Statistical Detection of Collective Data Fraud
arxiv(2020)
摘要
Statistical divergence is widely applied in multimedia processing, basically due to regularity and explainable features displayed in data. However, in a broader range of data realm, these advantages may not out-stand, and therefore a more general approach is required. In data detection, statistical divergence can be used as an similarity measurement based on collective features. In this paper, we present a collective detection technique based on statistical divergence. The technique extracts distribution similarities among data collections, and then uses the statistical divergence to detect collective anomalies. Our technique continuously evaluates metrics as evolving features and calculates adaptive threshold to meet the best mathematical expectation. To illustrate details of the technique and explore its efficiency, we case-studied a real world problem of click farming detection against malicious online sellers. The evaluation shows that these techniques provided efficient classifiers. They were also sufficiently sensitive to a much smaller magnitude of data alteration, compared with real world malicious behaviours. Thus, it is applicable in the real world.
更多查看译文
关键词
Statistical divergence, collective, fraud, detection
AI 理解论文
溯源树
样例
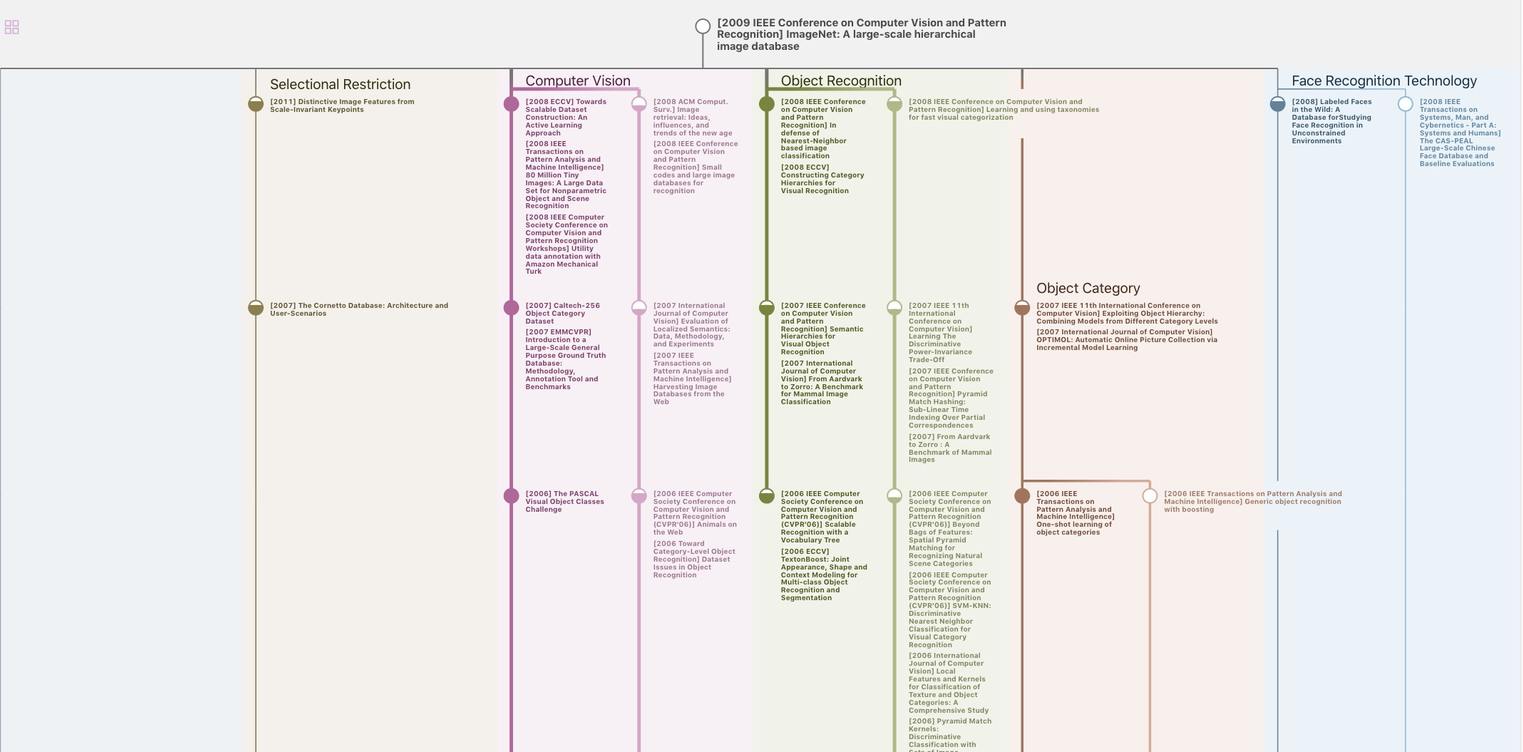
生成溯源树,研究论文发展脉络
Chat Paper
正在生成论文摘要