A Denoising SVR-MLP Method for Remaining Useful Life Prediction of Lithium-ion Battery
IEEE Energy Conversion Congress and Exposition(2019)
摘要
Remaining useful life (RUL) prognostic is critical for the safe and comfortable driving experience of Electric vehicles. A data-driven prediction model of low complexity and high accuracy is indispensable for on-broad and online application. In this paper, the combination of two computational efficient models, namely SVR and MLP, are studied for prediction accuracy elevation. By analyzing the prediction error distribution of the two models, an optimization objective function of the fusion model is designed to minimize the prediction noise. And an analytical form of the fusion protocol is derived when solving the optimization problem. The accuracy of the full life cycle is enhanced and training data size remains the same. NASA lithium-ion battery dataset is used to verify the superiority of the proposed method.
更多查看译文
关键词
Lithium-ion battery,Remaining useful life,Support Vector Regression,Multi Layer Perceptron I
AI 理解论文
溯源树
样例
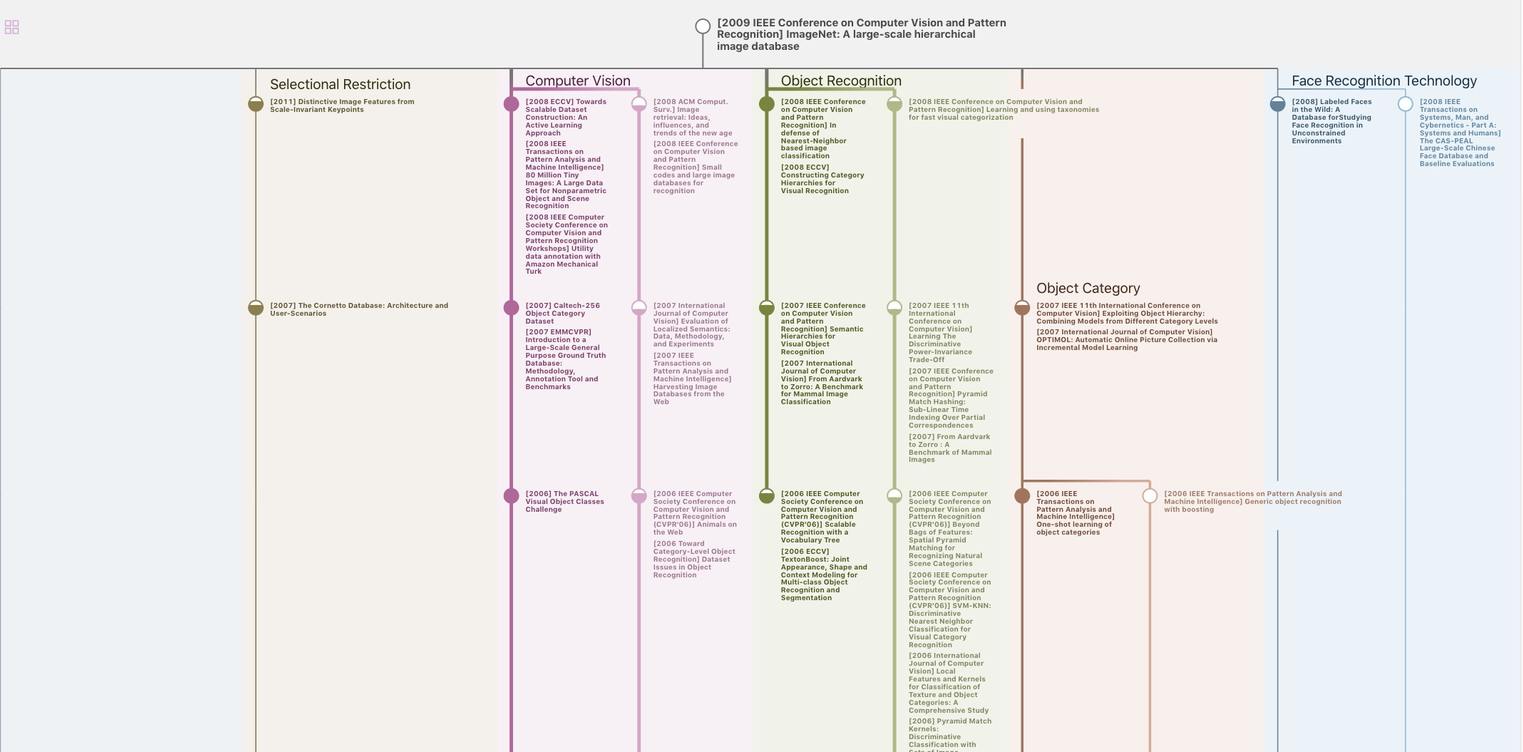
生成溯源树,研究论文发展脉络
Chat Paper
正在生成论文摘要