Towards large-scale mapping of local climate zones using multitemporal Sentinel 2 data and convolutional neural networks
Remote Sensing of Environment(2020)
摘要
In recent years, the concept of Local Climate Zones (LCZs) has become a new standard in the research of urban landscapes. LCZs outline a classification scheme, which is designed to categorize urban and rural surfaces according to their climate-relevant properties, irrespective of local building materials or cultural background. We present a novel workflow for a high-resolution derivation of LCZs using multi-temporal Sentinel 2 (S2) composites and supervised Convolutional Neural Networks (CNNs). We assume that CNNs, due to their potential invariance to size and illumination of objects, are best suited to predict the highly context-based LCZs on a large scale. As a first step, the proposed workflow includes a fully automated generation of cloud-free S2 composites. These composites serve as training data basis for the LCZ classifications carried out over eight German cities. Results show that by using a CNN, overall accuracies can be increased by an average of 16.5 and 4.8 percentage points when compared to a pixel-based and a texture-based Random Forest approach, respectively. If sufficient training data is available, CNN models proved to be robust in classifying unknown cities and achieved overall accuracies of up to 86.5%. The proposed method constitutes a feasible approach for automated, large scale mapping of LCZs, and could be the preferred alternative for LCZ classifications in upcoming studies.
更多查看译文
关键词
Local climate zones,Convolutional neural network,Sentinel 2
AI 理解论文
溯源树
样例
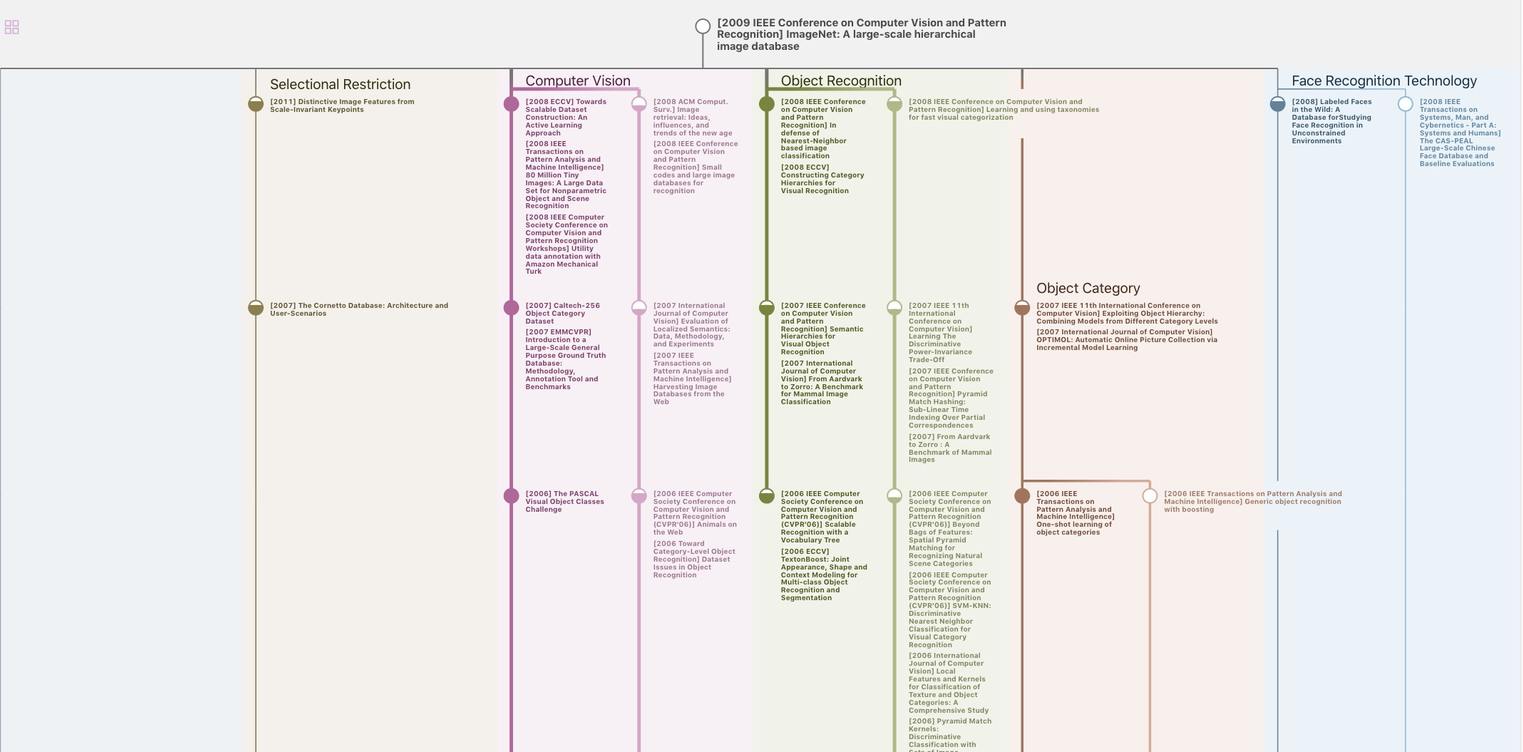
生成溯源树,研究论文发展脉络
Chat Paper
正在生成论文摘要