Problem-driven scenario generation: an analytical approach for stochastic programs with tail risk measure
MATHEMATICAL PROGRAMMING(2019)
摘要
Scenario generation is the construction of a discrete random vector to represent parameters of uncertain values in a stochastic program. Most approaches to scenario generation are distribution-driven , that is, they attempt to construct a random vector which captures well in a probabilistic sense the uncertainty. On the other hand, a problem-driven approach may be able to exploit the structure of a problem to provide a more concise representation of the uncertainty. In this paper we propose an analytic approach to problem-driven scenario generation. This approach applies to stochastic programs where a tail risk measure, such as conditional value-at-risk, is applied to a loss function. Since tail risk measures only depend on the upper tail of a distribution, standard methods of scenario generation, which typically spread their scenarios evenly across the support of the random vector, struggle to adequately represent tail risk. Our scenario generation approach works by targeting the construction of scenarios in areas of the distribution corresponding to the tails of the loss distributions. We provide conditions under which our approach is consistent with sampling, and as proof-of-concept demonstrate how our approach could be applied to two classes of problem, namely network design and portfolio selection. Numerical tests on the portfolio selection problem demonstrate that our approach yields better and more stable solutions compared to standard Monte Carlo sampling.
更多查看译文
关键词
90C15 (stochastic programming)
AI 理解论文
溯源树
样例
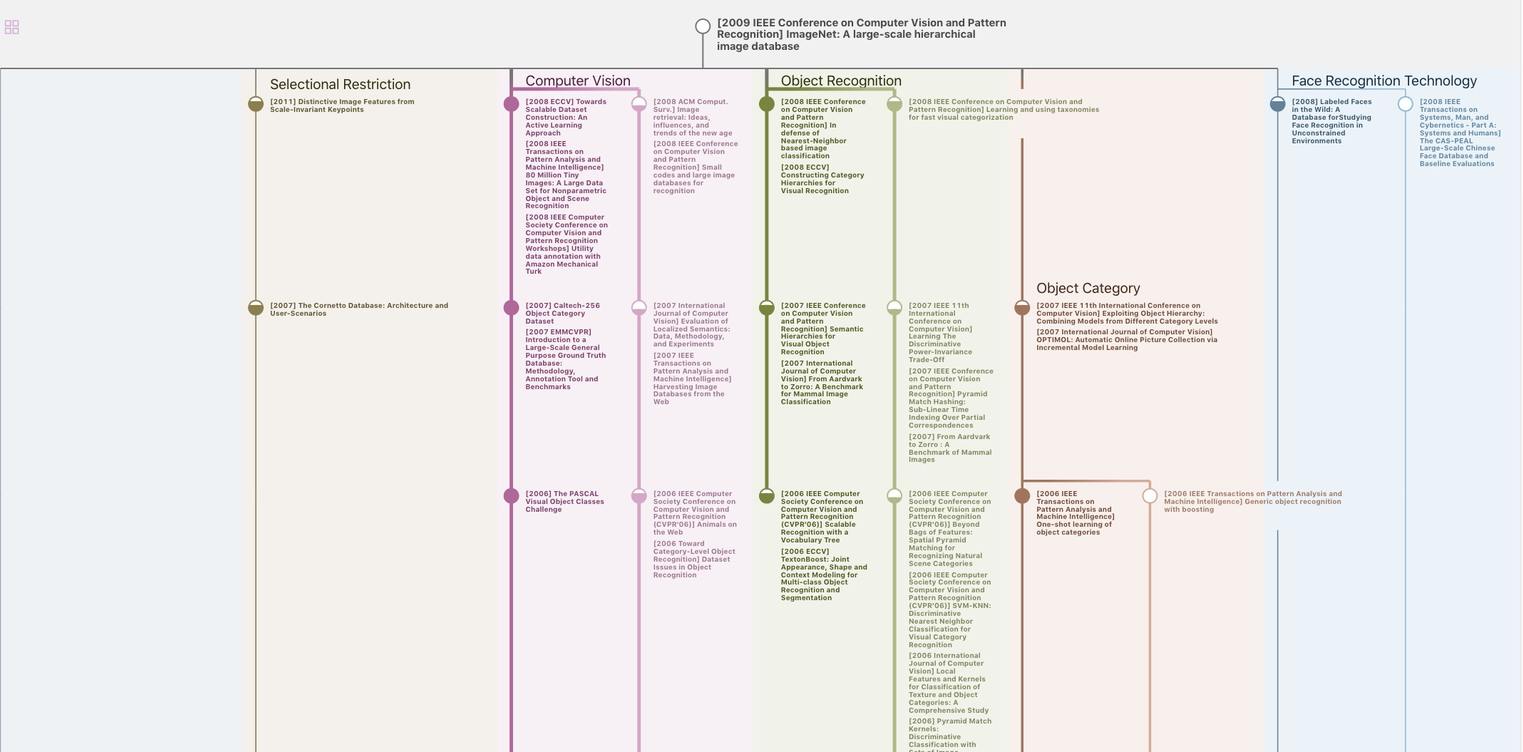
生成溯源树,研究论文发展脉络
Chat Paper
正在生成论文摘要