Probabilistic Deep Ordinal Regression Based On Gaussian Processes
2019 IEEE/CVF INTERNATIONAL CONFERENCE ON COMPUTER VISION (ICCV 2019)(2019)
Abstract
With excellent representation power for complex data, deep neural networks (DNNs) based approaches are state-of-the-art for ordinal regression problem which aims to classify instances into ordinal categories. However, DNNs are not able to capture uncertainties and produce probabilistic interpretations. As a probabilistic model, Gaussian Processes (GPs) on the other hand offers uncertainty information, which is nonetheless lack of scalability for large datasets. This paper adapts traditional GPs for ordinal regression problem by using both conjugate and non-conjugate ordinal likelihood. Based on that, it proposes a deep neural network with a GPs layer on the top, which is trained end-to-end by the stochastic gradient descent method for both neural network parameters and GPs parameters. The parameters in the ordinal likelihood function are learned as neural network parameters so that the proposed framework is able to produce fitted likelihood functions for training sets and make probabilistic predictions for test points. Experimental results on three real-world benchmarks - image aesthetics rating, historical image grading and age group estimation - demonstrate that in terms of mean absolute error, the proposed approach outperforms state-of-the-art ordinal regression approaches and provides the confidence for predictions.
MoreTranslated text
Key words
probabilistic deep ordinal regression,Gaussian processes,deep neural networks based approaches,DNNs,ordinal regression problem,ordinal categories,probabilistic interpretations,probabilistic model,traditional GPs regression,deep neural network,GPs layer,stochastic gradient descent method,neural network parameters,ordinal likelihood function,probabilistic predictions,ordinal regression approach
AI Read Science
Must-Reading Tree
Example
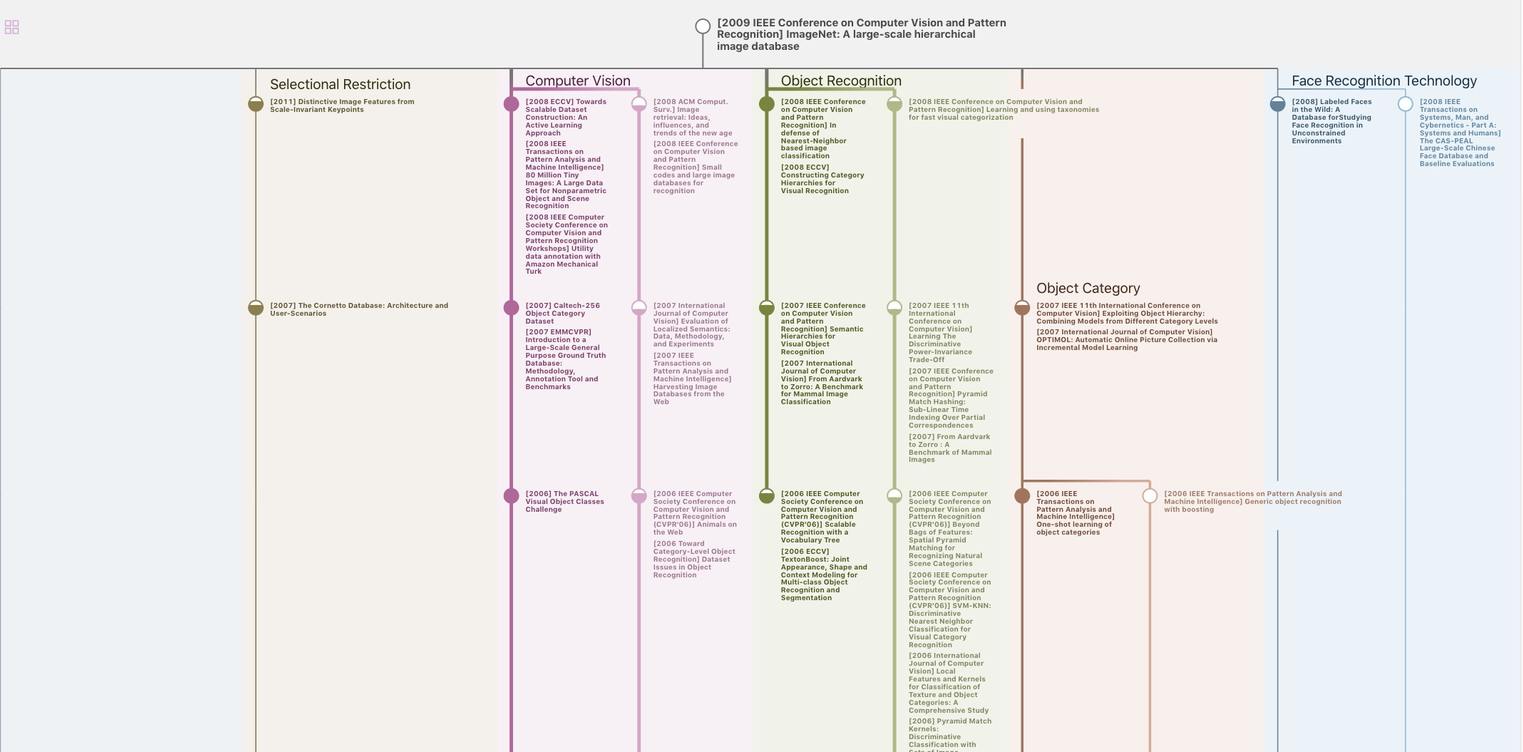
Generate MRT to find the research sequence of this paper
Chat Paper
Summary is being generated by the instructions you defined