Medical rolling bearing fault prognostics based on improved extreme learning machine
JOURNAL OF COMBINATORIAL OPTIMIZATION(2019)
摘要
The problem studied in this article:the random selection of the input weight and the implicit layer bias of the extreme learning machine leads to the instability of the medical rolling bearing fault prediction result of the algorithm. It requires more hidden layer nodes to ensure the accuracy of the algorithm, duing to this, Ensemble Error Minimized Extreme Learning Machine (EEM-ELM) is proposed. The EEM-ELM uses various error limit learning machines (EM-ELM) trained on different training sets as member classifiers. Member classifier factors are also used, including predictive entropy, which verifies the set’s accuracy and average and output weights as weights. All of these form a composite classifier by weighted linear combination. This method skillfully solves the problem of optimal hidden layer neurons number selection. The normalized energy and permutation entropy of each IMF component obtained by VMD decomposition fault signal are established as feature vectors, and the improved EEM-ELM algorithm is used as the fault diagnosis model for bearing fault classification algorithm. The fault diagnosis model is applied to the classification of bearing fault signals. The analysis of experimental data proves that the classification result of the proposed EEM-ELM algorithm is better than the ELM algorithm. At the same time, the accuracy rate is higher than each member classifier because of proper weighting processing. Apart from this, since the EEM-ELM algorithm integrates the error minimization limit learning machine, the EEM-ELM algorithm does not need to select the optimal hidden layer node number. The EEM-ELM algorithm only needs to specify the maximum number of training set samples that each EM-ELM-based classifier can tolerate misclassification to achieve high stability and high accuracy classification.
更多查看译文
关键词
Medical rolling bearing, Fault prognostics, Ensemble error minimized extreme learning machine, VMD, Accuracy rate
AI 理解论文
溯源树
样例
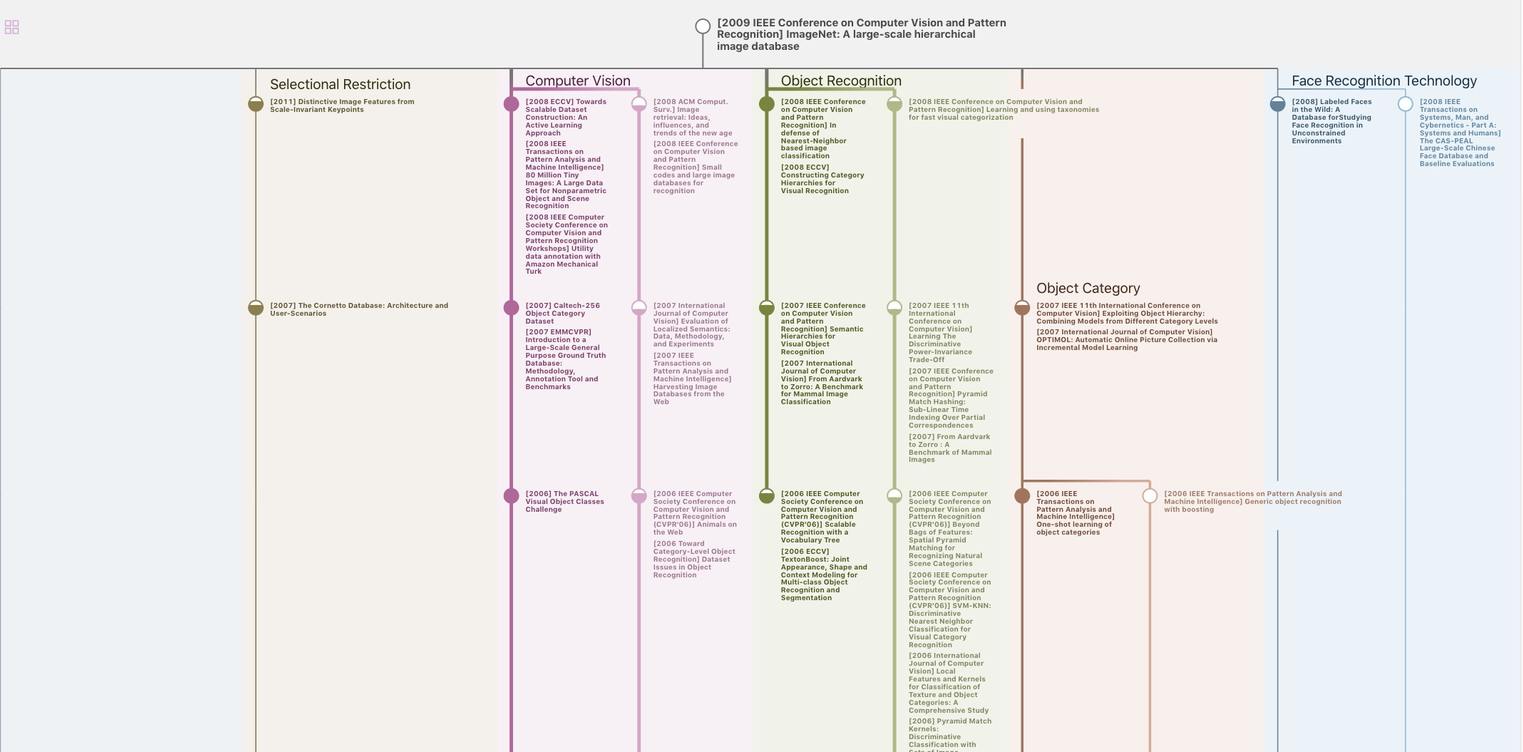
生成溯源树,研究论文发展脉络
Chat Paper
正在生成论文摘要