A multi-branch hand pose estimation network with joint-wise feature extraction and fusion
Signal Processing: Image Communication(2020)
摘要
The study of 3D hand pose estimation from a single depth image is regarded as a detection-based or regression-based problem among most of the existing deep learning-based methods, and this approach does not fully exploit the geometry of the hand, such as its structural and physical constraints. To overcome these weaknesses, we design a network with three simple parallel branches that correspond to the three functional parts of the hand. This observation is motivated by the biological viewpoint that each finger plays a different role in performing grasping and manipulation. In each branch, we perform a more detailed regression in two stages – top-down joint location regression followed by bottom-up hand pose regression – which fully exploits both the local and global structure of a hand. Finally, we further make use of the hand structure and physical constraints to refine each joint by its auxiliary points. The proposed network is a unified structure and function model that is more appropriate for hand pose estimation. Our system does not require pose pre-processing or feedback since it can directly perform training and predicting from end-to-end. The experimental results on three public datasets demonstrate that the proposed system achieves performance comparable to state-of-the-art methods.
更多查看译文
关键词
Hand pose estimation,Neural network,Human–computer interaction,Depth images
AI 理解论文
溯源树
样例
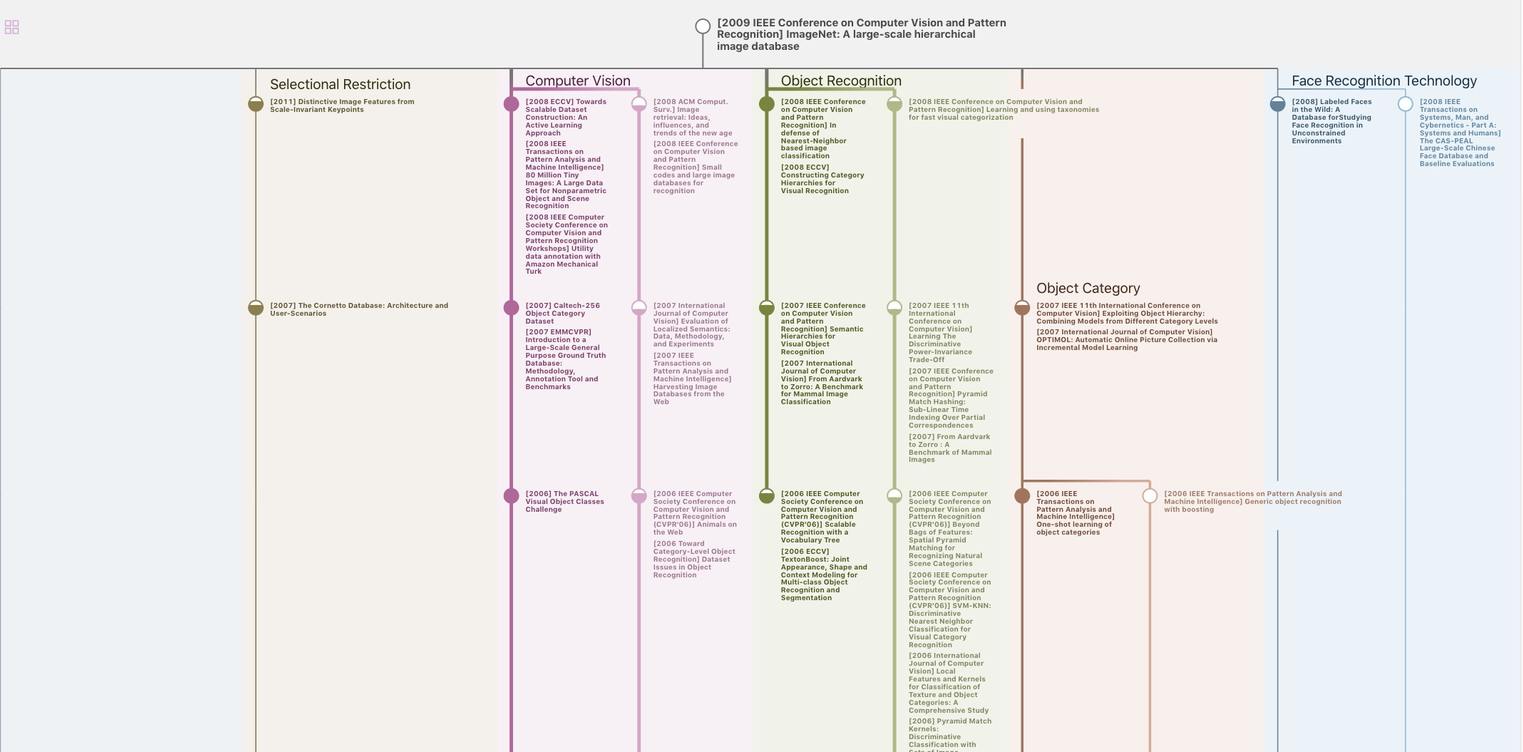
生成溯源树,研究论文发展脉络
Chat Paper
正在生成论文摘要