Dynamical Sampling with Langevin Normalization Flows
ENTROPY(2019)
摘要
In Bayesian machine learning, sampling methods provide the asymptotically unbiased estimation for the inference of the complex probability distributions, where Markov chain Monte Carlo (MCMC) is one of the most popular sampling methods. However, MCMC can lead to high autocorrelation of samples or poor performances in some complex distributions. In this paper, we introduce Langevin diffusions to normalization flows to construct a brand-new dynamical sampling method. We propose the modified Kullback-Leibler divergence as the loss function to train the sampler, which ensures that the samples generated from the proposed method can converge to the target distribution. Since the gradient function of the target distribution is used during the process of calculating the modified Kullback-Leibler, which makes the integral of the modified Kullback-Leibler intractable. We utilize the Monte Carlo estimator to approximate this integral. We also discuss the situation when the target distribution is unnormalized. We illustrate the properties and performances of the proposed method on varieties of complex distributions and real datasets. The experiments indicate that the proposed method not only takes the advantage of the flexibility of neural networks but also utilizes the property of rapid convergence to the target distribution of the dynamics system and demonstrate superior performances competing with dynamics based MCMC samplers.
更多查看译文
关键词
normalization flows,Langevin diffusions,Langevin normalization flows,Monte Carlo sampling
AI 理解论文
溯源树
样例
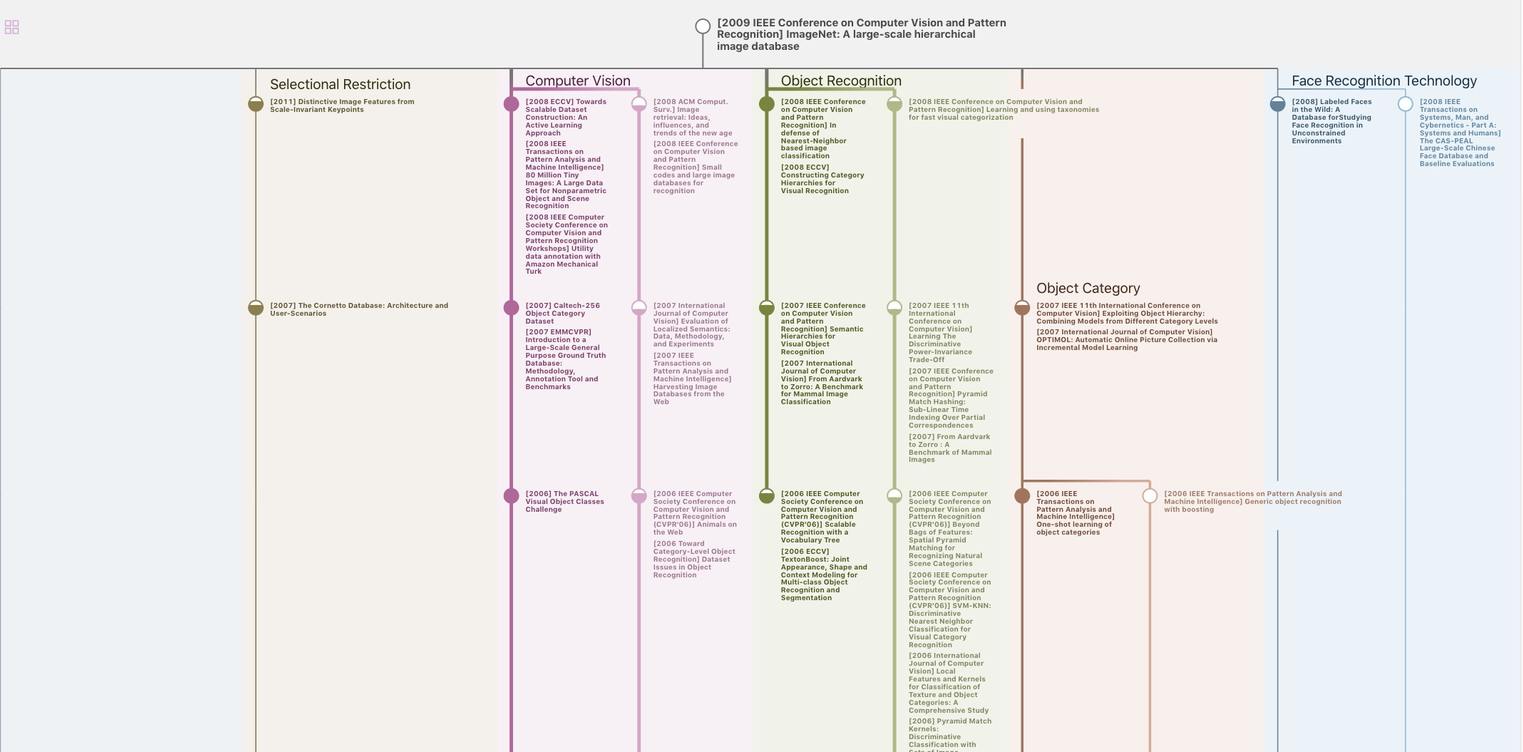
生成溯源树,研究论文发展脉络
Chat Paper
正在生成论文摘要