Intrinsic regularization effect in Bayesian nonlinear regression scaled by observed data
arxiv(2022)
摘要
Occam's razor is a guiding principle that models should be simple enough to describe observed data. While Bayesian model selection (BMS) embodies it by the intrinsic regularization effect (IRE), how observed data scale the IRE has not been fully understood. In the nonlinear regression with conditionally independent observations, we show that the IRE is scaled by observations' fineness, defined by the amount and quality of observed data. We introduce an observable that quantifies the IRE, referred to as the Bayes specific heat, inspired by the correspondence between statistical inference and statistical physics. We derive its scaling relation to observations' fineness. We demonstrate that the optimal model chosen by the BMS changes at critical values of observations' fineness, accompanying the IRE's variation. The changes are from choosing a coarse-grained model to a fine-grained one as observations' fineness increases. Our findings expand an understanding of BMS's typicality when observed data are insufficient.
更多查看译文
关键词
intrinsic regularization effect,nonlinear regression,bayesian,observed data
AI 理解论文
溯源树
样例
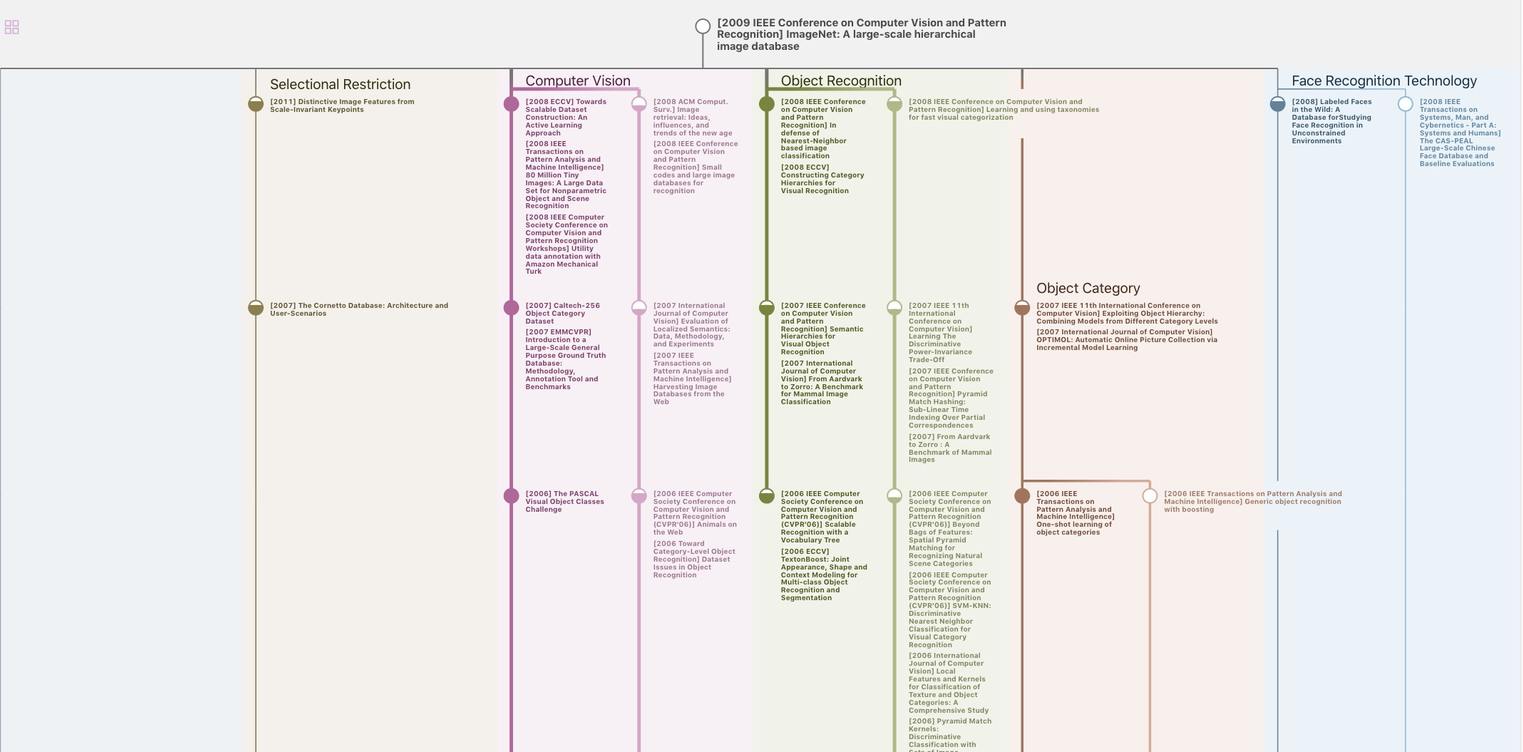
生成溯源树,研究论文发展脉络
Chat Paper
正在生成论文摘要