A Simple and Effective Framework for Pairwise Deep Metric Learning
European Conference on Computer Vision(2020)
摘要
Deep metric learning (DML) has received much attention in deep learning due to its wide applications in computer vision. Previous studies have focused on designing complicated losses and hard example mining methods, which are mostly heuristic and lack of theoretical understanding. In this paper, we cast DML as a simple pairwise binary classification problem that classifies a pair of examples as similar or dissimilar. It identifies the most critical issue in this problem—imbalanced data pairs. To tackle this issue, we propose a simple and effective framework to sample pairs in a batch of data for updating the model. The key to this framework is to define a robust loss for all pairs over a mini-batch of data, which is formulated by distributionally robust optimization. The flexibility in constructing the uncertainty decision set of the dual variable allows us to recover state-of-the-art complicated losses and also to induce novel variants. Empirical studies on several benchmark data sets demonstrate that our simple and effective method outperforms the state-of-the-art results.
更多查看译文
关键词
Deep metric learning, Distributed robust learning, Data imbalance
AI 理解论文
溯源树
样例
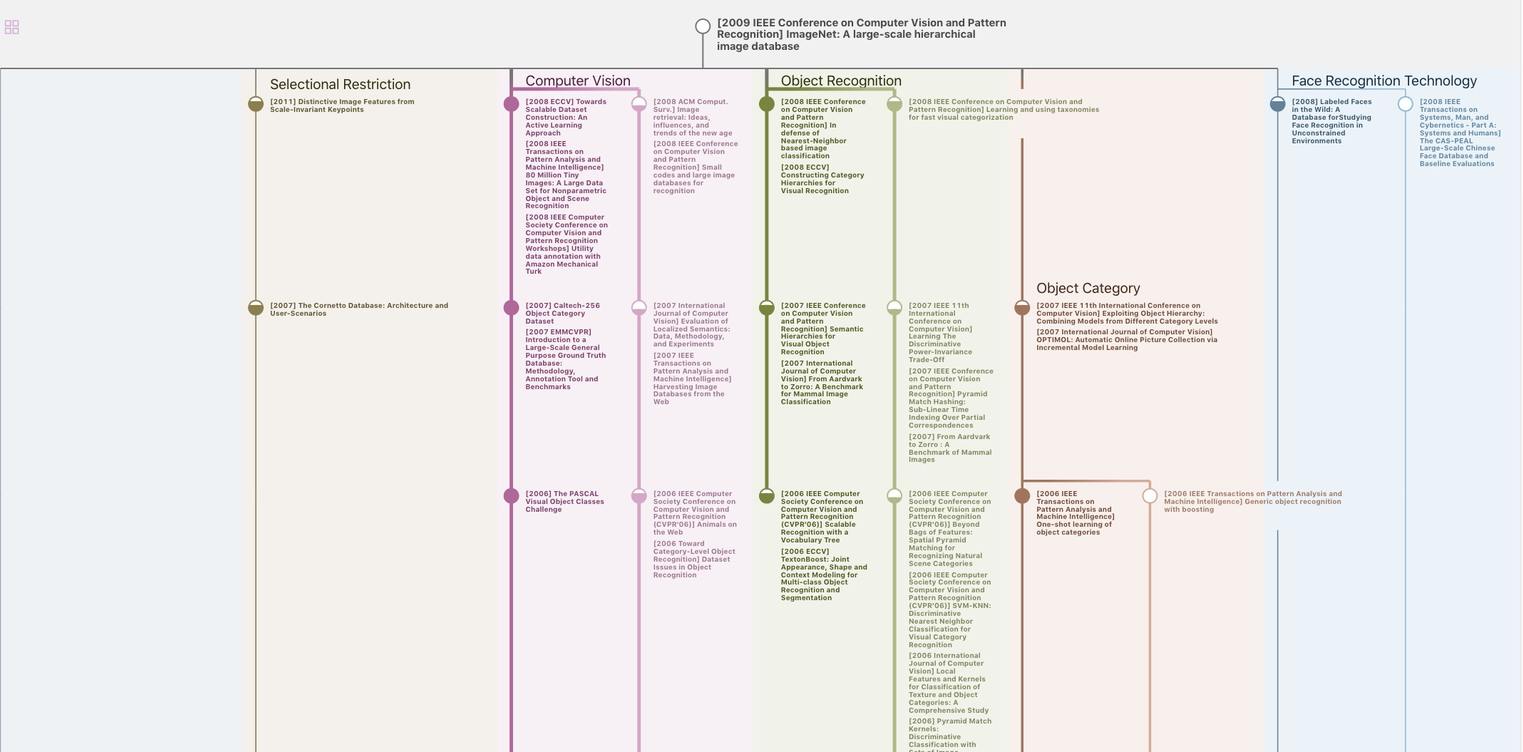
生成溯源树,研究论文发展脉络
Chat Paper
正在生成论文摘要