Multi-Modal Deep Learning for Vehicle Sensor Data Abstraction and Attack Detection
2019 IEEE International Conference on Vehicular Electronics and Safety (ICVES)(2019)
摘要
Mulimodal learning is the process of reasoning with different types of data simultaneously using a neural network. This is no easy feat. Challenges to tackle include mapping features from one modality to another and finding a joint representation. As applied to connected vehicles, we propose a multimodal module that detects manipulations in the image sensor through verifying its consistency with the LiDAR sensor.A state-of-the-art multimodal network, Regnet, is adapted and trained for attack detection. Adaptation includes replacing the input layer and abstracting the multimodal input process. The KITTI dataset is extended to represent two attacks on connected vehicles: inpainting and translation. The end product is a smart multimodal module that abstracts connected-vehicle sensor input and guards against data integrity breaches.
更多查看译文
关键词
mobile robots,multisensor systems,data security,neural networks
AI 理解论文
溯源树
样例
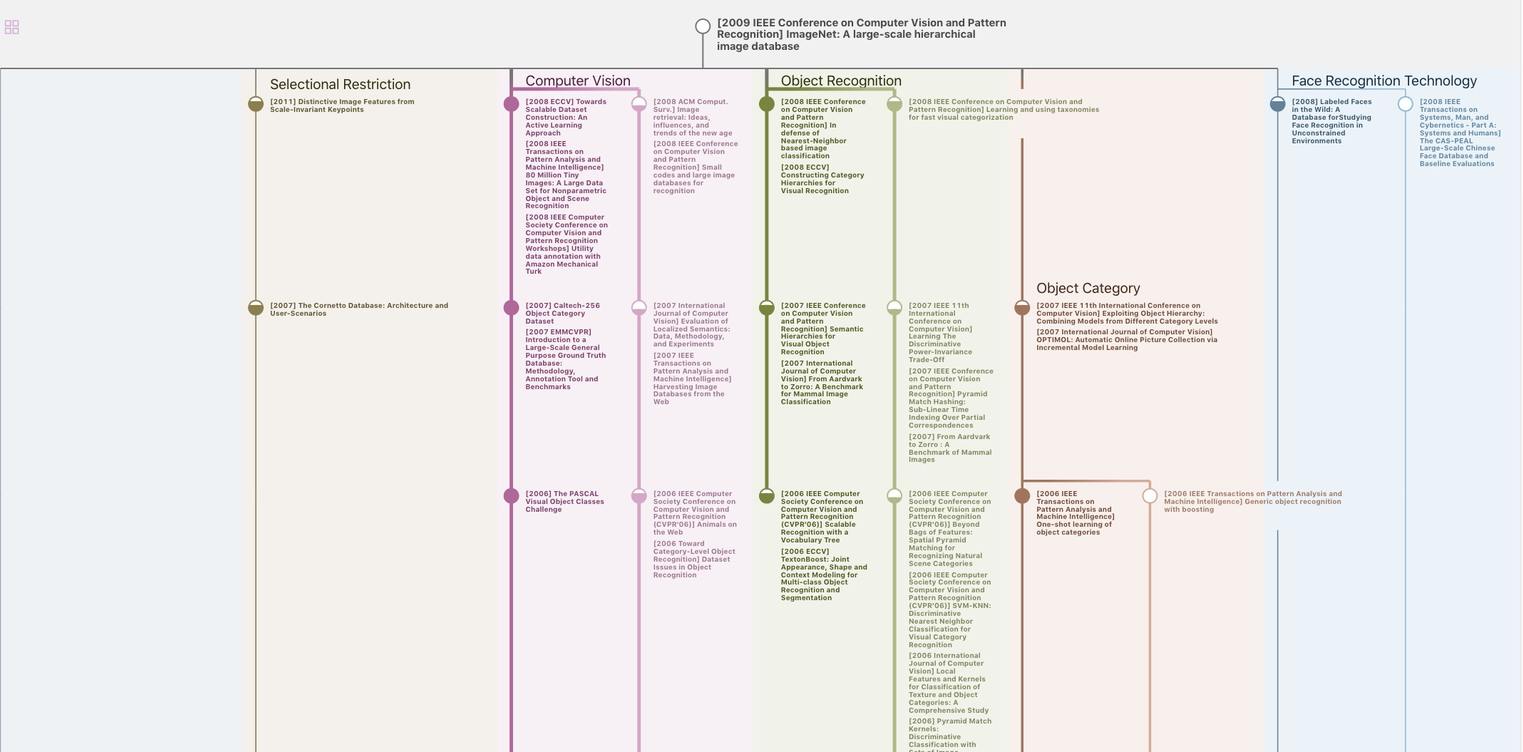
生成溯源树,研究论文发展脉络
Chat Paper
正在生成论文摘要