Estimating Treatment Importance in Multidrug‐resistant Tuberculosis Using Targeted Learning: an Observational Individual Patient Data Network Meta‐analysis
Biometrics(2020)
摘要
Persons with multidrug-resistant tuberculosis (MDR-TB) have a disease resulting from a strain of tuberculosis (TB) that does not respond to at least isoniazid and rifampicin, the two most effective anti-TB drugs. MDR-TB is always treated with multiple antimicrobial agents. Our data consist of individual patient data from 31 international observational studies with varying prescription practices, access to medications, and distributions of antibiotic resistance. In this study, we develop identifiability criteria for the estimation of a global treatment importance metric in the context where not all medications are observed in all studies. With stronger causal assumptions, this treatment importance metric can be interpreted as the effect of adding a medication to the existing treatments. We then use this metric to rank 15 observed antimicrobial agents in terms of their estimated add-on value. Using the concept of transportability, we propose an implementation of targeted maximum likelihood estimation, a doubly robust and locally efficient plug-in estimator, to estimate the treatment importance metric. A clustered sandwich estimator is adopted to compute variance estimates and produce confidence intervals. Simulation studies are conducted to assess the performance of our estimator, verify the double robustness property, and assess the appropriateness of the variance estimation approach.
更多查看译文
关键词
double robustness,individual patient data,meta-analysis,multidrug-resistant tuberculosis,targeted maximum likelihood estimation,transportability,treatment importance
AI 理解论文
溯源树
样例
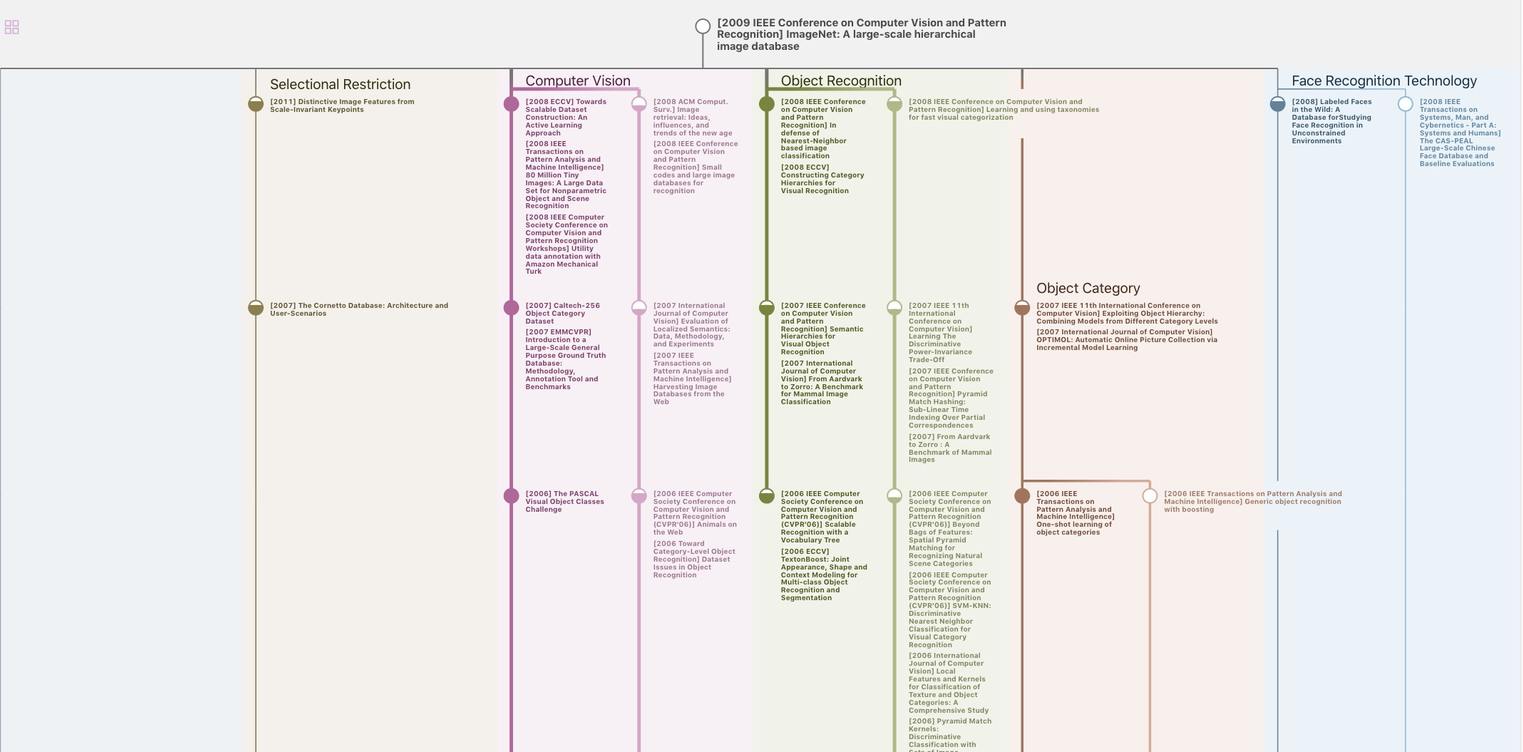
生成溯源树,研究论文发展脉络
Chat Paper
正在生成论文摘要