Gender-based speaker recognition from speech signals using GMM model
MODERN PHYSICS LETTERS B(2019)
摘要
Speech is a convenient medium for communication among human beings. Speaker recognition is a process of automatically recognizing the speaker by processing the information included in the speech signal. In this paper, a new approach is proposed for speaker recognition through speech signal. Here, a two-level approach is proposed. In the first-level, the gender of the speaker is recognized, and in the second-level speaker is recognized based on recognized gender at first-level. After recognizing the gender of the speaker, search space is reduced to half for the second-level as speaker recognition system searches only in a set of speech signals belonging to identified gender. To identify gender, gender-specific features: Mel Frequency Cepstral Coefficients (MFCC) and pitch are used. Speaker is recognized by using speaker specific features: MFCC, Pitch and RASTA-PLP. Support Vector Machine (SVM) and Gaussian Mixture Model (GMM) classifiers are used for identifying the gender and recognizing the speaker, respectively. Experiments are performed on speech signals of two databases: "IIT-Madras speech synthesis and recognition" (containing speech samples spoken by eight male and eight female speakers of eight different regions in English language) and "ELSDSR" (containing speech samples spoken by five male and five female in English language). Experimentally, it is observed that by using two-level approach, time taken for speaker recognition is reduced by 30-32% as compared to the approach when speaker is recognized without identifying the gender (single-level approach). The accuracy of speaker recognition in this proposed approach is also improved from 99.7% to 99.9% as compared to single-level approach. It is concluded through the experiments that speech signal of a minimum 1.12 duration (after neglecting silence parts) is sufficient for recognizing the speaker.
更多查看译文
关键词
Gender recognition,speaker recognition,GMM,SVM,MFCC
AI 理解论文
溯源树
样例
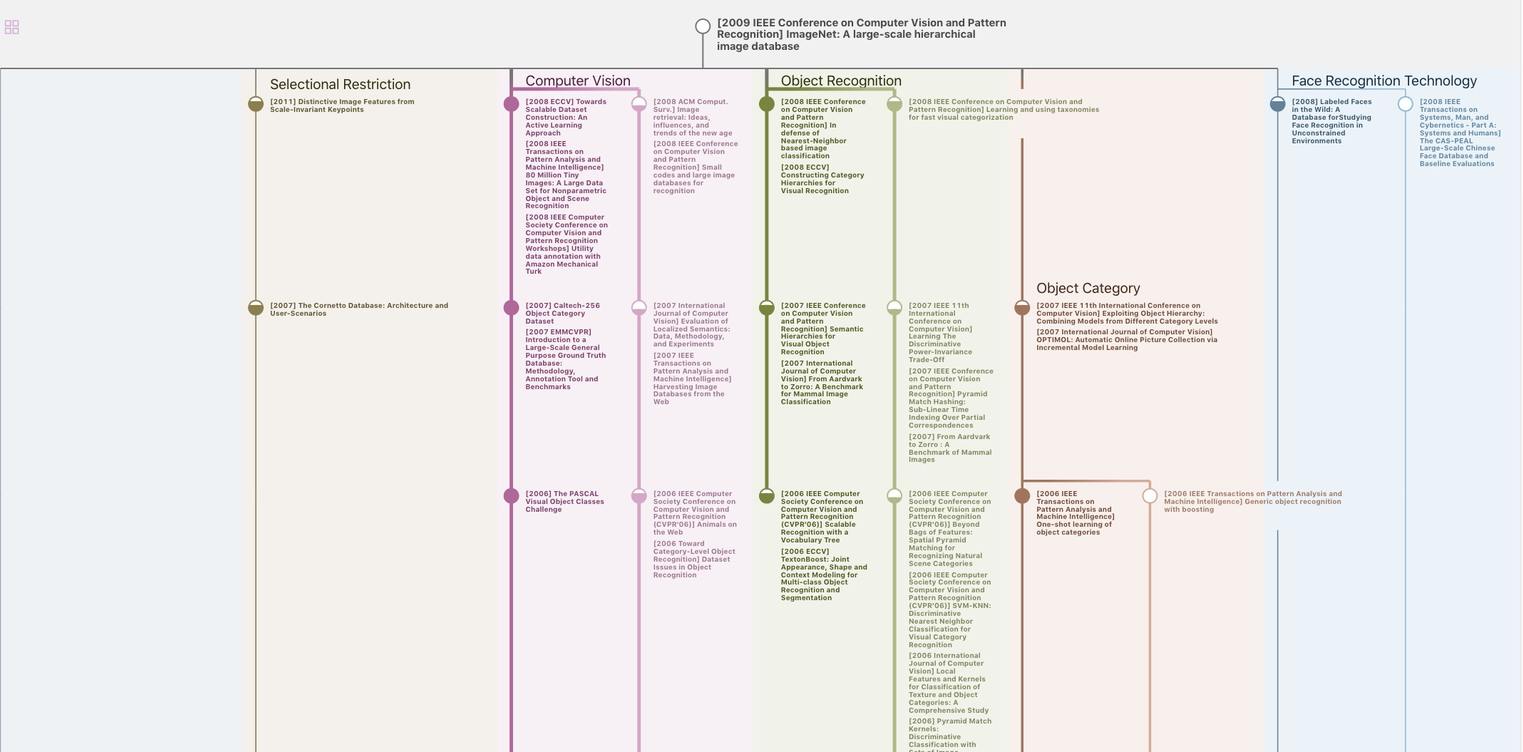
生成溯源树,研究论文发展脉络
Chat Paper
正在生成论文摘要