Glioma Grading Prediction by Exploring Radiomics and Deep Learning Features
Proceedings of the Third International Symposium on Image Computing and Digital Medicine(2019)
摘要
Glioma grading is critical to clinical prognosis and survival prediction. In this paper, we propose a noninvasive method which combines radiomics and deep learning features to conduct glioma grading. By integrating radiomics features with high-level deep learning features, a more comprehensive representation of the images was constructed. First, different types of radiomics features were extracted from region of interest (ROI) of the image. A VGG-16 model pretrained on ImageNet was finetuned for the extraction of the deep learning features. Subsequent feature selection was performed to obtain three kinds of optimal feature subsets: radiomics features only, deep learning features only, and combined features. Finally, these feature subsets were used to train three classifiers including logistic regression (LR), support vector machine (SVM) and linear discriminant analysis (LDA) to predict the glioma grade. Our method was evaluated on an open dataset, BraTS 2018, which contains 285 subjects from multiple institutions. The proposed combination method exhibits the best AUC value of 94.4% when compared with that use only radiomics or deep learning features.
更多查看译文
关键词
Deep learning, Feature extraction, Glioma grading, Magnetic resonance imaging, Radiomics
AI 理解论文
溯源树
样例
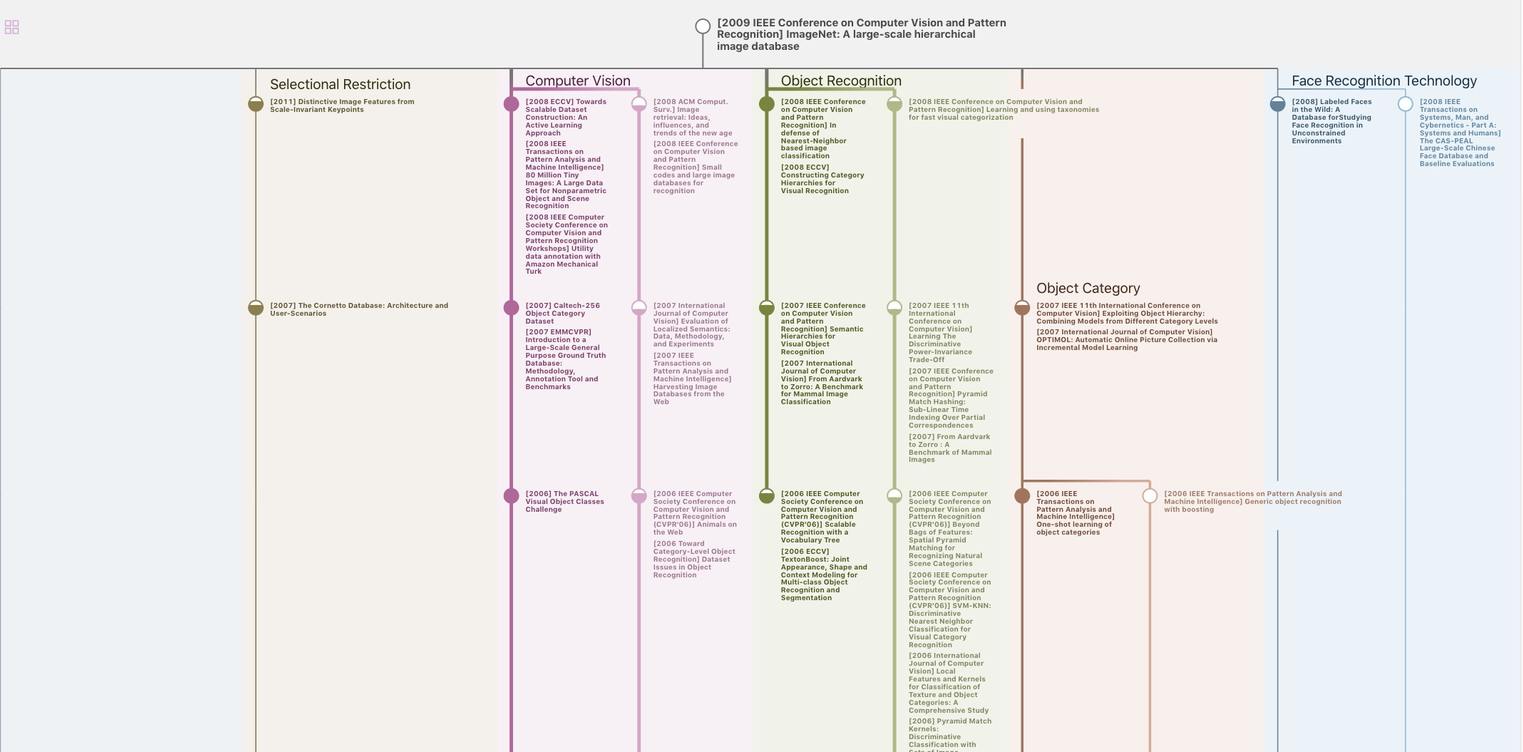
生成溯源树,研究论文发展脉络
Chat Paper
正在生成论文摘要