Hierarchical Weakly Supervised Learning for Residential Area Semantic Segmentation in Remote Sensing Images.
IEEE geoscience and remote sensing letters(2020)
摘要
Residential-area segmentation is one of the most fundamental tasks in the field of remote sensing. Recently, fully supervised convolutional neural network (CNN)-based methods have shown superiority in the field of semantic segmentation. However, a serious problem for those CNN-based methods is that pixel-level annotations are expensive and laborious. In this study, a novel hierarchical weakly supervised learning (HWSL) method is proposed to realize pixel-level semantic segmentation in remote sensing images. First, a weakly supervised hierarchical saliency analysis is proposed to capture a sequence of class-specific hierarchical saliency maps by computing the gradient maps with respect to the middle layers of the CNN. Then, superpixels and low-rank matrix recovery are introduced to highlight the common salient areas and fuse class-specific saliency maps with adaptive weights. Finally, a subtraction operation between class-specific saliency maps is conducted to generate hierarchical residual saliency maps and fulfill residential-area segmentation. Comprehensive evaluations with two remote sensing data sets and comparison with seven methods validate the superiority of the proposed HWSL model.
更多查看译文
关键词
Image segmentation,Semantics,Remote sensing,Task analysis,Image color analysis,Feature extraction,Fuses,Deep learning,remote sensing,saliency analysis,semantic segmentation,weakly supervised
AI 理解论文
溯源树
样例
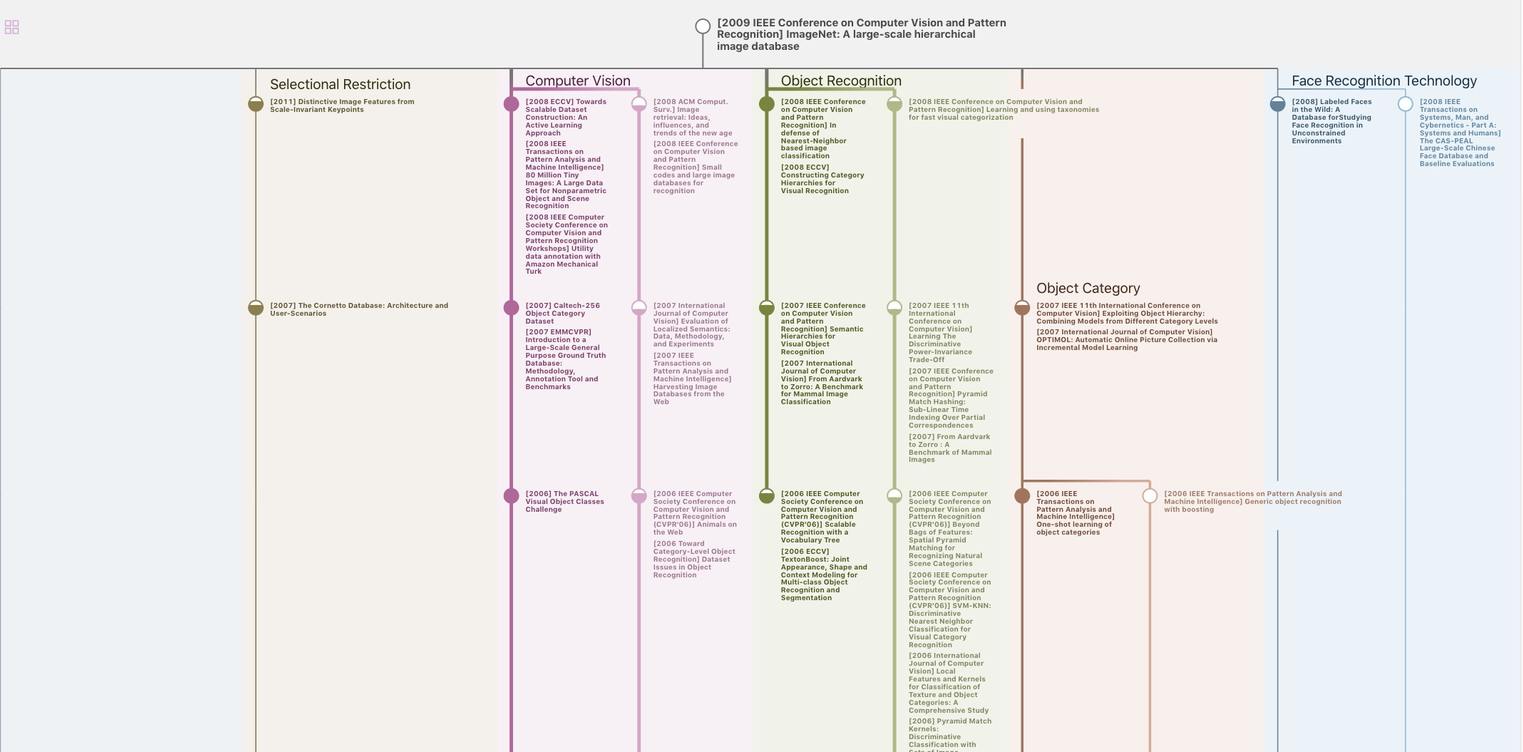
生成溯源树,研究论文发展脉络
Chat Paper
正在生成论文摘要