Memory Network-Based Quality Normalization Of Magnetic Resonance Images For Brain Segmentation
INTELLIGENCE SCIENCE AND BIG DATA ENGINEERING: VISUAL DATA ENGINEERING, PT I(2019)
摘要
Medical images of the same modality but acquired at different centers, with different machines, using different protocols, and by different operators may have highly variable quality. Due to its limited generalization ability, a deep learning model usually cannot achieve the same performance on another database as it has done on the database with which it was trained. In this paper, we use the segmentation of brain magnetic resonance (MR) images as a case study to investigate the possibility of improving the performance of medical image analysis via normalizing the quality of images. Specifically, we propose a memory network (MemNet)-based algorithm to normalize the quality of brain MR images and adopt the widely used 3D U-Net to segment the images before and after quality normalization. We evaluated the proposed algorithm on the benchmark IBSR V2.0 database. Our results suggest that the MemNet-based algorithm can not only normalize and improve the quality of brain MR images, but also enable the same 3D U-Net to produce substantially more accurate segmentation of major brain tissues.
更多查看译文
关键词
Medical image quality normalization, Deep learning, Magnetic resonance image, Brain tissue segmentation
AI 理解论文
溯源树
样例
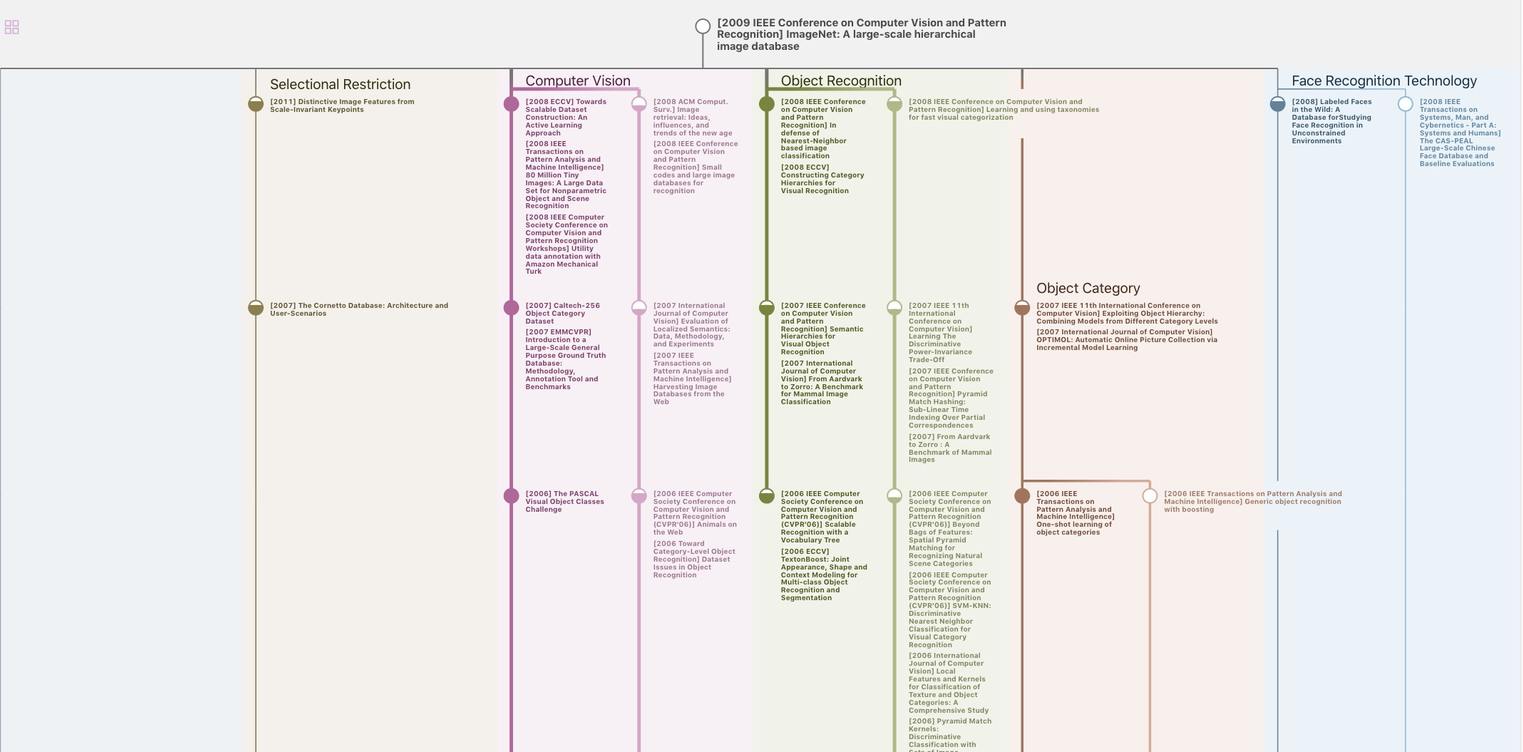
生成溯源树,研究论文发展脉络
Chat Paper
正在生成论文摘要