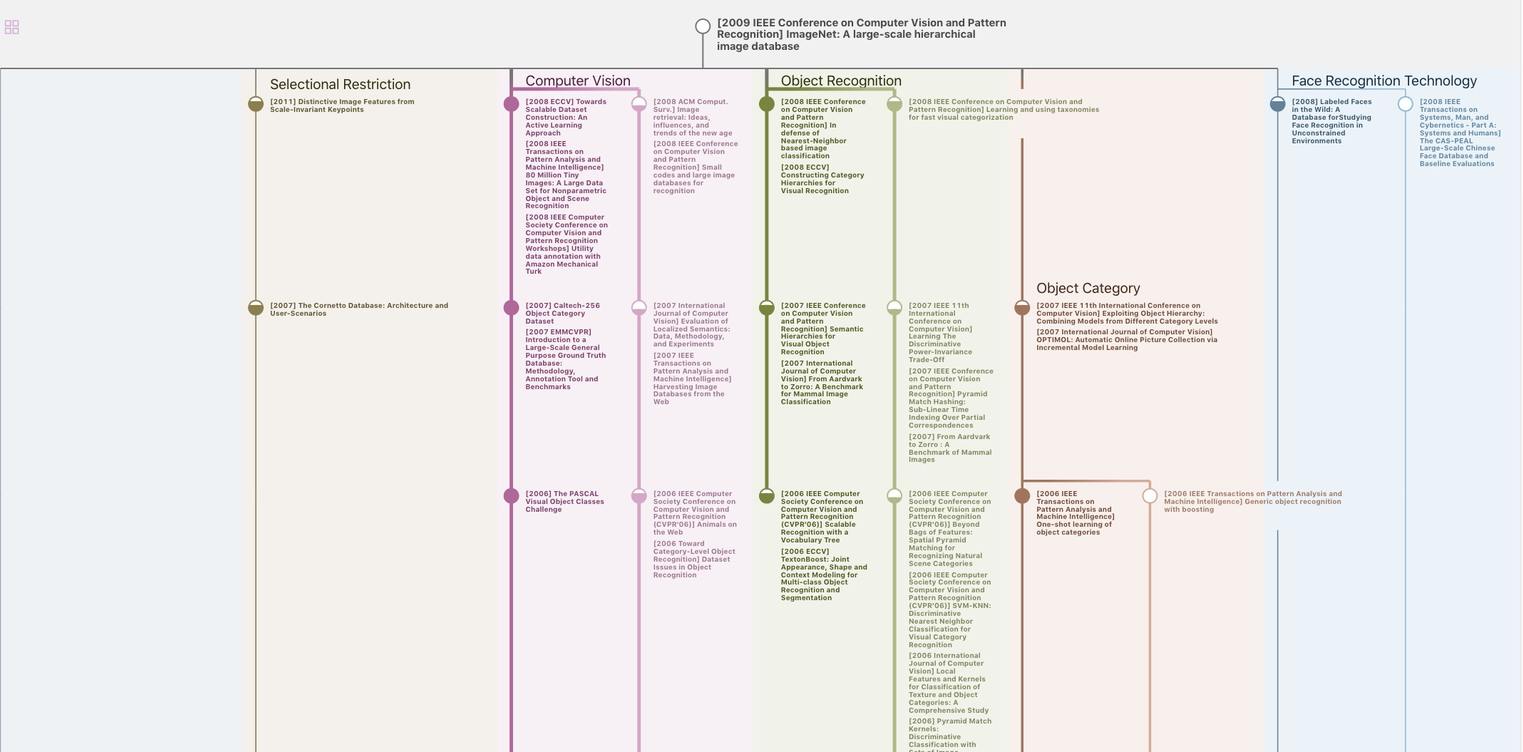
生成溯源树,研究论文发展脉络
Chat Paper
正在生成论文摘要