Accurate image super-resolution using dense connections and dimension reduction network
Multimedia Tools and Applications(2019)
摘要
Recently, the convolution neural network (CNN) has achieved significant performance improvements toward the image Super-Resolution (SR) problems. Yet, the existing benchmark arts exist multiple limitations, including make use of the feature information deficiently, accompany with the gradient disappearance phenomenon and have serious time consumption. The paper utilizes a newly designed fully convolutional neural network named Accurate Image Super-resolution Using Dense Connections and Dimension Reduction Network ( DCDRN ) to fully exploit the image features. Contextual information of image regions utilizes efficiently and accurately through uniting dense connections and cascading small filters multiple times. And such implementation can be regarded as feature extractors to fuse local and global image features. We newly introduce 1 × 1 CNNs parallelization structure in the image reconstruction section to reduce data dimensions of the previous layers, which alleviates the computational burden effectively while avoiding the context info losing. The calculation becomes more complex and the convergence becomes slower during training because of the pre-processed images. The proposed DCDRN invents a simple and effective method which processes the original image directly and the optimization of layers and filters of CNNs shorten the cost of training significantly. Experiments on benchmark datasets with different methods show that DCDRN achieves gratifying performance against state-of-the-art methods. Code is available at https://github.com/doctorwgd/DCDRN .
更多查看译文
关键词
Super-resolution,Dense connections,Parallelization,Contextual information
AI 理解论文
溯源树
样例
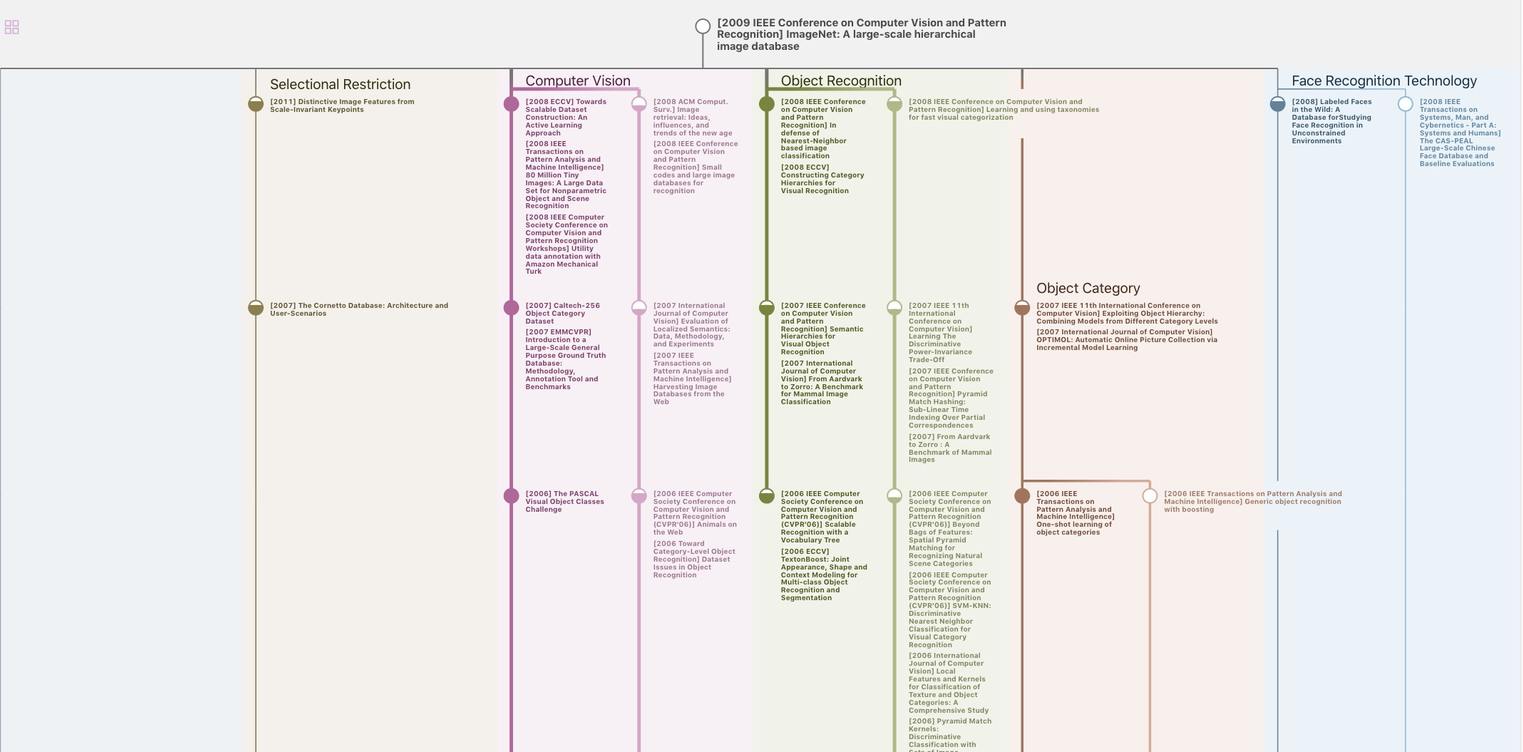
生成溯源树,研究论文发展脉络
Chat Paper
正在生成论文摘要