Circular Regression Trees and Forests with an Application to Probabilistic Wind Direction Forecasting
JOURNAL OF THE ROYAL STATISTICAL SOCIETY SERIES C-APPLIED STATISTICS(2020)
摘要
Although circular data occur in a wide range of scientific fields, the methodology for distributional modelling and probabilistic forecasting of circular response variables is quite limited. Most of the existing methods are built on generalized linear and additive models, which are often challenging to optimize and interpret. Specifically, capturing abrupt changes or interactions is not straightforward but often relevant, e.g. for modelling wind directions subject to different wind regimes. Additionally, automatic covariate selection is desirable when many predictor variables are available, as is often the case in weather forecasting. To address these challenges we suggest a general distributional approach using regression trees and random forests to obtain probabilistic forecasts for circular responses. Using trees simplifies model estimation as covariates are used only for partitioning the data and subsequently just a simple von Mises distribution is fitted in the resulting subgroups. Circular regression trees are straightforward to interpret, can capture non-linear effects and interactions, and automatically select covariates affecting location and/or scale in the von Mises distribution. Circular random forests regularize and smooth the effects from an ensemble of trees. The new methods are applied to probabilistic wind direction forecasting at two Austrian airports, considering other common approaches as a benchmark.
更多查看译文
关键词
Circular data,Distributional regression,Probabilistic forecasting,Random forests,Regression trees,von Mises distribution
AI 理解论文
溯源树
样例
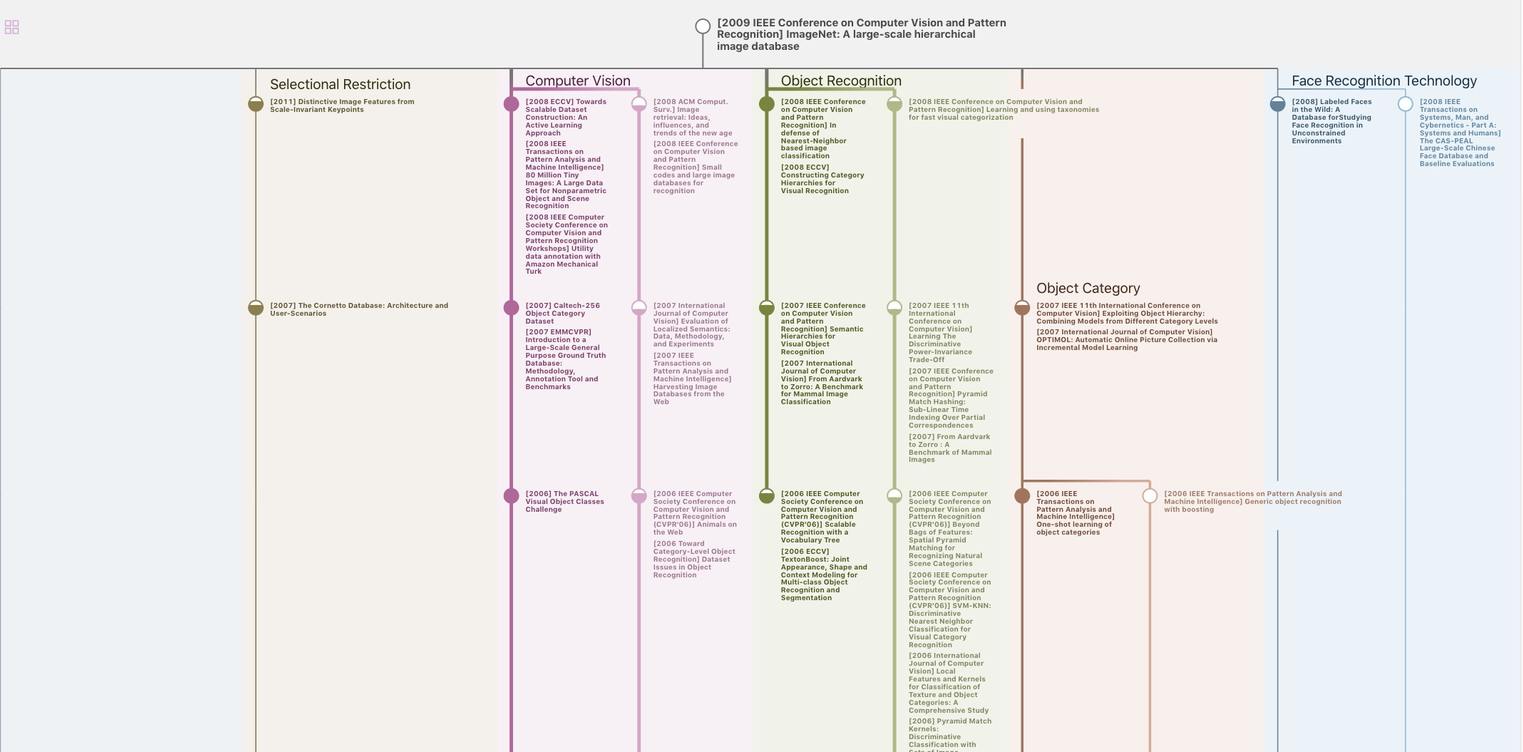
生成溯源树,研究论文发展脉络
Chat Paper
正在生成论文摘要