Sub-Graph Regularization on Kernel Regression for Robust Semi-Supervised Dimensionality Reduction
ENTROPY(2019)
摘要
Dimensionality reduction has always been a major problem for handling huge dimensionality datasets. Due to the utilization of labeled data, supervised dimensionality reduction methods such as Linear Discriminant Analysis tend achieve better classification performance compared with unsupervised methods. However, supervised methods need sufficient labeled data in order to achieve satisfying results. Therefore, semi-supervised learning (SSL) methods can be a practical selection rather than utilizing labeled data. In this paper, we develop a novel SSL method by extending anchor graph regularization (AGR) for dimensionality reduction. In detail, the AGR is an accelerating semi-supervised learning method to propagate the class labels to unlabeled data. However, it cannot handle new incoming samples. We thereby improve AGR by adding kernel regression on the basic objective function of AGR. Therefore, the proposed method can not only estimate the class labels of unlabeled data but also achieve dimensionality reduction. Extensive simulations on several benchmark datasets are conducted, and the simulation results verify the effectiveness for the proposed work.
更多查看译文
关键词
kernel regression,semi-supervised learning,dimensionality reduction,anchor graph regularization
AI 理解论文
溯源树
样例
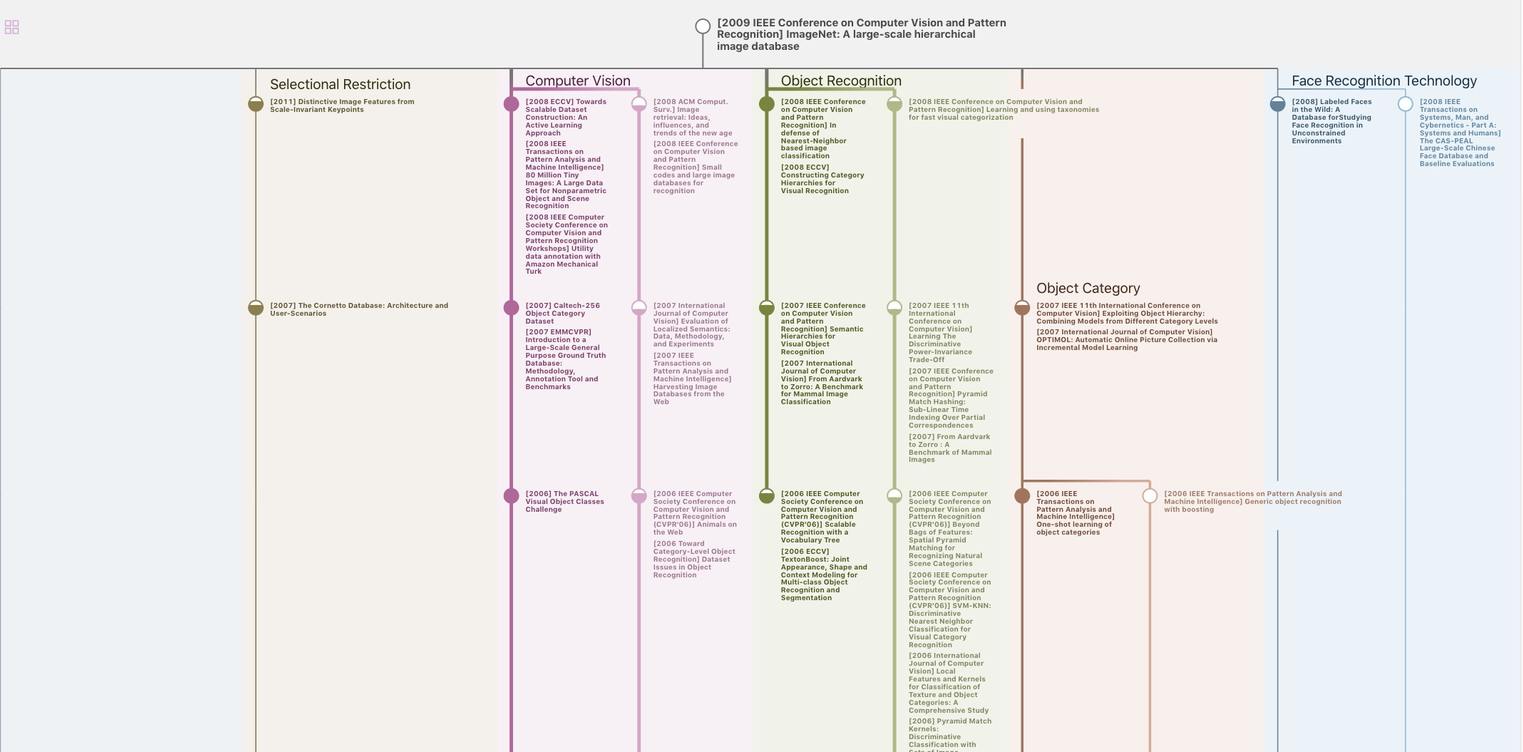
生成溯源树,研究论文发展脉络
Chat Paper
正在生成论文摘要