Tree Species Classification Based on Hybrid Ensembles of a Convolutional Neural Network (CNN) and Random Forest Classifiers
REMOTE SENSING(2019)
摘要
In this paper, we evaluate different popular voting strategies for fusion of classifier results. A convolutional neural network (CNN) and different variants of random forest (RF) classifiers were trained to discriminate between 15 tree species based on airborne hyperspectral imaging data. The spectral data was preprocessed with a multi-class linear discriminant analysis (MCLDA) as a means to reduce dimensionality and to obtain spatial-spectral features. The best individual classifier was a CNN with a classification accuracy of 0.73 +/- 0.086. The classification performance increased to an accuracy of 0.78 +/- 0.053 by using precision weighted voting for a hybrid ensemble of the CNN and two RF classifiers. This voting strategy clearly outperformed majority voting (0.74), accuracy weighted voting (0.75), and presidential voting (0.75).
更多查看译文
关键词
hyperspectral imaging,tree species,multiple classifier fusion,convolutional neural network,random forest,rotation forest
AI 理解论文
溯源树
样例
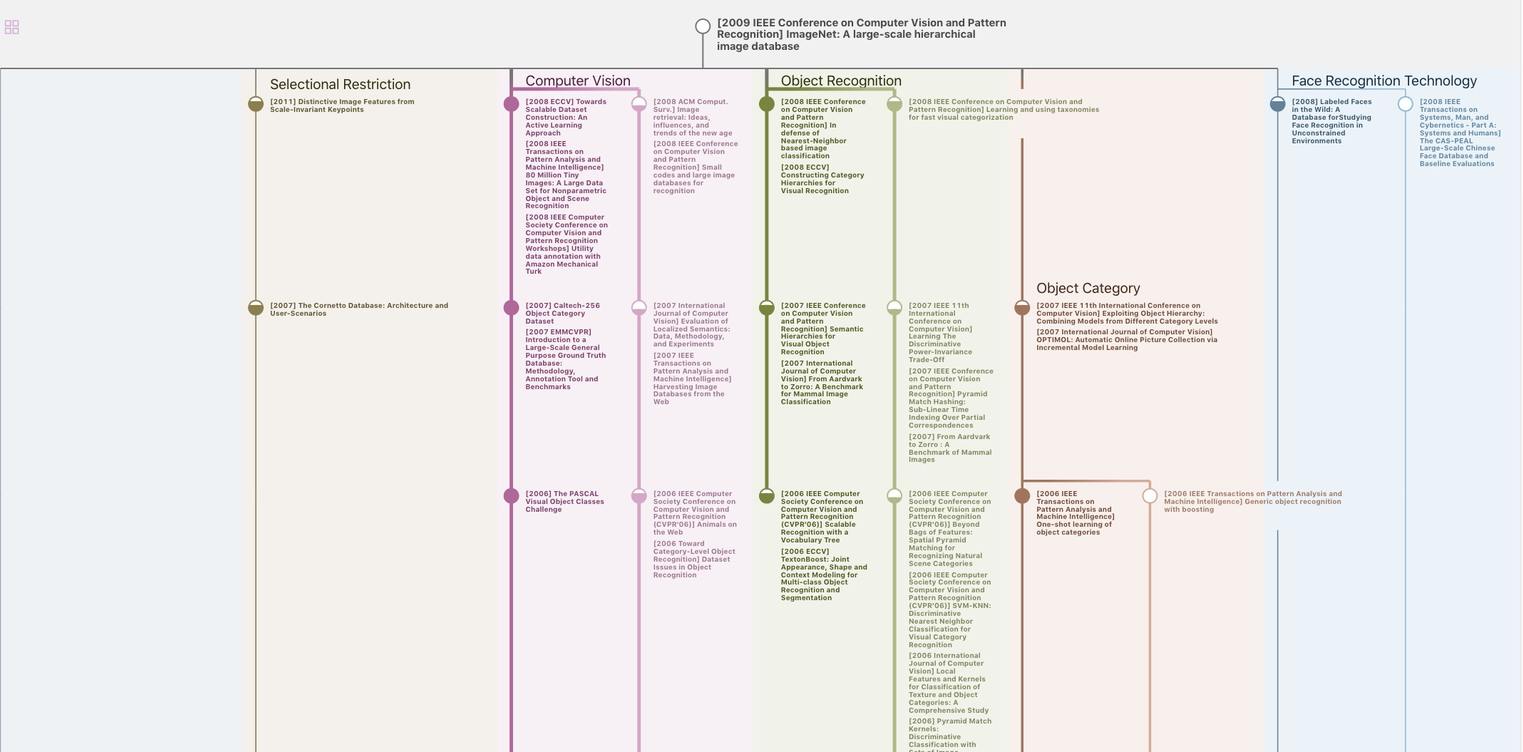
生成溯源树,研究论文发展脉络
Chat Paper
正在生成论文摘要