Privacynet: Semi-Adversarial Networks For Multi-Attribute Face Privacy
IEEE TRANSACTIONS ON IMAGE PROCESSING(2020)
摘要
Recent research has established the possibility of deducing soft-biometric attributes such as age, gender, and race from an individual's face image with high accuracy. However, this raises privacy concerns, especially when face images collected for biometric recognition purposes are used for attribute analysis without the person's consent. To address this problem, we develop a technique for imparting soft biometric privacy to face images via an image perturbation methodology. The image perturbation is undertaken using a GAN-based Semi-Adversarial Network (SAN) - referred to as PrivacyNet - that modifies an input face image such that it can be used by a face matcher for matching purposes but cannot be reliably used by an attribute classifier. Further, PrivacyNet allows a person to choose specific attributes that have to be obfuscated in the input face images (e.g., age and race), while allowing for other types of attributes to be extracted (e.g., gender). Extensive experiments using multiple face matchers, multiple age/gender/race classifiers, and multiple face datasets demonstrate the generalizability of the proposed multi-attribute privacy enhancing method across multiple face and attribute classifiers.
更多查看译文
关键词
Faces, Privacy, Face recognition, Biomedical imaging, Perturbation methods, Biological system modeling, Image recognition, Privacy, semi-adversarial, neural networks, autoencoder, face image, perturbation, soft biometrics, deep learning
AI 理解论文
溯源树
样例
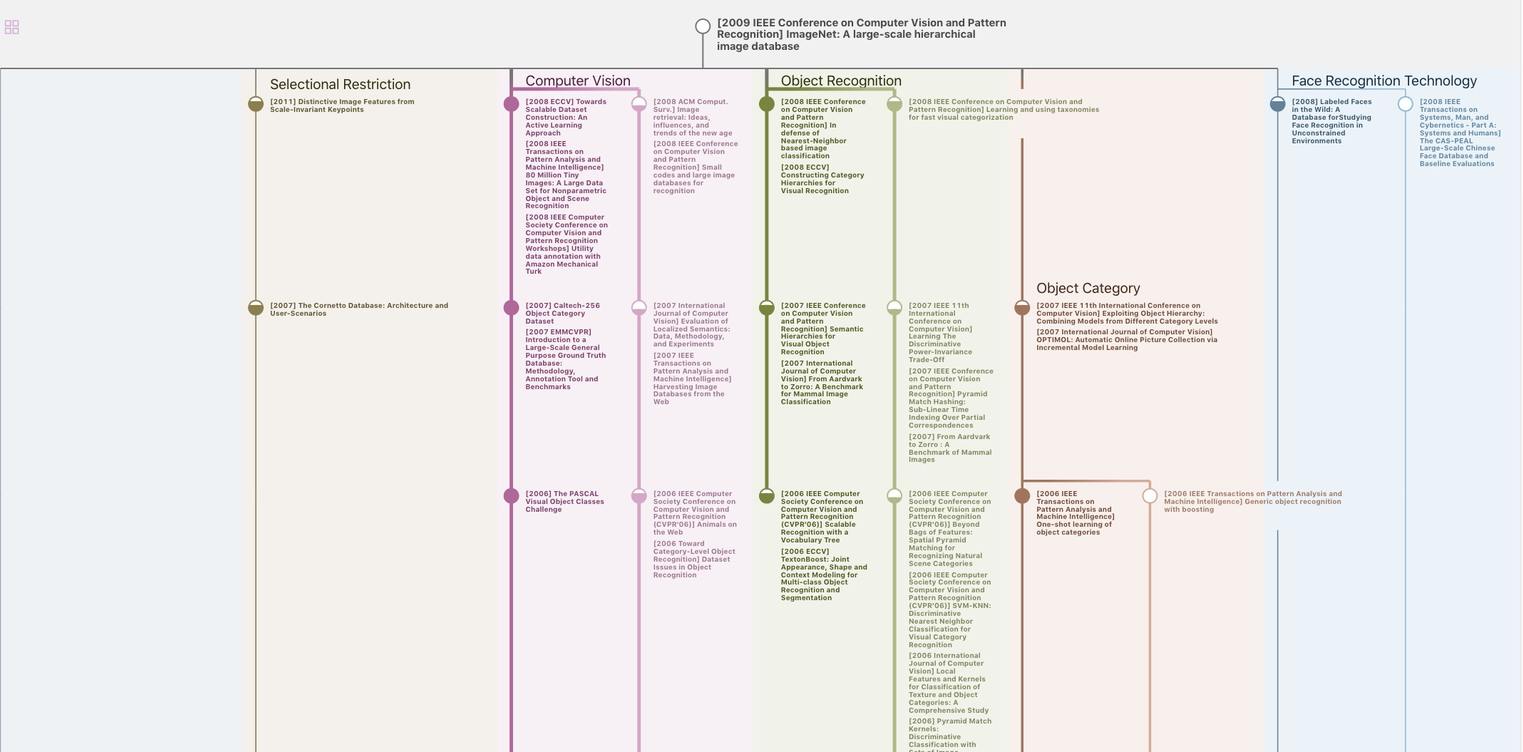
生成溯源树,研究论文发展脉络
Chat Paper
正在生成论文摘要