Detecting Web Attacks using Stacked Denoising Autoencoder and Ensemble Learning Methods
Proceedings of the Tenth International Symposium on Information and Communication Technology(2019)
摘要
Web-based anomalies remains a serious security threat on the Internet. This paper proposes the use of Sum Rule and Xgboost to combine the outputs related to various Stacked Denoising Autoencoders (SDAEs) in order to detect abnormal HTTP queries. Sum Rule and Xgboost inherit the distinct advantage of SDAE that does not require handcrafted features to be extracted. Furthermore, these methods can cope with the changing web vulnerabilities, where malicious code is added into different parts of the request header and body. Experiments were carried out on the DVWA dataset and the dataset that obtained from a real-world application. Sum Rule and Xgboost demonstrate to achieve higher F1-score as compared to the state-of-the-art Regularized Deep Autoencoders, Isolation Forest, C4.5 decision tree and Long Short-term Memory network.
更多查看译文
关键词
Anomaly Detection, Stacked Denoising Autoencoder, Sum Rule, Web attack detection, Xgboost
AI 理解论文
溯源树
样例
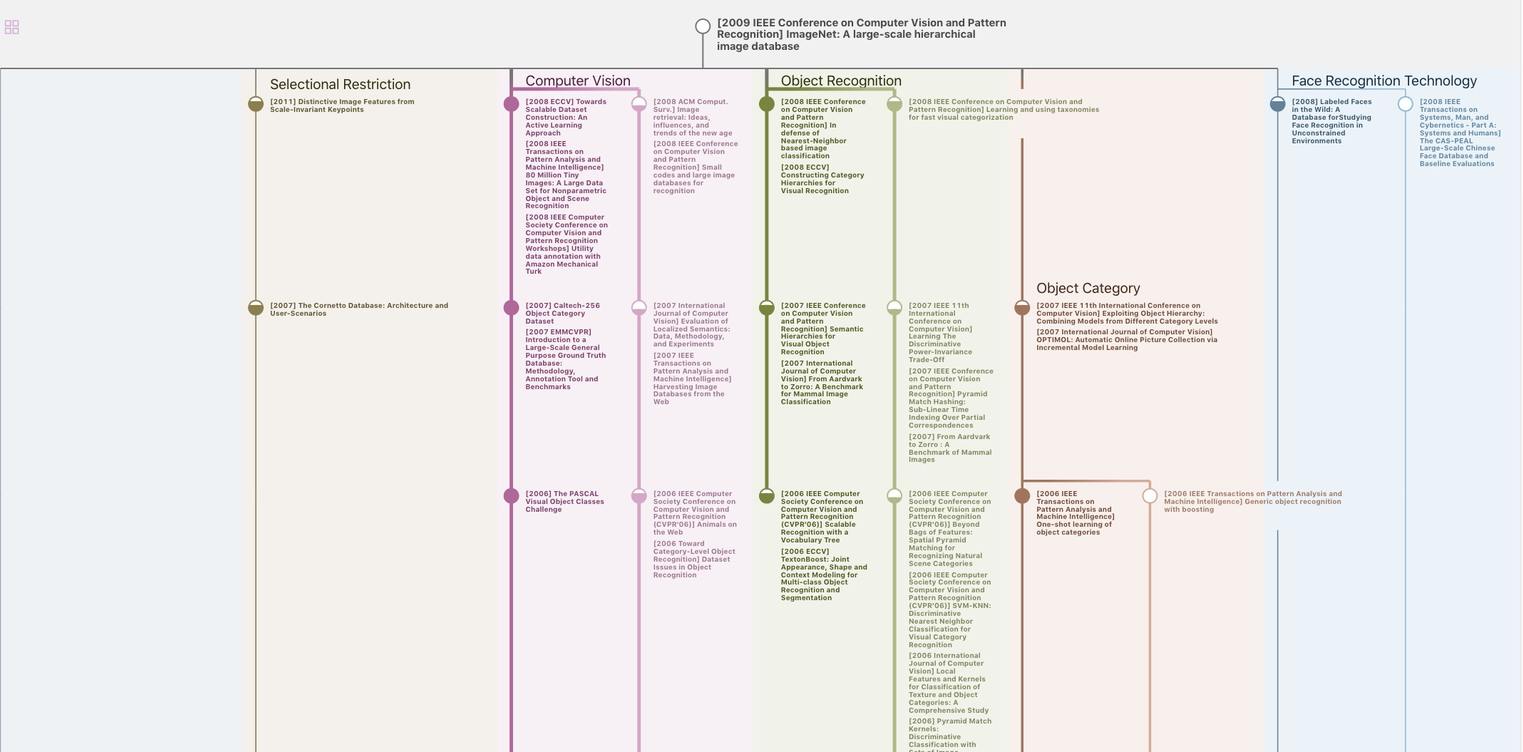
生成溯源树,研究论文发展脉络
Chat Paper
正在生成论文摘要