Heterogeneity-aware and Communication-Efficient Distributed Statistical Inference
arXiv (Cornell University)(2019)
摘要
Summary In multicentre research, individual-level data are often protected against sharing across sites. To overcome the barrier of data sharing, many distributed algorithms, which only require sharing aggregated information, have been developed. The existing distributed algorithms usually assume the data are homogeneously distributed across sites. This assumption ignores the important fact that the data collected at different sites may come from various subpopulations and environments, which can lead to heterogeneity in the distribution of the data. Ignoring the heterogeneity may lead to erroneous statistical inference. We propose distributed algorithms which account for the heterogeneous distributions by allowing site-specific nuisance parameters. The proposed methods extend the surrogate likelihood approach (Wang et al. 2017; Jordan et al. 2018) to the heterogeneous setting by applying a novel density ratio tilting method to the efficient score function. The proposed algorithms maintain the same communication cost as existing communication-efficient algorithms. We establish a nonasymptotic risk bound for the proposed distributed estimator and its limiting distribution in the two-index asymptotic setting, which allows both sample size per site and the number of sites to go to infinity. In addition, we show that the asymptotic variance of the estimator attains the Cramér–Rao lower bound when the number of sites is smaller in rate than the sample size at each site. Finally, we use simulation studies and a real data application to demonstrate the validity and feasibility of the proposed methods.
更多查看译文
关键词
Data integration,Distributed inference,Efficient score,Surrogate likelihood,Two-index asymptotics
AI 理解论文
溯源树
样例
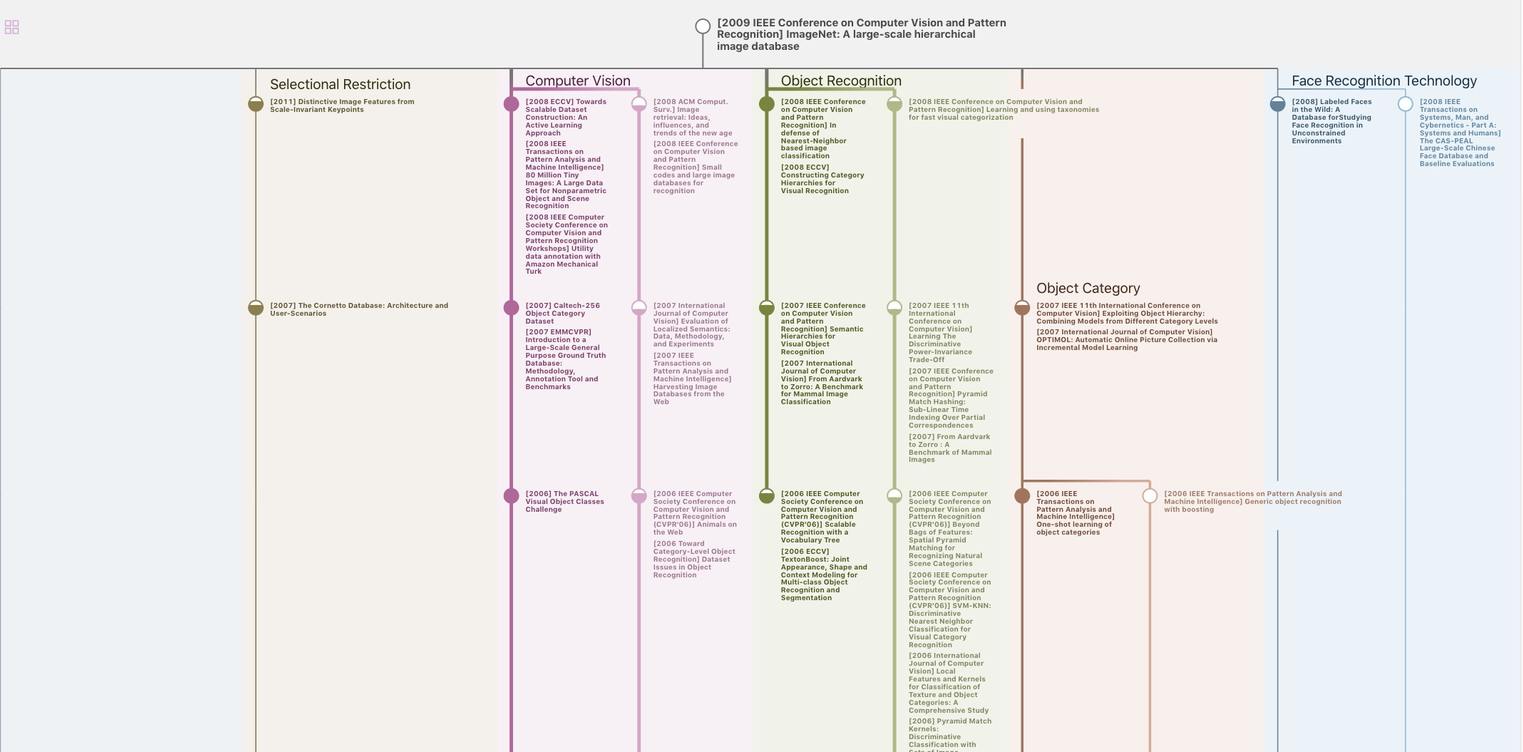
生成溯源树,研究论文发展脉络
Chat Paper
正在生成论文摘要