Learning Reserve Prices in Second-Price Auctions
arxiv(2022)
摘要
This paper proves the tight sample complexity of {\sf Second-Price Auction with Anonymous Reserve}, up to a logarithmic factor, for each of all the value distribution families studied in the literature: $[0,\, 1]$-bounded, $[1,\, H]$-bounded, regular, and monotone hazard rate (MHR). Remarkably, the setting-specific tight sample complexity $\mathsf{poly}(\varepsilon^{-1})$ depends on the precision $\varepsilon \in (0, 1)$, but not on the number of bidders $n \geq 1$. Further, in the two bounded-support settings, our learning algorithm allows {\em correlated} value distributions. In contrast, the tight sample complexity $\tilde{\Theta}(n) \cdot \mathsf{poly}(\varepsilon^{-1})$ of {\sf Myerson Auction} proved by Guo, Huang and Zhang (STOC~2019) has a nearly-linear dependence on $n \geq 1$, and holds only for {\em independent} value distributions in every setting. We follow a similar framework as the Guo-Huang-Zhang work, but replace their information theoretical arguments with a direct proof.
更多查看译文
AI 理解论文
溯源树
样例
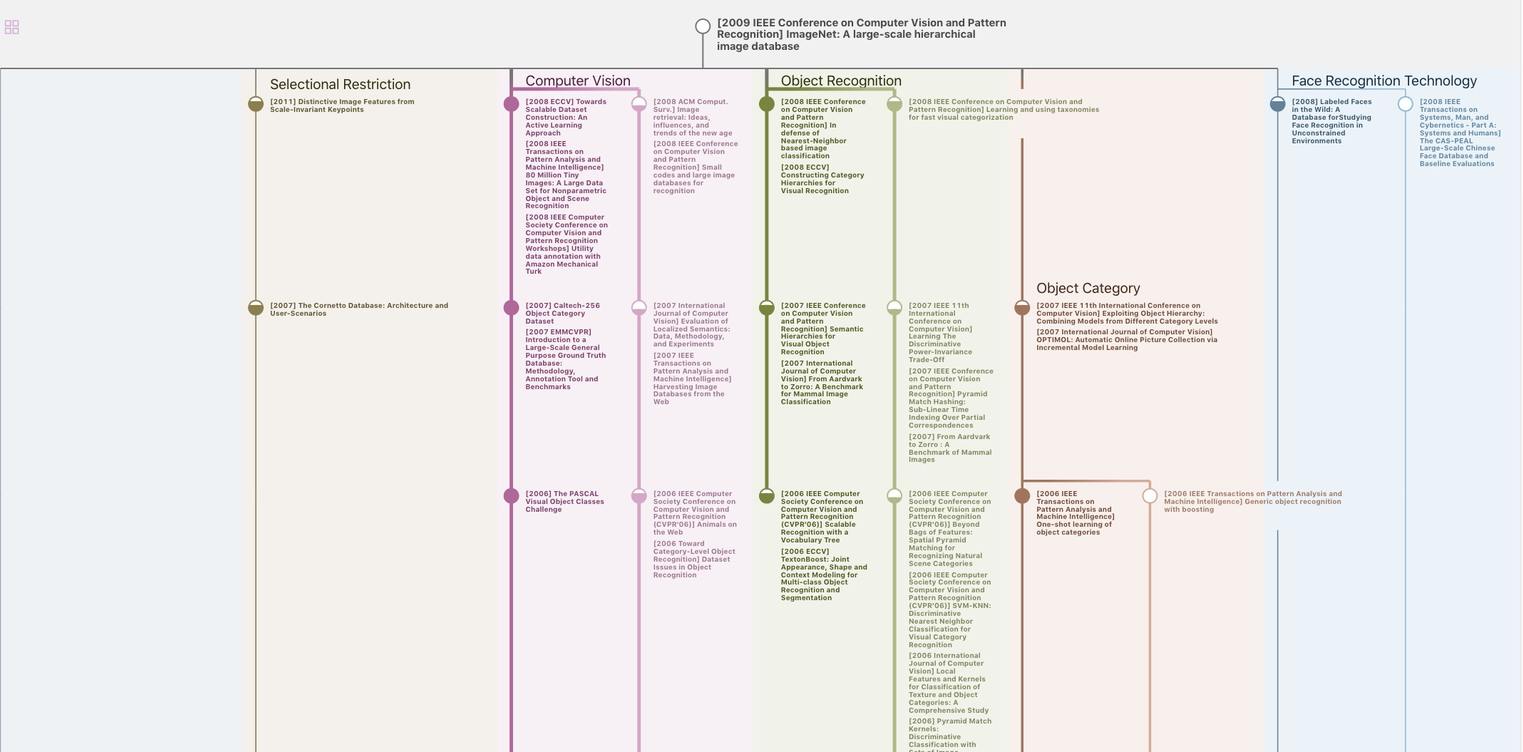
生成溯源树,研究论文发展脉络
Chat Paper
正在生成论文摘要