Urban air temperature model using GOES-16 LST and a diurnal regressive neural network algorithm
Remote Sensing of Environment(2020)
摘要
An urban air temperature model is presented using the enterprise GOES-16 land surface temperature product. The model is constructed by fitting the difference between ground-truth air temperature data against satellite LST using a Gaussian function. A time-match algorithm aligns the ground and satellite measurements within 5-min of one another, and the resulting matched values are compared over ten months to investigate their correlation. Land cover, latitude, longitude, local time, and elevation are input to a regressive neural network to fit each unique GOES-16 pixel according to ground-based properties. Over 150 ground stations and satellite pixels throughout the continental U.S. are used near urban areas to construct the diurnal Gaussian relationship and approximate air temperature. Statistics from a five month validation period generates an RMSE of 2.6 K, a bias of 0.8 K, and R2 of 0.86, which are in strong competition with other studies at lower resolution, less geographic integration, and less temporal resolvability. The algorithm also produced strong spatial correlations with a high resolution numerical model, resulting in a mean RMSE value of 2.1 K for nearly 7000 pixels. The overall presentation of this model aims to simplify the calculation of air temperature from satellite LST and create a successful model that performs well in heterogeneous environments. The improvement of urban air temperature calculations will also result in improved satellite land surface products such as relative humidity and heat index.
更多查看译文
关键词
Air temperature,GOES-16,Neural network,Regression,LST,Air temperature model,Satellite remote sensing
AI 理解论文
溯源树
样例
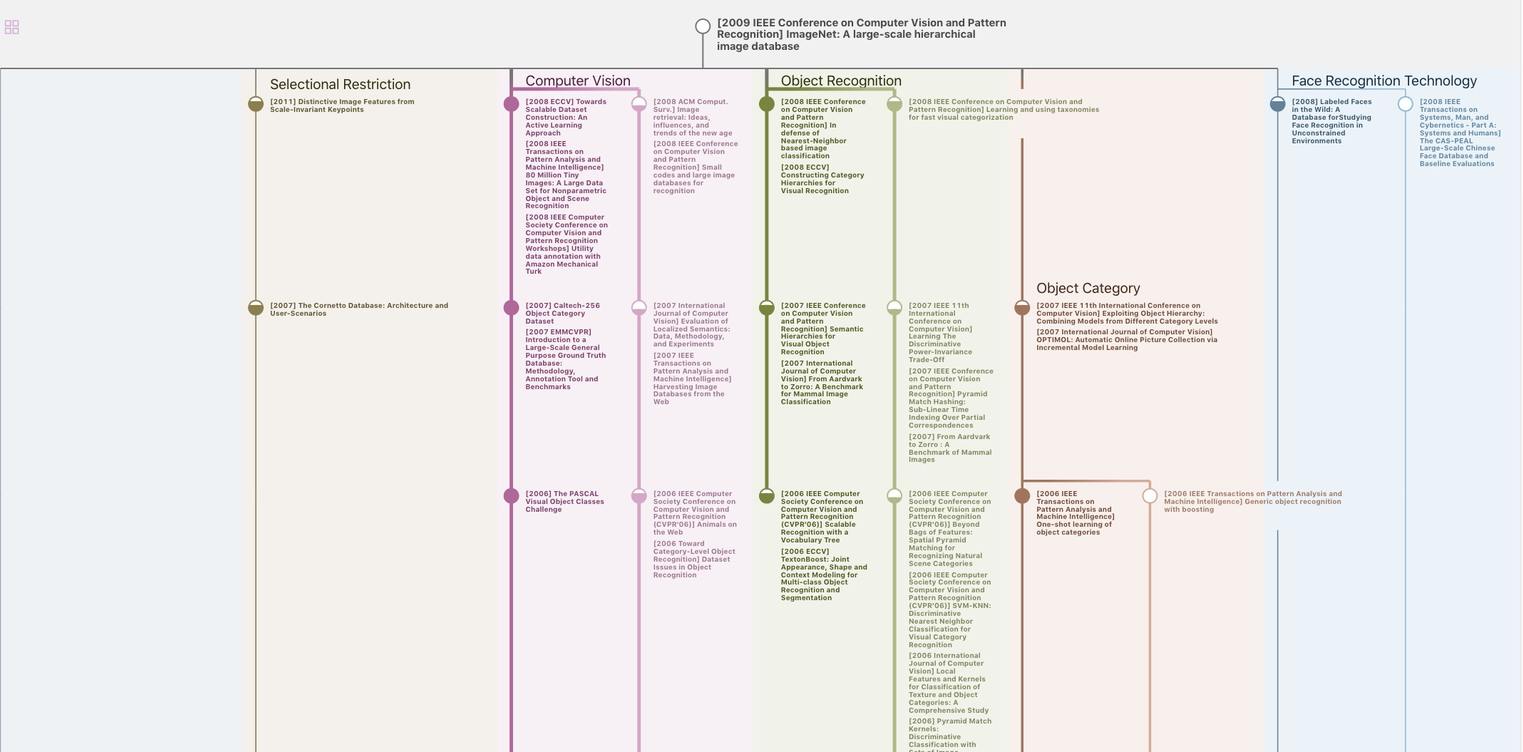
生成溯源树,研究论文发展脉络
Chat Paper
正在生成论文摘要