Dimensionality Reduction In The Presence Of Highly Correlated Variables For Random Forests: Wetland Case Study
2019 IEEE INTERNATIONAL GEOSCIENCE AND REMOTE SENSING SYMPOSIUM (IGARSS 2019)(2019)
摘要
Random Forest variable importance measures such as mean decrease in accuracy or Gini index are often used to reduce the number of predictor variables for a given classification problem. However, previous studies suggest that Random Forest variable ranking measures are biased, particularly in the presence of highly correlated variables. As a result, variables selected after ranking might not achieve the highest possible classification accuracy. Here, we introduce a new variable importance metric based on a simple statistical measure of association, which can be interpreted as a (statistically) low order measure of class separability. The method is applied to a multi-temporal/multi-sensor data set used to classify wetland types. Results show that variable reduction using the suggested measure results in higher accuracies compared to variable reduction based on the mean decrease in accuracy or Gini index, which fail to rank variables by true importance due to the effects of correlation.
更多查看译文
关键词
Random Forests, variable reduction, wetland, classification, predictor variables
AI 理解论文
溯源树
样例
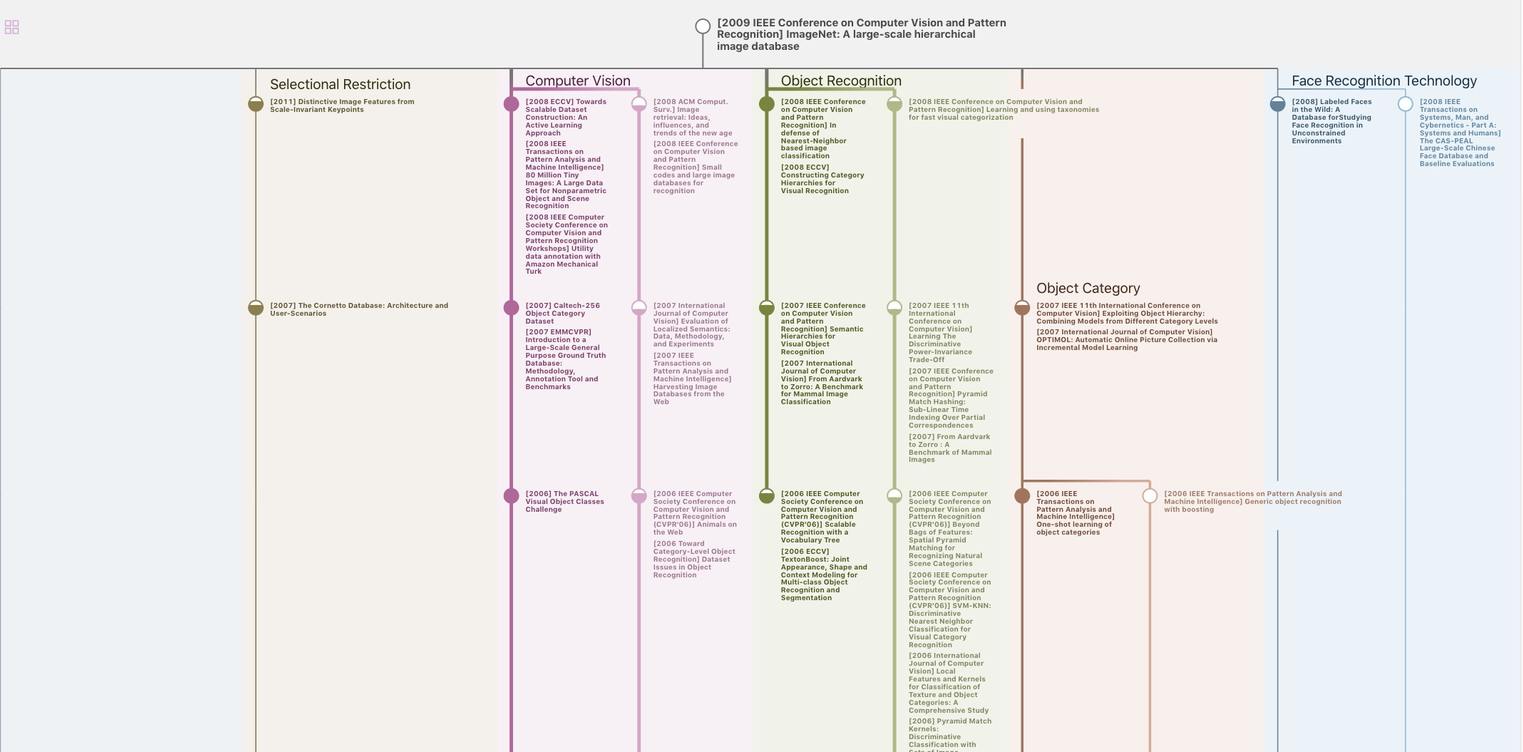
生成溯源树,研究论文发展脉络
Chat Paper
正在生成论文摘要