Sequential image encoding for vision-to-language problems
MULTIMEDIA TOOLS AND APPLICATIONS(2019)
摘要
The combination of visual recognition and language understanding is aim to build a commonly shared space between heterogeneous data of vision and text, such as the tasks of image captioning and visual question answering (VQA). Most existing approaches convert an image into a semantic visual feature vector via deep convolutional neural networks (CNN), while keep the sequential property of text data and represent it with Recurrent Neural Networks(RNN). The key to analyse multi-source heterogeneous data is to construct the inherent correlations between data. In order to reduce the heterogeneous gap among the vision and language, in this work, we represent the image in a sequential way as well as the text. We utilize the objects in the visual scenes and convert the image to a sequence of detected objects and their locations. Then we analogize a sequence of objects(visual language) to a sequence of words(natural language). We take the order of objects into account and evaluate different permutations and combinations of objects. Experimental results on the image captioning and VQA benchmarks demonstrate our hypothesis it’s beneficial to appropriately arrange objects sequence on the Vision-to-Language(V2L) problems.
更多查看译文
关键词
Image captioning,Visual question answering,Object detection
AI 理解论文
溯源树
样例
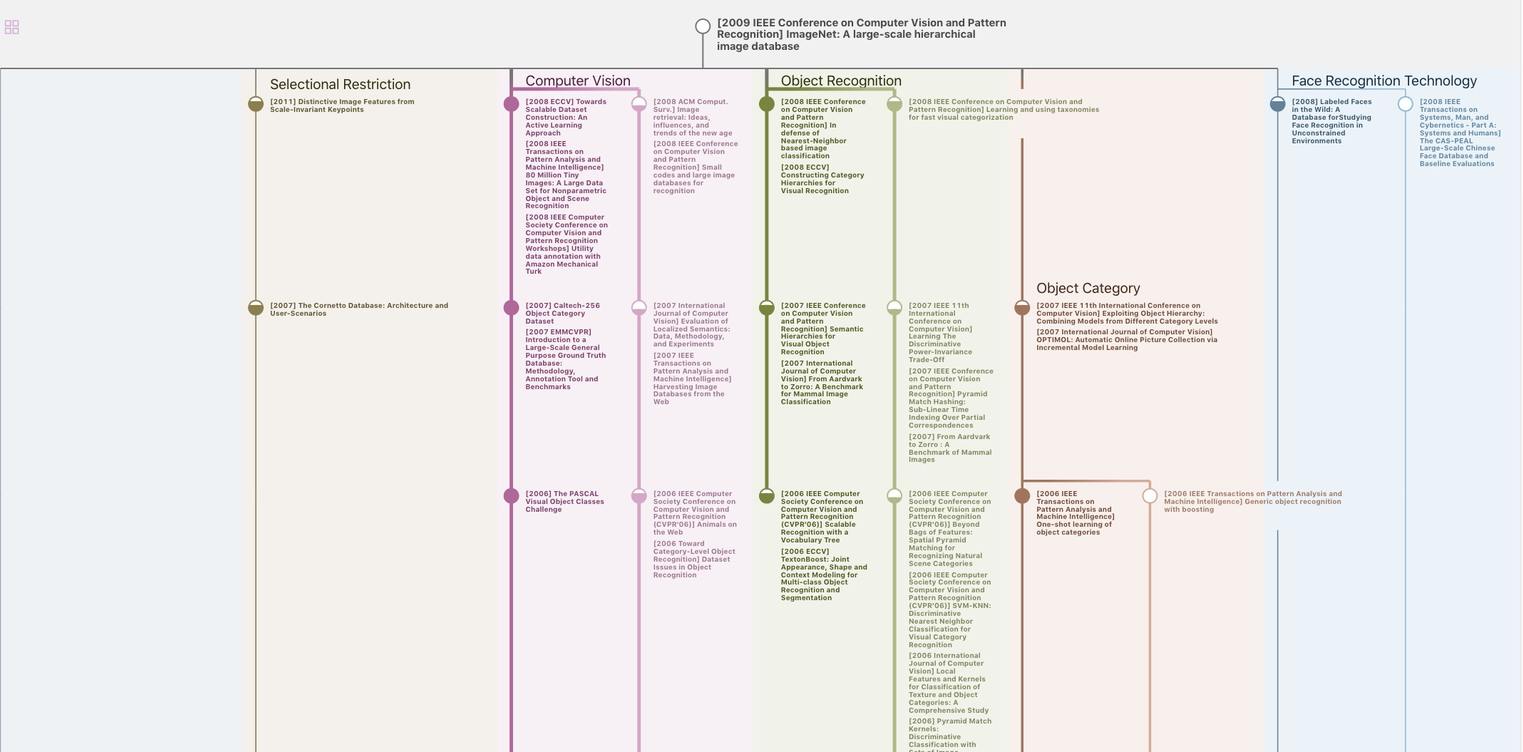
生成溯源树,研究论文发展脉络
Chat Paper
正在生成论文摘要