Deep Convolutional Neural Networks for Comprehensive Structural Health Monitoring and Damage Detection
Structural Health Monitoring 2019(2019)
摘要
Vision-based structural health monitoring (SHM) has become an important approach to recognize and evaluate structural damage after natural disasters. Deep convolutional neural networks (CNNs) have recently attained a breakthrough in computer vision field, in particular for image classification task. In this article, we adopted deep residual neural network (ResNet) whose residual representations and shortcut connections mechanism has gained significant performance in various computer vision tasks. In addition, we applied transfer learning due to a relatively small number of training images. To test our approach, we used the dataset from the 2018 PEER Hub ImageNet Challenge dis- tributed by Pacific Earthquake Engineering Research Center. This challenge proposed eight structural damage detection tasks: scene classification, damage check, spalling condition, material type, collapse check, component type, damage level and damage type which can be categorized as binary and multi-class (3 or 4 classes) classification problems. Our experiments with eight different tasks showed that reliable classification can be obtained for some tasks. Corresponding above eight tasks, classification accuracy varied from 63.1% to 99.4%. Our approach has attained third place for overall tasks in this challenge. Through the individual observation of training dataset, it is found that there are a large number of confusing images. Therefore, it is believed that the accuracy will be improved after making a precise training data.
更多查看译文
关键词
Structural Damage Detection,Defect Detection,Vibration-based Damage Identification,Deep Learning,Crack Detection
AI 理解论文
溯源树
样例
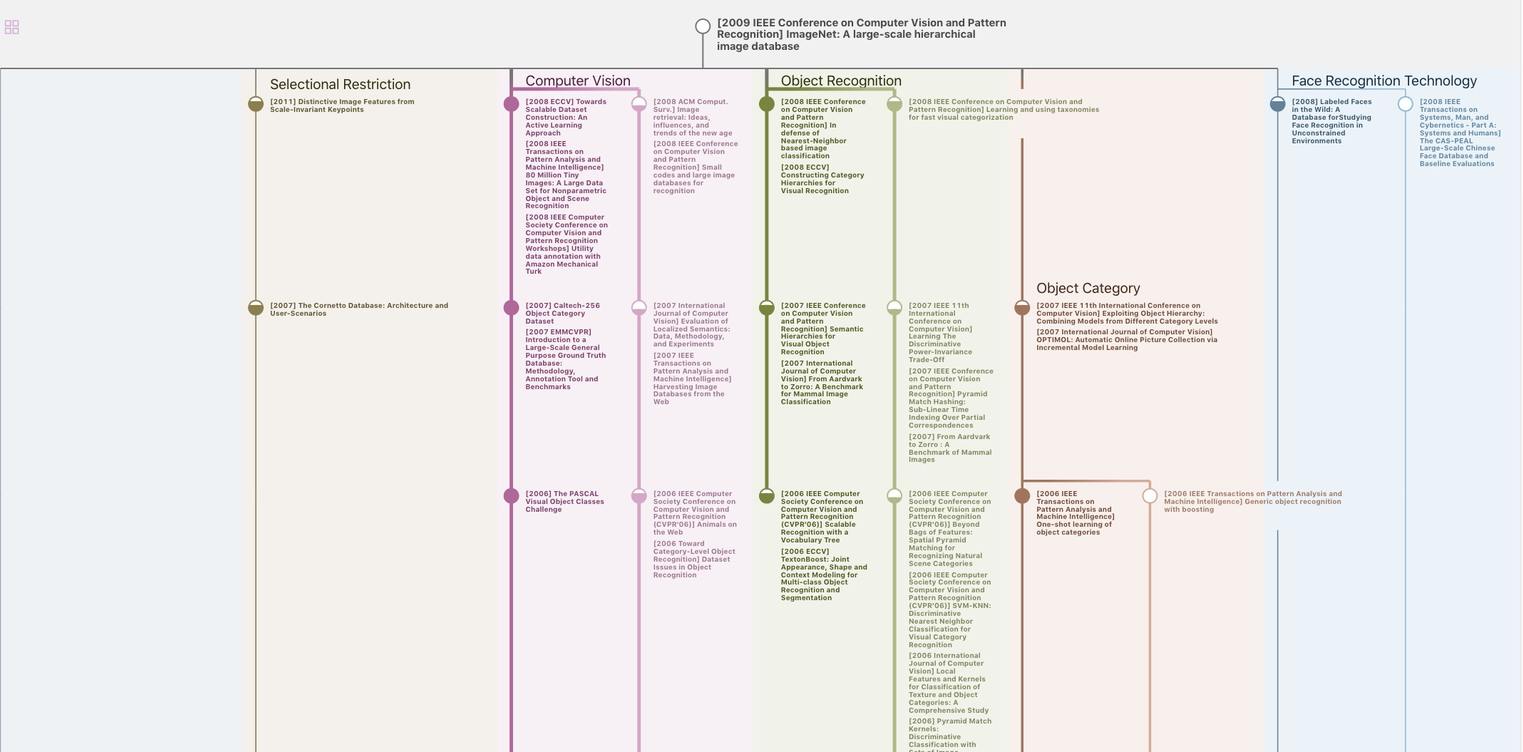
生成溯源树,研究论文发展脉络
Chat Paper
正在生成论文摘要