Online Robust Principal Component Analysis With Change Point Detection
IEEE Transactions on Multimedia(2020)
摘要
Robust principal component analysis (PCA) is a key technique for dynamical high-dimensional data analysis, including background subtraction for surveillance video. Typically, robust PCA requires all observations to be stored in memory before processing. The batch manner makes robust PCA inefficient for big data. In this paper, we develop an efficient online robust PCA method, namely, online moving window robust principal component analysis (OMWRPCA). Unlike the existing algorithms, OMWRPCA can successfully track not only slowly changing subspaces but also abruptly changing subspaces. Embedding hypothesis testing into the algorithm enables OMWRPCA to detect change points of the underlying subspaces. Extensive numerical experiments, including real-time background subtraction, demonstrate the superior performance of OMWRPCA compared with other state-of-the-art approaches.
更多查看译文
关键词
Principal component analysis,Sparse matrices,Matrix decomposition,Microsoft Windows,Surveillance,Big Data,Synthetic aperture sonar
AI 理解论文
溯源树
样例
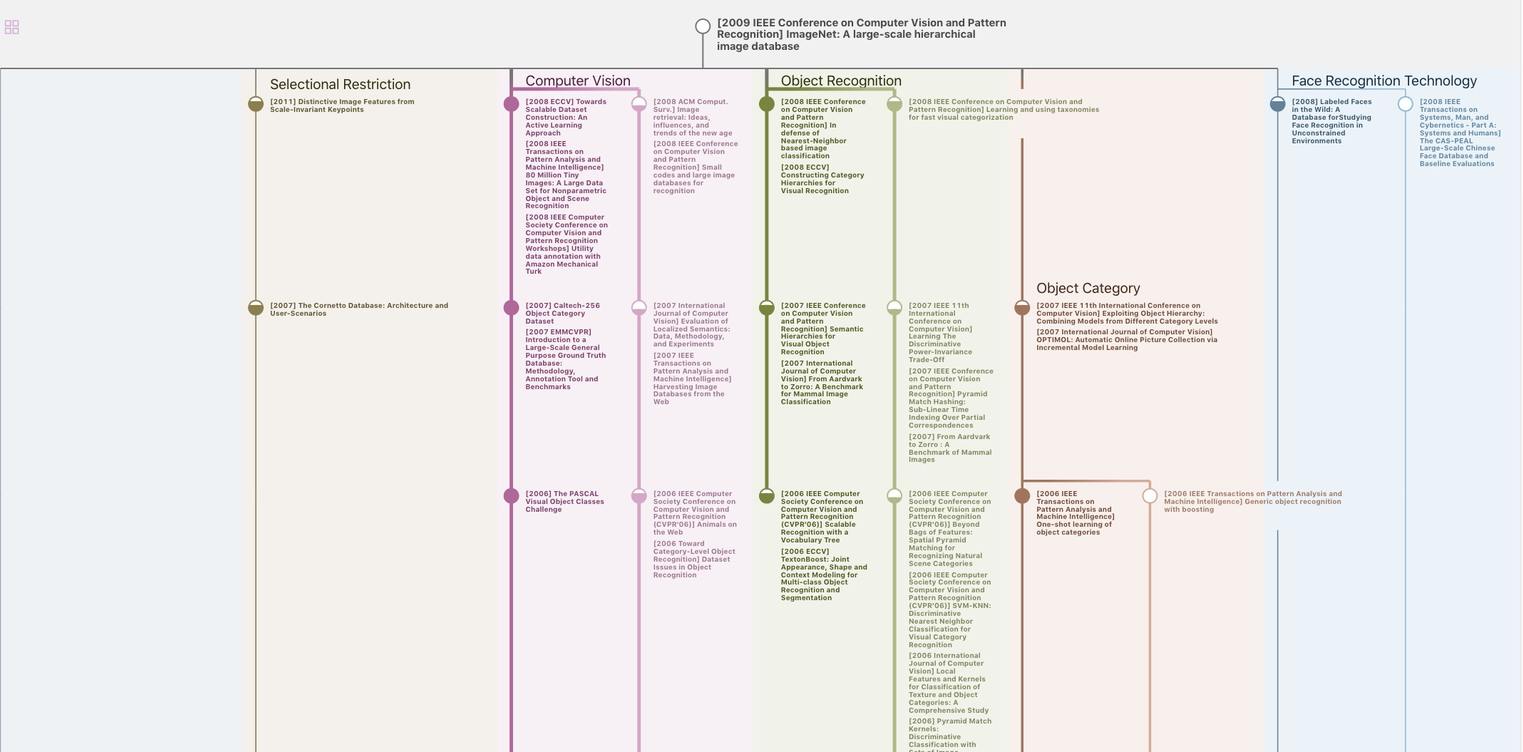
生成溯源树,研究论文发展脉络
Chat Paper
正在生成论文摘要